Blind test shows AI-enhanced MRI scans are just as good but 4 times faster
An AI-based technique to accelerate MRI scans has been shown to produce as good or better results than traditional methods in a blind comparison by expert radiologists. The system halves or even quarters the time necessary to get a good scan without sacrificing quality, which could reduce wait times and costs correspondingly.
FastMRI is a project that began in 2015 at NYU and became a team effort with Facebook AI Research (FAIR) almost exactly two years ago. The medical and imaging expertise on NYU's side was a good match the AI expertise on FAIR's, and the collaboration has been fruitful.
The idea behind it is simple enough to grasp. MRIs can take anywhere from 15 minutes to an hour depending on how much data must be collected, from what angles, and so on. This can be a very difficult process for the patient, since they have to remain immobile inside a large, loud machine, and of course that means a machine can only scan so many people per day, driving costs and wait times up.
It was theorized by the FastMRI team that some of the data collected is essentially redundant and a well-trained machine learning system could fill in the gaps if less information was collected in the first place. It would only work because MRIs (of knees in this case) are very orderly and predictable in many ways, and there's a large body of imagery for an AI to learn from.
Over the last two years the team put together a study whereby clinical radiologists examined both traditionally generated MRIs and the ones created by an AI using substantially (about 75 percent) less source data - never aware of which was which, naturally, and at least a month apart from one another.
The results were quite promising:
The study found there were no significant differences in the radiologists' evaluations. They found the same abnormalities and pathology regardless of whether they were examining the standard or AI-generated MRIs. All the examiners judged the AI-accelerated images to be of better overall quality than the traditional ones. Five of the six radiologists were not able to correctly discern which images were generated using AI.
It's interesting that the AI group of images was universally rated better than the ground truth group, but only one person was able to say which was which at a better-than-chance rate.
There are slight differences in the amount and type of noise' in the AI-generated and traditional MRIs that the one radiologist may have potentially noticed," said FAIR's Larry Zitnick, one of the authors of the paper. I was curious about how the AI might produce better" images, and he obliged me with some details:
Since the AI learns on prior MRI knees, i.e., what typical knees look like, it can improve the appearance of the MRI by removing noise or other artifacts," Zitnick said. So, for example, white noise looks like a random pattern to the AI, so it's difficult to learn how to reproduce it. However, the structure of human knees is similar across patients making it easier to learn and reconstruct. As a result, when the AI reconstructs the knee, it tends to reduce the noise (what it has trouble learning) and increase the structures (what it learns)."
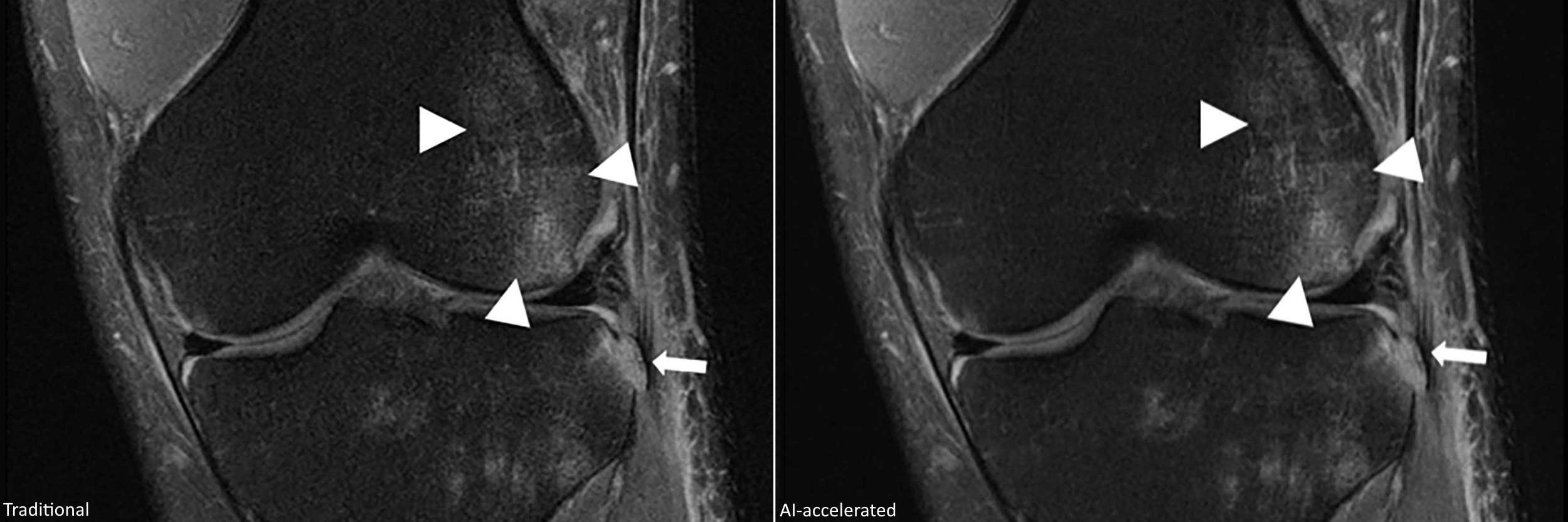
Traditional MRI, left, and AI-accelerated MRI, right, using a quarter of the data.
The research comes with a couple small caveats. For one, it's only shown to work for knees at present, though brain scans are the next stop and there's no reason to think it won't work for those and other types.
More importantly, the study is based on partly synthetic data, in that the undersampled scans were traditional scans with data removed (so the AI version can easily be compared to the original) rather than scans that never collected the extra" info in the first place. Due to the nature of MRIs, however, removing the information and never collecting it are pretty much the same thing, so this may not actually matter at all. But there's a certain amount of confidence necessary for a hospital to not do a full scan, since it may be the only chance to get that scan.
The study is an important step towards making this practice actually usable in a medical facility with real patients in a hurry. If it's accepted in this way, it could reduce not only the time someone might have to spend in the machine considerably, but aid radiologists in turning diagnoses around faster and helping more patients.