Intelligent models for smarter decision-making
The popularity of the design, build, and test approach to engineering is fast-waning as today's engineers face unprecedented pressure to innovate, keep pace with the latest technologies, and design creative solutions to urgent problems.

Consider, for example, automated driving systems. Although autonomous vehicles promise to significantly improve mobility, engineers must test these frameworks for critical factors such as safety and potential system failures. Toyota is one of the automakers working to make driverless systems safe. In 2016, Toyota president and CEO Akio Toyoda said more testing would be needed to complete its mission-some 8.8 billion miles of it.
Fortunately, says Stefan Jockusch, vice president of strategy at Siemens Digital Industries Software, simulation can help. By virtually testing millions of real-world scenarios, from snowy road conditions to careless pedestrians, simulation technology can analyze autonomous vehicles' performance while accelerating development and reducing costs.
But while simulation is critical to the digital development and manufacturing of today's and tomorrow's products, challenges such as increased complexity and a lack of domain knowledge are prompting organizations to bolster their simulation processes with artificial intelligence (AI) capabilities.
AI as intelligent augmenterAlthough challenges can vary, Don Tolle, a director at consulting and research company CIMdata, says, one of the key barriers to simulation is the fair amount of time it takes to turn around a complex simulation and share the results with others, including design engineers and simulation analysts." In fact, Tolle says it can take weeks" to design, collect information, build, execute, and analyze simulation models to support decision-making.
Complexity is another obstacle engineers must tackle. Simulation models can provide deeper and more accurate insights into the behavior of manufacturing systems-but these additional details can come at the cost of greater computation. Building simulation models also demands talent with deep domain and mathematical knowledge. Many organizations are focused on democratizing access to simulation tools