This App Could Help Detect COVID-19 By Analyzing A Person’s Speech
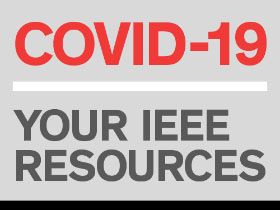
THE INSTITUTE A major challenge in containing the spread of COVID-19 is that its symptoms may not be conspicuous until a few days after an individual gets infected. During that time, a person could unwittingly be spreading the virus.
In the absence of a treatment vaccine or cure, early detection of symptoms is vital to cut down on the transmission. Based on existing literature and our ongoing research, there is strong evidence that COVID-19 symptoms could be detected from human speech, by applying signal processing techniques and artificial intelligence (AI) algorithms.
Speech contains inherent information about the physical, physiological, psychological, and emotional status of the speaker. Any variation in any of these is reflected in the person's speech. It generally isn't hard for a person to detect when someone is tired, happy, sad, angry, or sick just by listening to them speak.
Using signal processing and AI, finer variations in speech characteristics, which may not even be perceived by the listener or the speaker, can be detected and used for diagnostic purposes. For example, Mayo Clinic has been working on vocal biomarkers to diagnose health conditions such as heart disease. Dr. Charles Marmar, a psychiatrist at NYU Langone Health, has been investigating the possibility of diagnosing psychiatric disorders from speech samples. Sonde Health, a tech firm in Boston, is considering using voice tests to diagnose aging-related diseases such as dementia and Parkinson's.
We are developing an app that can analyze an individual's speech to detect COVID-19 symptoms so that the person can be quarantined, tested, and provided with medical support at a much earlier stage. The app will have the AI model incorporated so that people can regularly monitor themselves for COVID-19 symptoms. Our proposed diagnostic method using speech is only meant for initial screening and flagging of suspected COVID-19 positive individuals. [It is meant] to complement existing clinical diagnostic procedures, not replace them.
SPEECH VARIATIONSWhile COVID-19 symptoms may not be conspicuous to the affected individual or others, they will cause subtle variations to speech characteristics that can be detected by artificial intelligence (AI) algorithms. That's because infected individuals undergo changes to body parameters such as temperature, heart rate, blood pressure, and breathing rate. All of these affect the physiology of speech and are reflected in speech signals.
To train, validate, and optimize AI models, we will use a dataset comprised of speech recordings and body parameter measurements collected from a test population of hundreds of people. The dataset will include samples from healthy and asymptomatic individuals, as well as COVID-19 patients.

We are currently working with various hospitals and medical centers in India and Saudi Arabia to collect data, subject to approvals with regard to data-protection requirements. While we have already started collecting data for healthy individuals, we expect that by mid-July to have all formalities completed to collect data from symptomatic individuals as well as confirmed COVID-19 patients. Once the data-protection approvals and agreements are finalized, data collection would be an ongoing process.
DATA COLLECTIONTwo types of speech recordings will be collected: a complete sentence and a set of vowel sounds sustained for a few seconds, such as aaa or eee, to capture the finer details of the human voice box.
The recordings, along with body parameters measured at the same time using conventional biomedical devices, will be used to train the AI algorithms. Identity and personal details of the participating volunteers will be kept confidential. The only identifying information will be their age and gender. As variations are expected across age groups and genders, this information is necessary to understand those differences and incorporate them into the AI algorithms.
SIGNAL PROCESSING AND AIUsing signal processing techniques such as filtering and voice activity detection, the recorded speech signal, which is in a digital format, will be preprocessed to remove unwanted components and background noise. The preprocessed speech signal will be further refined using feature extraction algorithms to extract traits that characterize the speech signal. These features are applied as input to AI algorithms, which recognize a pattern or some intrinsic parameter associated with that pattern.
During the training phase of AI models, speech as well as the measured body parameter will be used as input to the AI algorithms. Each body parameter is associated with a different characteristic or pattern within signal-processed speech. Heart rate, for example, is reflected in certain frequency features (the Mel Frequency Cepstral Coefficients). As a result, a separate model for each measured body parameter needs to be developed. We plan to use 80 percent of the samples in the dataset for training purposes and the remaining 20 percent for testing, validating, and optimizing the AI algorithms. Body temperature, blood pressure, and other parameters (not to mention speech characteristics) vary between healthy people.
This is precisely the reason why we need a lot of samples from individuals in each category-healthy, symptomatic, and COVID-19 patients. With a large number of samples, individual variations within each category can, in a sense, be averaged out to get a more accurate AI model. The validation will be done by comparing the diagnosis of the AI model with the clinical diagnosis corresponding to the collected data.
The validated and tested AI models will then be deployed for field testing in collaboration with healthcare agencies. A large-scale deployment is possible by incorporating the AI models into an app.
CHALLENGESOne of the main challenges we face in this project is data collection. Getting access to COVID-19 patients or even symptomatic individuals to collect their data is difficult as it involves several procedures related to approvals, agreements, and precautions.
Secondly, not all patients might be willing to share their samples.
Another is ensuring that false positives are minimized. Too many false positives (or false alarms) can cause unnecessary panic and distress in people and can also lead to chaotic scenarios, such as people rushing to their nearest healthcare centers and overwhelming them.
Of course, false negatives also have to be minimized. Too many false negatives simply mean the app doesn't detect COVID-19 symptoms. The objective is to have an app that detects COVID-19 symptoms with high accuracy, while minimizing false positives.
AI algorithms can be trained and tuned to deliver high accuracy but they are not 100 percent accurate. Therefore, it important to convey the error-performance metrics of the algorithms to users.
OTHER USESIf successful, we think our proposed method could have broad applications in areas such as medical diagnosis and patient monitoring and care. Speech can be used to monitor the psychological and emotional effects the pandemic is having on individuals resulting from lockdowns, the death of loved ones, and the loss of jobs and income.
Mohammed Usman, Mohd Wajid and Mohammed Zubair are all IEEE senior members. Usman and Zubair are assistant and associate professors, respectively, of electrical engineering at King Khalid University in Abha, Saudi Arabia. Wajid is an assistant professor of electronics engineering at Aligarh Muslim University in India. Ahmed is a physician and internist with Blackpool Teaching Hospitals NHS Trust, in Blackpool, Lancashire, U.K.
Attention IEEE members: are you part of a team responding to the COVID-19 crisis? We want to hear from you! Wherever you are and whatever you are doing, if you are helping deal with the outbreak in some way, let us know. Send us accounts of anywhere from 200 to 800 words, or simply give us a rough idea of what you are doing and your contact information. Write to: k.pretz@ieee.org
< Back to IEEE COVID-19 Resources