Using Asset Data to Create Effective Maintenance Strategies
The post Using Asset Data to Create Effective Maintenance Strategies appeared first on POWER Magazine.
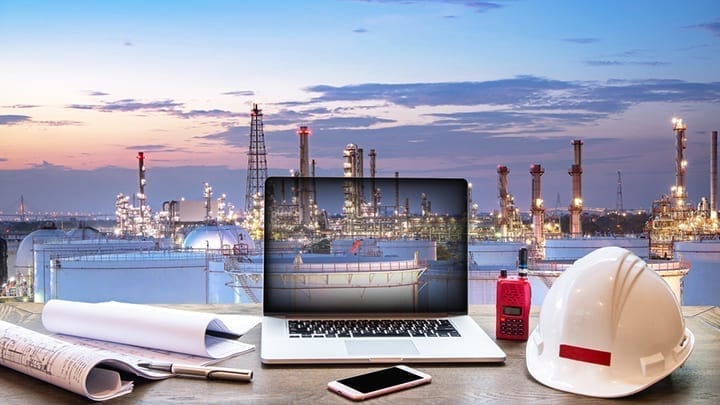
Relationships between asset data utilization and maintenance strategies often get complicated, especially in cases where data collection practices are designed to merely follow and not build strategies. In the maintenance, repair, and operations (MRO) sector, the best approach is to optimize the strategy from time to time, which requires comprehensive analysis of asset data collection practices, integrations, and implementation procedures.
Preventive and Predictive Maintenance StrategiesNo matter what maintenance strategy you follow, insight generated out of data can only help you when it is accurate and detailed. In the case of preventive maintenance, you are less dependent on using data for decision-making. Data collection for this type of maintenance strategy is focused on recordkeeping in a time-bound manner. In this type of maintenance strategy, you trigger actions based on existing information; whereas, in predictive maintenance, machines help you trigger required action.
What the action should be depends on the parameters and criteria you have set for specific patterns and anomalies. The role of your data collection framework is crucial here, which supports the analysis (mainly of condition), inventory, and performance of assets.
A major problem occurs when the framework is not designed to link to multiple aspects of data-asset type, people, and processes that impact the service delivery. A solid framework must link these aspects to help decision-makers correlate data and generate more useful insight.
Data LinkageData linkage is usually done on a need-to-know basis to achieve an objective. In maintenance operations, processes are intertwined. For example, when a service request is raised against a maintenance requirement, the service manager creates a job out of it, and then schedules and dispatches service technicians to fix the issue. That means teams across the department have to collaborate and share information for a successful resolution.
If the objective is to optimize the reliability of equipment, keep equipment in functional condition, or enhance equipment for future needs, that may require purchasing accessories in order to generate accurate insight. You must consider various sources of information across the departments, such as:
- Asset type, including critical assets, expensive assets, low-priority assets, and assets that are likely to fail frequently.
- Activity associated with the asset, such as action taken on the asset, warranties, downtime, depreciation, and spare parts inventory.
- Number of service technicians, including experts, veterans, and novices.
- Compliance, such as validations, approvals, inspections, policies, and regulations.
The interdependence of these variables requires you to link your data from one variable to another to get a clear picture of maintenance operations. For instance, you need experts to handle your critical, expensive assets, which would increase the resource management cost. In that case, if your purpose is to improve the productivity of service teams, having the following information in hand is a major driving factor to make successful decisions:
- How many service technicians are experts in their job?
- How many technicians need skill-based specialized training?
- How much time is required by novice technicians to become productive?
- How many jobs can a technician complete in a given period of time, such as an average day or week?
- What assets do technicians spend most of their time on?
- Is there any asset that is beyond maintenance, that is, where replacement would be a more cost-effective solution?
- What are the most-used spare parts and tools in the inventory?
An evaluation of well-linked datasets might reveal to you that although every technician is working optimal hours, productivity is low because spare parts are not available when needed. You may find that your technicians are spending a huge amount of time servicing non-critical assets, or that novice technicians are not trained properly, which may come to light through the number of tasks they perform that do not meet the required standards. All of these things could affect your customers' experience.
IntegrationsWhen one refers to asset data, it covers every aspect of the asset-what, who, when, and how. While most MRO companies yearn for a unified view of all these aspects of maintenance operations, there is rarely any all-in-one tool available, not until you are willing to spend a fortune.
Another option is to use data integration tools to get a unified view of maintenance operations. These tools can integrate data from computerized maintenance management systems (CMMSs), field service management software, accounting software, and enterprise resource planning (ERP) software on a centralized platform, offering a 360-degree view of operations. Centralized platforms increase accessibility of critical data within teams, while making it easier for them to identify patterns and spot anomalies.
Quality of DataData-driven organizations are often accused of extensively focusing on volume of data and overlooking efforts to maintain the quality of data. Such practices add to the complexity, as with time, master data gets corrupted with inaccurate, inconsistent, outdated, incomplete, and duplicate information.
Poor data quality can totally derail the whole purpose of data collection-to generate insight and use it to make decisions, improvise strategies, and remove gaps in the processes. How can one make effective strategies with false or incomplete information in hand? To generate accurate insight, your datasets must have the following attributes:
- Completeness. The dataset contains all the requisite information.
- Accuracy. The data reflects the real-world value.
- Consistency. The data across the systems represents the same information for any entity.
- Timeliness. The data reflects the real-world value in a timeframe.
- Uniqueness. There are no duplicate records across data systems.
- Integrity. All data in the database can be traced and connected to other data.
In order to ensure that datasets have these attributes, you need to set up processes for data quality control. This includes creating a team to clean and manage data. In the end, only authentic, reliable data should be used to make maintenance strategies, and that calls for a systematic approach to collection, storage, and analysis of data.
-Bhupendra Choudhary is the business head of FieldCircle, a field service management software company. He helps businesses streamline their operations, improve transparency, and operate at high efficiency to maximize productivity.
The post Using Asset Data to Create Effective Maintenance Strategies appeared first on POWER Magazine.