by making them a standard part of design and manufacturing processes. But the challenge, warns Tolle, is making these tools consumable by the average engineer who may not have deep domain knowledge in the specifics of a simulation and simulation technology." After all, developing AI algorithms is only part of the simulation process; engineers need domain knowledge to understand the broader context of how the models are being built and the purpose they serve.
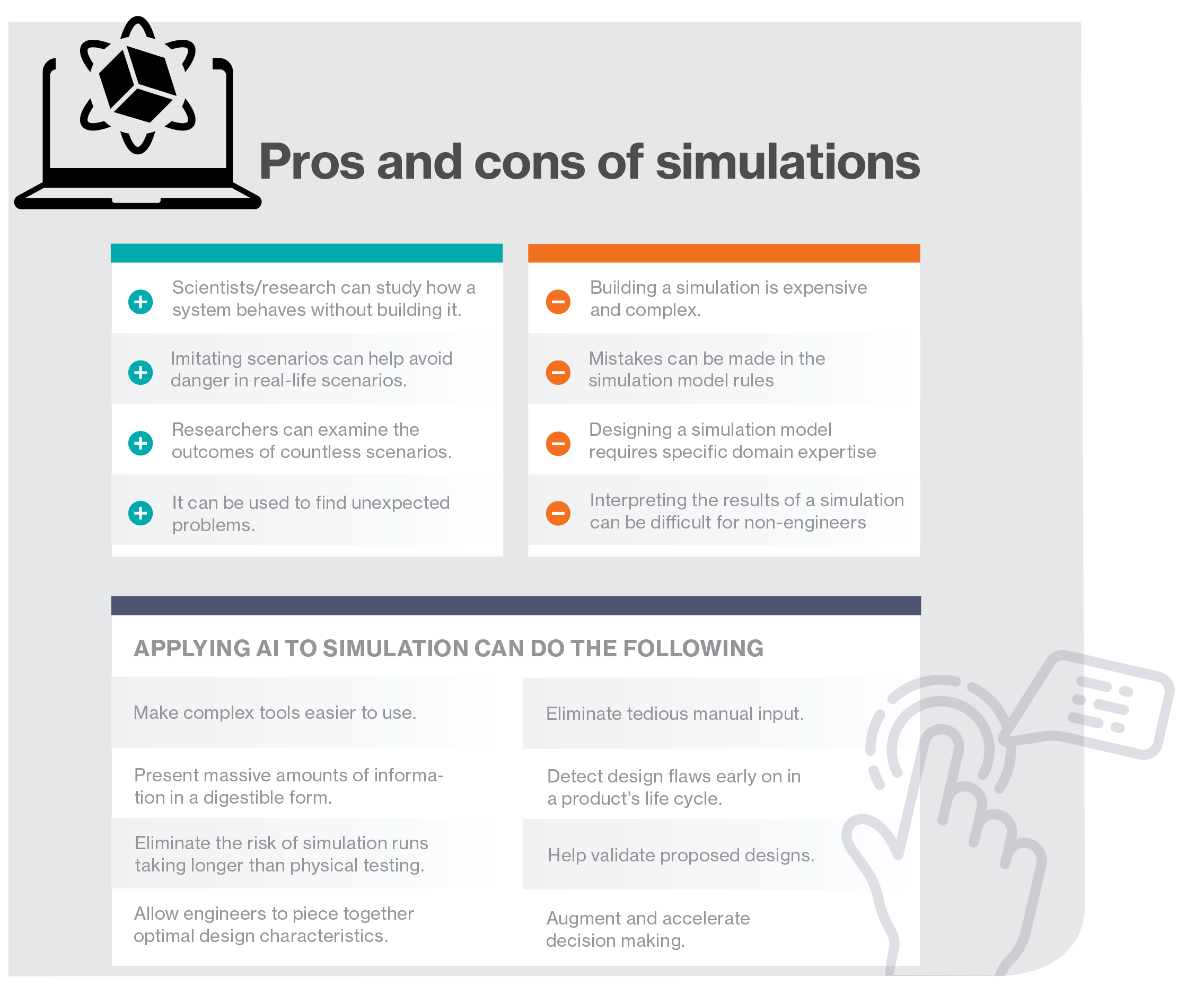
In response to the hurdles, many organizations are turning to AI to accelerate and simplify simulation-and for good reason. AI can distill information into a form that's easier for engineers to understand and more transparent, eliminating the need to interact with every detail of a model. The ability to create these incredibly complex models is one of the areas where artificial intelligence and machine learning will have the biggest impact," says Tolle.
That's because AI can learn" expertise from the vast volume of simulation datasets created by thousands of simulation runs in similar applications. As a result, AI can propose model parameters that allow for an optimal set of design characteristics for the system while eliminating the risk of simulation runs taking longer than physical testing. Following this, engineers can begin piecing together optimal design characteristics for more detailed designs, such as 3D computer-aided designs, software development, and electronics. Simulation augments the intelligence of the engineer by using AI and [machine learning] to improve how we conduct analytics and use data," says Tolle.
No shortage of use casesAI can help make simulation practical in cases where it otherwise wouldn't be-for example, when a designer quickly wants to test and validate many design configurations.
Simulations can be computationally expensive-for example, the charging behavior of a hybrid electric vehicle for thousands of types of drive cycles," Jockusch says. AI helps develop so-called surrogate models, using thousands of existing simulations to derive highly simplified, computationally much less expensive models that are accurate enough to guide designers through a complex decision space."
