The POWER Interview: AI, Big Data, and Efficiency
The post The POWER Interview: AI, Big Data, and Efficiency appeared first on POWER Magazine.
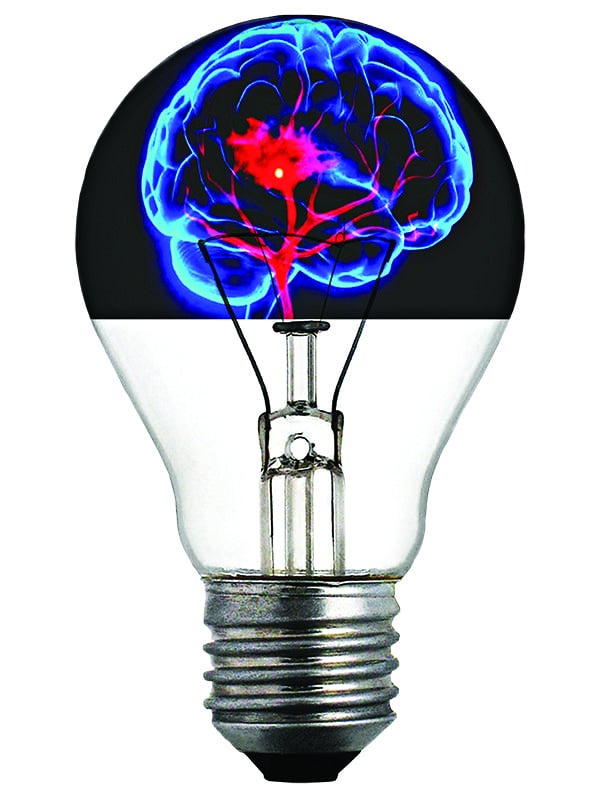
The increased use of artificial intelligence (AI) and machine learning (ML) in the power generation sector has a goal of making electricity production both more efficient, and secure. Developing ways to more quickly analyze ever-larger amounts of data is driving innovation among those people responsible for the operation of power plants and generation equipment.
Many companies are involved in the research and development of technologies to support AI and ML. Beyond Limits, a California company launched in 2014, works in several markets, from energy-including the power generation and oil and gas exploration sectors-to manufacturing and industrial, as well as healthcare. The company says, Our mission is to create automated solutions with human-like powers of reasoning that amplify the talents and capabilities of people. We specialize in complex challenges in extreme environments."
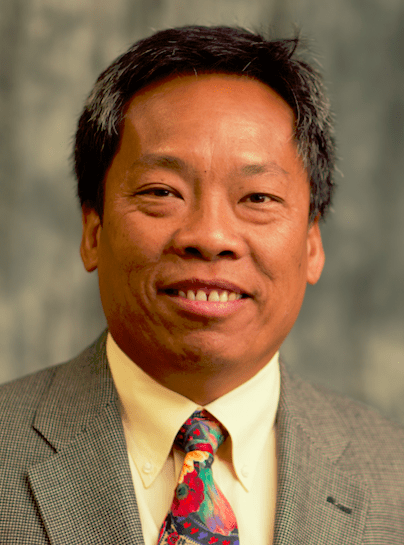
Stephen Kwan, the company's director of product management for Power Generation/Grid Management, provided POWER with his insight into AI and ML as it applies to the electricity sector, with a look at what the future holds as the power generation landscape continues to change.
POWER: How can the power generation industry benefit from AI and machine learning?
Kwan: Machine learning (ML) and advanced artificial intelligence (AI) solutions are valuable sources of technology currently powering the development of smart applications that have the ability to make very accurate decisions, autonomously, based on learned historical data. Such technologies are being used extensively by the power generation industry to develop customer-centric solutions that understand the evolution of their needs, leveraging essential data such as historical knowledge, expertise, and best practices to make automatic recommendations when decisions to challenging scenarios have to be made in real-time.
Globally, experienced power generation operators represent an ever-shrinking workforce whose deep domain know-how and expertise are critical to efficient, safe, and reliable operations. This subject matter expertise needs to be captured, digitized, and made accessible across the workforce in order to ensure the long-term continuity, efficiency, and reliability of operations across the power generation domain. Novel hybrid AI/ML approaches represent a necessary avenue for combining the value of data and domain knowledge to tackle global challenges facing the power generation industry.
POWER: What about the impact of AI and ML on distributed power generation?
Kwan: AI/ML approaches will add significant value to the ever-growing deployment of large-scale, geographically distributed energy resources. The optimal management of trade-offs between meeting demand, ensuring low risk and reliable operations, and maintaining the integrity of key assets will require AI/ML systems capable of building and supporting holistic models of specific power planning and scheduling, generation, and distribution processes.
Advanced AI/ML solutions can significantly improve the management of the integrity and health of key large-scale assets spanning the generation (e.g. turbines) and distribution (e.g. grids/smart meters, etc.) domains. This can be achieved by combining predictive models (both supervised-learning to recognize and predict specific events and patterns- and unsupervised-learning to detect anomalies and potential events of interest) that learn from a wealth of historical data on critical assets and their operations. Such systems are educated and informed by relevant domain expertise captured and digitized from skilled operators, engineers, and decision-makers.
