2021 was the year of monster AI models
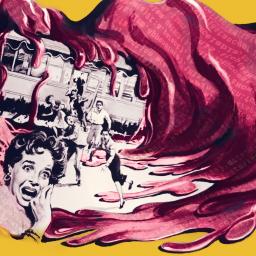
It's been a year of supersized AI models.
When OpenAI released GPT-3, in June 2020, the neural network's apparent grasp of language was uncanny. It could generate convincing sentences, converse with humans, and even autocomplete code. GPT-3 was also monstrous in scale-larger than any other neural network ever built. It kicked off a whole new trend in AI, one in which bigger is better.
Despite GPT-3's tendency to mimic the bias and toxicity inherent in the online text it was trained on, and even though an unsustainably enormous amount of computing power is needed to teach such a large model its tricks, we picked GPT-3 as one of our breakthrough technologies of 2020-for good and ill.
But the impact of GPT-3 became even clearer in 2021. This year brought a proliferation of large AI models built by multiple tech firms and top AI labs, many surpassing GPT-3 itself in size and ability. How big can they get, and at what cost?
To support MIT Technology Review's journalism, please consider becoming a subscriber.
GPT-3 grabbed the world's attention not only because of what it could do, but because of how it did it. The striking jump in performance, especially GPT-3's ability to generalize across language tasks that it had not been specifically trained on, did not come from better algorithms (although it does rely heavily on a type of neural network invented by Google in 2017, called a transformer), but from sheer size.
We thought we needed a new idea, but we got there just by scale," said Jared Kaplan, a researcher at OpenAI and one of the designers of GPT-3, in a panel discussion in December at NeurIPS, a leading AI conference.
We continue to see hyperscaling of AI models leading to better performance, with seemingly no end in sight," a pair of Microsoft researchers wrote in October in a blog post announcing the company's massive Megatron-Turing NLG model, built in collaboration with Nvidia.
What does it mean for a model to be large? The size of a model-a trained neural network-is measured by the number of parameters it has. These are the values in the network that get tweaked over and over again during training and are then used to make the model's predictions. Roughly speaking, the more parameters a model has, the more information it can soak up from its training data, and the more accurate its predictions about fresh data will be.
GPT-3 has 175 billion parameters-10 times more than its predecessor, GPT-2. But GPT-3 is dwarfed by the class of 2021. Jurassic-1, a commercially available large language model launched by US startup AI21 Labs in September, edged out GPT-3 with 178 billion parameters. Gopher, a new model released by DeepMind in December, has 280 billion parameters. Megatron-Turing NLG has 530 billion. Google's Switch-Transformer and GLaM models have one and 1.2 trillion parameters, respectively.
The trend is not just in the US. This year the Chinese tech giant Huawei built a 200-billion-parameter language model called PanGu. Inspur, another Chinese firm, built Yuan 1.0, a 245-billion-parameter model. Baidu and Peng Cheng Laboratory, a research institute in Shenzhen, announced PCL-BAIDU Wenxin, a model with 280 billion parameters that Baidu is already using in a variety of applications, including internet search, news feeds, and smart speakers. And the Beijing Academy of AI announced Wu Dao 2.0, which has 1.75 trillion parameters.
Meanwhile, South Korean internet search firm Naver announced a model called HyperCLOVA, with 204 billion parameters.
Every one of these is a notable feat of engineering. For a start, training a model with more than 100 billion parameters is a complex plumbing problem: hundreds of individual GPUs-the hardware of choice for training deep neural networks-must be connected and synchronized, and the training data split must be into chunks and distributed between them in the right order at the right time.
Large language models have become prestige projects that showcase a company's technical prowess. Yet few of these new models move the research forward beyond repeating the demonstration that scaling up gets good results.
There are a handful of innovations. Once trained, Google's Switch-Transformer and GLaM use a fraction of their parameters to make predictions, so they save computing power. PCL-Baidu Wenxin combines a GPT-3-style model with a knowledge graph, a technique used in old-school symbolic AI to store facts. And alongside Gopher, DeepMind released RETRO, a language model with only 7 billion parameters that competes with others 25 times its size by cross-referencing a database of documents when it generates text. This makes RETRO less costly to train than its giant rivals.
Yet despite the impressive results, researchers still do not understand exactly why increasing the number of parameters leads to better performance. Nor do they have a fix for the toxic language and misinformation that these models learn and repeat. As the original GPT-3 team acknowledged in a paper describing the technology: Internet-trained models have internet-scale biases."
DeepMind claims that RETRO's database is easier to filter for harmful language than a monolithic black-box model, but it has not fully tested this. More insight may come from the BigScience initiative, a consortium set up by AI company Hugging Face, which consists of around 500 researchers-many from big tech firms-volunteering their time to build and study an open-source language model.
In a paper published at the start of the year, Timnit Gebru and her colleagues highlighted a series of unaddressed problems with GPT-3-style models: We ask whether enough thought has been put into the potential risks associated with developing them and strategies to mitigate these risks," they wrote.
For all the effort put into building new language models this year, AI is still stuck in GPT-3's shadow. In 10 or 20 years, large-scale models will be the norm," said Kaplan during the NeurIPS panel. If that's the case, it is time researchers focused not only on the size of a model but on what they do with it.