Beyond the AI hype cycle: Trust and the future of AI
There's no shortage of promises when it comes to AI. Some say it will solve all problems while others warn it will bring about the end of the world as we know it. Both positions regularly play out in Hollywood plotlines like Westworld, Carbon Black, Minority Report, Her, and Ex Machina. Those stories are compelling because they require us as creators and consumers of AI technology to decide whether we trust an AI system or, more precisely, trust what the system is doing with the information it has been given.
This content was produced by Nuance. It was not written by MIT Technology Review's editorial staff.
Joe Petro is CTO at Nuance.
Those stories also provide an important lesson for those of us who spend our days designing and building AI applications: trust is a critical factor for determining the success of an AI application. Who wants to interact with a system they don't trust?
The black box and understanding the unknownsEven as a nascent technology AI is incredibly complex and powerful, delivering benefits by performing computations and detecting patterns in huge data sets with speed and efficiency. But that power, combined with black box" perceptions of AI and its appetite for user data, introduces a lot of variables, unknowns, and possible unintended consequences. Hidden within practical applications of AI is the fact that trust can have a profound effect on the user's perception of the system, as well as the associated companies, vendors, and brands that bring these applications to market.
Advancements such as ubiquitous cloud and edge computational power make AI more capable and effective while making it easier and faster to build and deploy applications. Historically, the focus has been on software development and user-experience design. But it's no longer a case of simply designing a system that solves for x. It is our responsibility to create an engaging, personalized, frictionless, and trustworthy experience for each user.
AI is data hungry: Know what you're feeding itThe ability to do this successfully is largely dependent on user data. System performance, reliability, and user confidence in AI model output is affected as much by the quality of the model design as the data going into it. Data is the fuel that powers the AI engine that virtually converts the potential energy of user data into kinetic energy in the form of actionable insights and intelligent output. Just as filling a Formula 1 race car with poor or tainted fuel would diminish performance, and the driver's ability to compete, an AI system trained with incorrect or inadequate data can produce inaccurate or unpredictable results that break user trust. Once broken, trust is hard to regain. That is why rigorous data stewardship practices by AI developers and vendors are critical for building effective AI models as well as creating customer acceptance, satisfaction, and retention.
Responsible data stewardship establishes a chain of trust that extends from consumers to the companies collecting user data and those of us building AI-powered systems. It's our responsibility to know and understand privacy laws and policies and consider security and compliance during the primary design phase. We must have a deep understanding of how the data is used and who has access to it. We also need to detect and eliminate hidden biases in the data through comprehensive testing.
Treating user data as proprietary source code'Treat user data as sensitive intellectual property (IP). It is the proprietary source code used to build AI models that solve specific problems, create bespoke experiences, and achieve targeted desired outcomes. This data is derived from personal user interactions, such as conversations between consumers and call agents, doctors and patients, and banks and customers. It is sensitive because it creates intimate, highly detailed digital user profiles based on private financial, health, biometric, and other information.
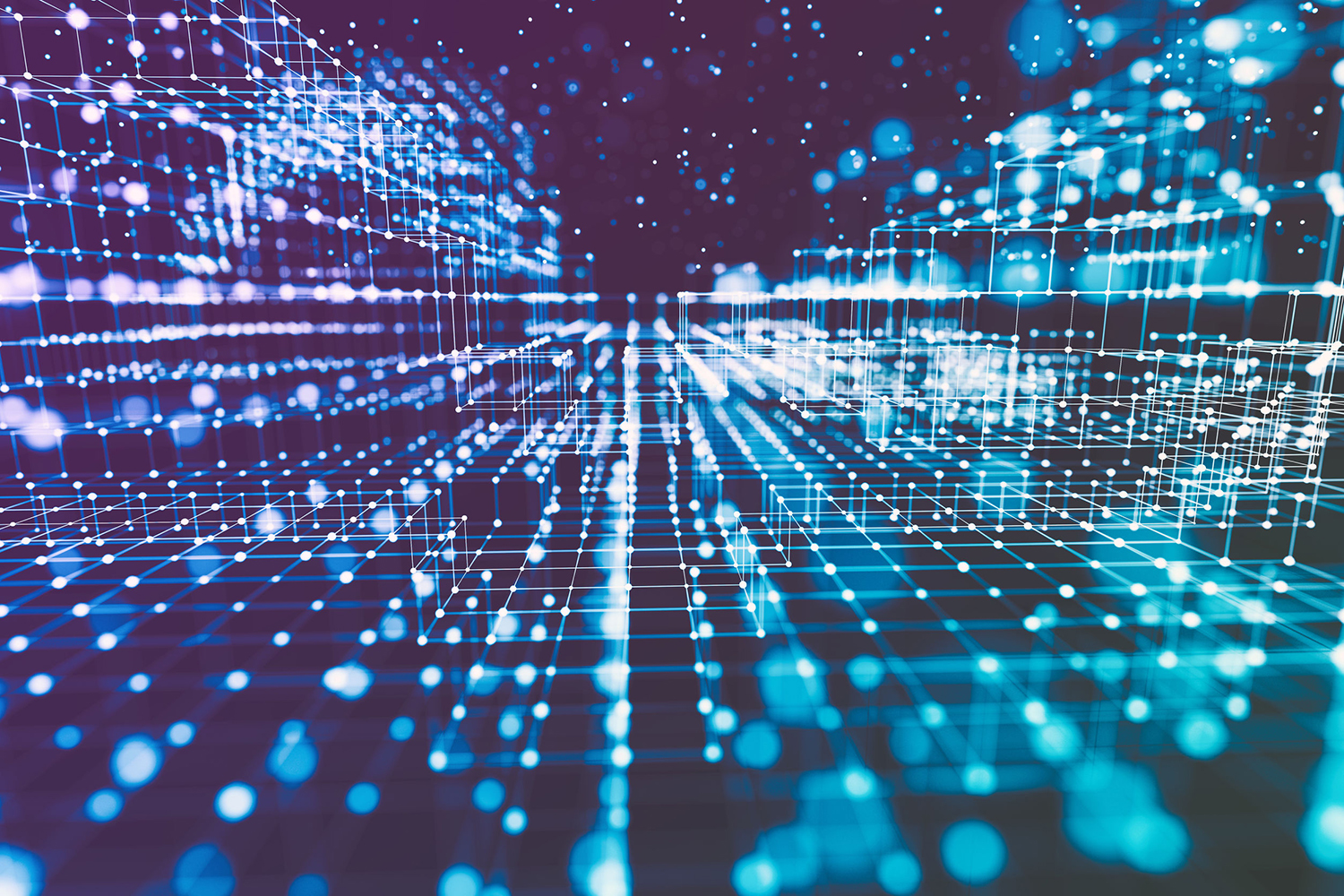
User data needs to be protected and used as carefully as any other IP, especially for AI systems in highly regulated industries such as health care and financial services. Doctors use AI speech, natural-language understanding, and conversational virtual agents created with patient health data to document care and access diagnostic guidance in real time. In banking and financial services, AI systems process millions of customer transactions and use biometric voiceprint, eye movement, and behavioral data (for example, how fast you type, the words you use, which hand you swipe with) to detect possible fraud or authenticate user identities.
Health-care providers and businesses alike are creating their own branded digital front door" that provides efficient, personalized user experiences through SMS, web, phone, video, apps, and other channels. Consumers also are opting for time-saving real-time digital interactions. Health-care and commercial organizations rightfully want to control and safeguard their patient and customer relationships and data in each method of digital engagement to build brand awareness, personalized interactions, and loyalty.