Asset health and performance are constantly changing as time progresses. In order to maximize profits, it's important to continually learn from, and adapt to, these changes. AI solutions can provide a dynamic assessment of key parameters, in combination with leveraged domain expertise, leading to more optimal operations of valuable power generation assets. Power generation facilities are often optimized to run within a specific operating envelope and with most operators being trained to support these scenarios. With the proliferation of various energy resources, such as solar and wind, operators must adapt to running such equipment independent of traditional operating ranges. AI can take into consideration the interactions of these unconventional operating set points-in combination with subject-matter expert expertise-to more effectively operate the assets optimally, with lower risk.
POWER: Can machines used in power generation learn from their experiences; would an example be that a machine could perform more efficiently over time based on past experience?
Kwan: Advanced artificial intelligence solutions have the ability to empower software applications to help the power generation industry better analyze large data sets and ID patterns. Such AI systems continually monitor entire processes and learn over time based on historical experiences and data, helping power generation facilities better detect anomalies and make more precise predictions, thus improving overall operational efficiency.
Advanced AI approaches have the potential to revolutionize the management of critical assets across the power generation domain, combining historical and real-time operational datasets with deep subject matter expertise to provide recommendations on asset health management to maximize the efficiency, reliability, and safety of operations while meeting power generation demand and financial objectives.
An example would be leveraging AI solutions to help minimize unexpected system trips and other risks to asset health, integrity, and longevity. Many variables and factors, both historical and real-time operations, such as planning and scheduling targets, equipment constraints, maintenance and more must be monitored holistically in order to remediate and mitigate potential issues in a timely manner, reducing risks to generated power output targets, personnel, assets, and reliability of operations.
AI/ML solutions, trained with and exposed to data from both normal operations, and-when applicable-circumstances representing anomalies or abnormal operations, can extract relevant patterns across historical and current data, predicting and detecting potential system trips and quantifying the risk of unexpected downtime. By means of digitizing relevant domain knowledge, such advanced solutions can then generate explainable and actionable recommendations to help mitigate and remediate potential risks identified by data-driven models targeting critical equipment and assets.
POWER: Are there specific challenges for power plant operators that AI and ML can help solve?
Kwan: A challenging scenario could be that a particular power generation facility may need to take drastic actions if another facility in the grid goes down. In such an instance, machine learning and other AI approaches can be used to predict a facility's limit to ramp up in support of the generation facility experiencing loss. For example, if a power plant goes down due to a trip, the remaining generation facilities participating in the grid would need to make up the loss of many megawatt hours. In that instance, it's critical to know the maximum each facility can produce to make up for the loss.
Machine learning and other AI approaches continually learn from historical operating parameters and conditions, decreasing the odds of faltering in the execution necessary to achieve specific targets and goals. This fact remains true under all operating conditions regardless of the skillset, experience, or knowledge base of any one operator. In addition, such solutions digitalize and streamline how essential knowledge is passed between operators thereby decreasing misinterpretation or miscommunication.
POWER: There are certainly many uses for AI and ML in the power industry; what are some of the major ways the technology can support operations?
Kwan: Artificial intelligence can advance entire operations in the power industry by improving infrastructure and asset monitoring, power trading, and outage supervision, prediction, and planning. AI systems can also help better forecast scenarios of various natures including load and power generation. Such unparalleled insights could yield greater potential for power trading and increased risk mitigation.
Outside of uptime optimization for power generation equipment and facilities (e.g. turbines, distributed energy resources, etc.), advanced AI solutions also have the capacity to digitalize and democratize domain knowledge and operational know-how captured from skilled workforces to help solve the challenge of a shrinking global workforce. Improved asset integrity management, predictive maintenance, and anomaly detection with recommendations for remediation/mitigation lead to maximization of operational efficiency against risk, reliability, demand planning, and other similar constraints. This is accomplished via optimized placement and selection of sensors distributed across critical assets to maximize the accuracy of forecasting and detecting operational anomalies.
The issue of distributed energy resources can also be impacted by artificial intelligence. Optimizing operations in a safe and reliable manner without negatively affecting asset health and longevity (e.g. faster start-up and shutdown of gas turbines) can be the basis for minimizing cost, maximizing profit, and ensuring stability for grid equipment health/lifespan. Advanced AI software can act as virtual sensors to infer what a measurement would be in the absence of a real sensor or unmeasurable property, thus providing support for better assessment and prediction of electric grid stability and potential brownouts or blackouts during urban planning processes.
POWER: How can the trend of decentralized power generation benefit from AI?
Kwan: The global energy market is transforming from highly centralized power distribution systems to more complex networks of decentralized systems and the industry is having trouble integrating distributed energy resources and renewables during this transitional period. Intelligent asset management strategies and notifications systems provided by AI solutions can increase grid reliability and provide better maintenance for an aging infrastructure (transmission and distribution systems).