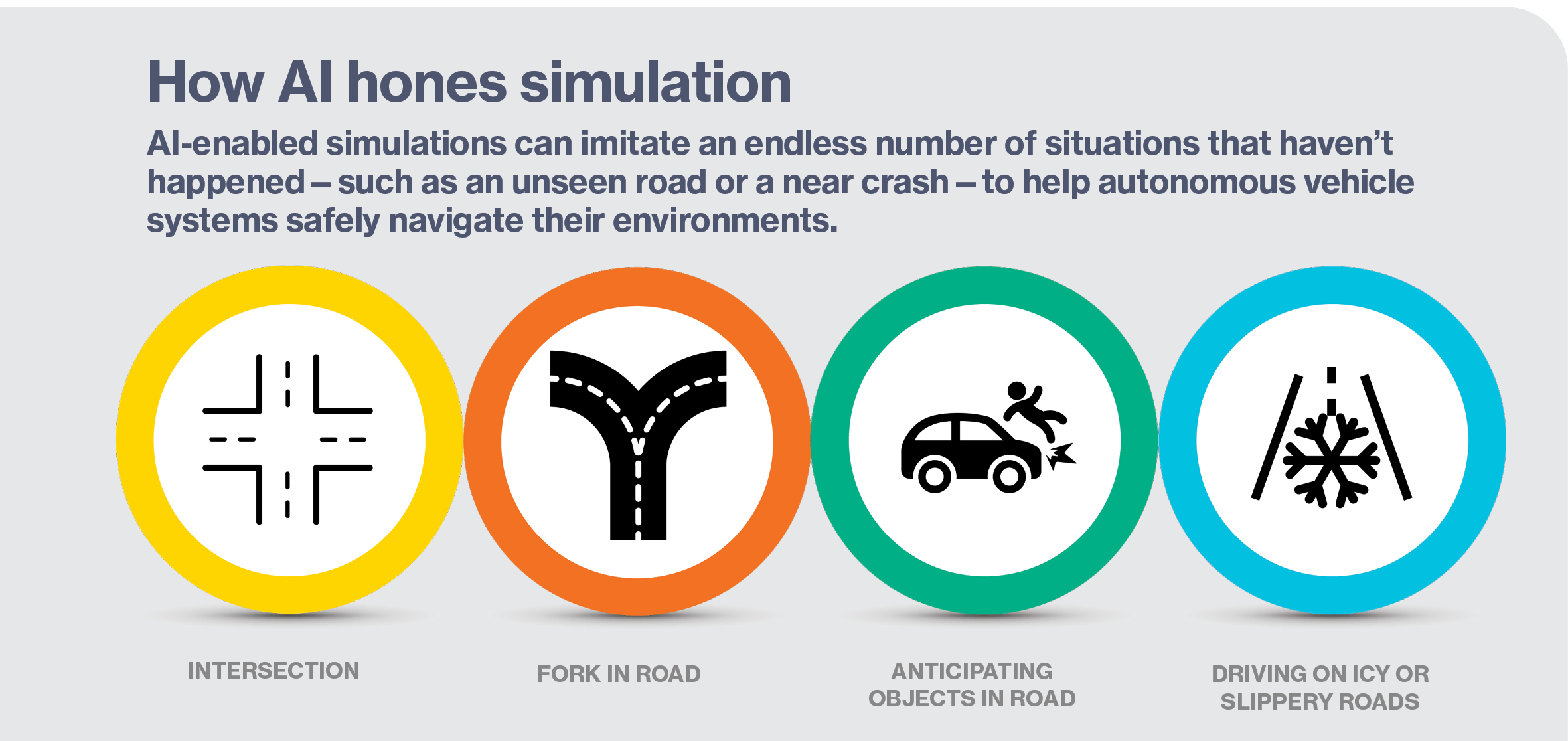
Another advantage of AI is its ability to detect design flaws early on in a product's life cycle. There have been some notable examples of system failures or system oversights in the last four or five years in both the aerospace and the automotive industries with major recalls and major problems," says Tolle. The cost of making decisions late in the life cycle is huge."
The good news, he says, is AI can minimize the risk of introducing flaws into product design by enabling engineers to validate systems all throughout their development. This allows for smarter and faster design decisions and trade-offs early in the design life cycle rather than having to change the design later on, which can be costly in complex systems."
Download the full report.
This content was produced by Insights, the custom content arm of MIT Technology Review. It was not written by MIT Technology Review's editorial staff.