Every AI vendor and developer not only needs to be aware of the inherently sensitive nature of user data but also of the need to operate with high ethical standards to build and maintain the required chain of trust.
Here are key questions to consider:
Who has access to the data? Have a clear and transparent policy that includes strict protections such as limiting access to certain types of data, and prohibiting resale or third-party sharing. The same policies should apply to cloud providers or other development partners.
Where is the data stored, and for how long? Ask where the data lives (cloud, edge, device) and how long it will be kept. The implementation of the European Union's General Data Protection Regulation, the California Consumer Privacy Act, and the prospect of additional state and federal privacy protections should make data storage and retention practices top of mind during AI development.
How are benefits defined and shared? AI applications must also be tested with diverse data sets to reflect the intended real-world applications, eliminate unintentional bias, and ensure reliable results.
How does the data manifest within the system? Understand how data will flow through the system. Is sensitive data accessed and essentially processed by a neural net as a series of 0's and 1's, or is it stored in its original form with medical or personally identifying information? Establish and follow appropriate data retention and deletion policies for each type of sensitive data.
Who can realize commercial value from user data? Consider the potential consequences of data-sharing for purposes outside the original scope or source of the data. Account for possible mergers and acquisitions, possible follow-on products, and other factors.
Is the system secure and compliant? Design and build for privacy and security first. Consider how transparency, user consent, and system performance could be affected throughout the product or service lifecycle.
The reality of AI in actionBiometric applications help prevent fraud and simplify authentication. HSBC's VoiceID voice biometrics system has successfully prevented the theft of nearly 400 million (about $493 million) by phone scammers in the UK. It compares a person's voiceprint with thousands of individual speech characteristics in an established voice record to confirm a user's identity. Other companies use voice biometrics to validate the identities of remote call center employees before they can access proprietary systems and data. The need for such measures is growing as consumers conduct more digital and phone-based interactions.
Intelligent applications deliver secure, personalized, digital-first customer service. A global telecommunications company is using conversational AI to create consistent, secure, and personalized customer experiences across its large and diverse brand portfolio. With customers increasingly engaging across digital channels, the company looked to technology partners to expand its own in-house expertise while ensuring it would retain control of its data in deploying a virtual assistant for customer service.
A top-three retailer uses voice-powered virtual assistant technology to let shoppers upload photos of items they've seen offline, then presents items for them to consider buying based on those images.
Ambient AI-powered clinical applications improve health-care experiences while alleviating physician burnout. EmergeOrtho in North Carolina is using the Nuance Dragon Ambient eXperience (DAX) application to transform how its orthopedic practices across the state can engage with patients and document care. The ambient clinical intelligence telehealth application accurately captures each doctor-patient interaction in the exam room or on a telehealth call, then automatically updates the patient's health record. Patients have the doctor's full attention while streamlining the burnout-causing electronic paperwork physicians need to complete to get paid for delivering care.
AI-driven diagnostic imaging systems ensure that patients receive necessary follow-up care. Radiologists at multiple hospitals use AI and natural language processing to automatically identify and extract recommendations for follow-up exams for suspected cancers and other diseases seen in X-rays and other images. The same technology can help manage a surge of backlogged and follow-up imaging as covid-19 restrictions ease, allowing providers to schedule procedures, begin revenue recovery, and maintain patient care.
As digital transformation accelerates, we must solve the challenges we face today while preparing for an abundance of future opportunities. At the heart of that effort is the commitment to building trust and data stewardship into our AI development projects and organizations.