The variability and uncertainty associated with decentralized systems further complicate the management of critical assets as opposed to smaller-scale, localized power generation facilities. AI/ML approaches will play a significant role in augmenting, monitoring, and managing capabilities across decentralized resources, leveraging a wealth of current and historical data streams while incorporating the deep industry know-how required to ensure the adoption and deployment of such AI-based systems.
Decentralized systems often operate in silos, affecting their ability to detect and account for perturbations that can be introduced by others. AI provides the basis to understand the effects of individual actions on the stability and reliability of the grid and overall generation capabilities.
POWER: How important is AI to smart grids? How important is AI to the integration of e-mobility (electric vehicles, etc.) to the grid?
Kwan: Artificial intelligence is very important to smart grids as they consist of smart meters/appliances, renewable and other energy-efficient resources, and a large number of other devices. These devices provide a gold mine of data that only intelligent systems can decipher and convert into valuable insights and forecasts. It is also extremely difficult to model those devices because it's challenging to understand the effects of changes on the overall availability and reliability of the grid. Advanced AI solutions can provide the ability to model and understand changes that can affect the operations of the electric grid-such as the effect of increasing EV usage, for example.
POWER: How can AI be used in power trading, with regard to forecasts, etc.?
Kwan: Artificial intelligence solutions such as supervised learning models can predict the behavior of creditors or consumers with a high degree of accuracy. Algorithmic trading leverages reinforcement learning to reward and/or rebuke trading bots in response to how much money is made. Rules must be put in place such as stop-loss prevention, of course. This is comparable to a self-driving car security mechanism that would prevent pedestrian/car collisions. Advanced AI can reduce risks from current events and their inherent impacts on the market as models can be retrained with new market conditions front-of-mind. While human intervention and careful monitoring of deployed algorithms will still be required for the foreseeable future, the support of AI has the potential to help the industry ramp up technological advancements at a more accelerated pace.
The accuracy of electricity demands has a huge impact on power trading. Inaccurate forecasts can lead to losses for the traders and (most importantly) affect power availability. For example, if forecasts are wrong and power generation units are sitting idle when power shortfalls exist in the grid, this can lead to very costly and disruptive mitigations. AI solutions can support complex forecasting models with a large number of inputs and simple updating capabilities to reflect dynamic changes that provide more accurate near real-time forecasts.
POWER: How important is AI to the design and construction" of virtual power plants?
Kwan: A virtual power plant is a clustering of distributed heterogeneous generation units and an integral part of the Internet of Energy" (improved monitoring and control of smart grid). Intelligent technological solutions are necessary to utilize before the plant construction phase to ensure the design meets the intended supply generation needs and for identification of potential problems, the projected location and cause of those problems, and probable actions for minimization of adverse impacts. Intelligent electronic devices are used to control the flow of power and operate equipment while making local decisions. AI solutions can enable the analysis and interpretation of what-if" scenarios enabled by the design and construction of virtual power plants, enhancing learning capabilities that can ultimately be transferred to real-world operations in the form of predictive models and decision-making advisors. AI models can supplement first-principle based models when developing a virtual power plant - especially in cases where first-principle methods are too slow or too complex.
POWER: Machine learning and AI in power generation rely on digitalization. As the use of data becomes more important, what steps need to be taken to support AI and machine learning while still accounting for cybersecurity?
Kwan: Given AI requires a lot of data, it's vital to incorporate subject matter experts when creating the machine learning/AI models so as to understand the minimum required data set. It's also important to follow IT best practices to ensure data is isolated from critical infrastructure like the control network, confirm only the least-required privilege is given to the users or applications, and implement logging and audits per industry security measures.
In a typical power generation facility, the control system resides on an isolated and dedicated network. Meanwhile, business users are typically on separate networks that are more open" for cybersecurity threats. All AI/ML applications require good/clean data for optimal functionality. As such, any data necessary for AI/ML solutions-that reside on the control network- need to be pushed to business networks in a safe and reliable manner. AI/ML applications should avoid reaching across isolated networks to access necessary data. This requires good IT practices, employing a solution to take advantage of products that permit only one-way data flow (e.g. data diodes).
By preventing network traffic into the control network and only allowing data to be pushed from the control network, it decreases the chance of cybersecurity incidents on the control network. Industry best practices for ensuring data integrity should be deployed to ensure data is not changed. If changes are necessary, they must be auditable. Lastly, any recommendations for the AI/ML application should be explainable so that subject matter experts can verify the results if/when appropriate.
POWER: What do you see as the future of AI and machine learning for power generation / utilities?
Kwan: Integration and adoption of machine learning and other artificial intelligence solutions are already increasing exponentially. At this pace, the outlook for advanced technologies points toward AI approaches becoming the gold standard for the future growth of the power generation/utilities industry.
-Darrell Proctor is associate editor for POWER (@POWERmagazine).
The post The POWER Interview: AI, Big Data, and Efficiency appeared first on POWER Magazine.