AI Isn’t Artificial or Intelligent
Krystal Kauffman has been a Turker for the last seven years. She works on Mechanical Turk (MTurk), an Amazon-owned crowdsourcing website that allows businesses to hire workers to perform various tasks for compensation. Many of these tasks, Kauffman says, have been training AI projects.
In the past, we've worked on several large AI projects. So there are tasks where people just need to simply repeat the same phrase six times, so it's training AI to recognize different voices and things," Kauffman told Motherboard. So I kind of get to do a little bit of everything, but there is definitely a lot of machine learning, AI data labeling out there right now. We've been seeing an increase in those requesters that are listing the work."
Kauffman is part of the large labor force powering AI, doing jobs that include looking through large datasets to label images, filter NSFW content, and annotate objects in images and videos. These tasks, deemed rote and unglamorous for many in-house developers, are often outsourced to gig workers and workers who largely live in South Asia and Africa and work for data training companies such as iMerit, Sama, and Alegion. For example, Facebook has one of the most advanced algorithmic content moderation systems on the internet. That system's so-called artificial intelligence, though, is "learning from thousands of human decisions" made by human moderators.
Large companies like Meta and Amazon boast robust AI development teams and claim that AI is at the forefront of their work. Meta writes our future depends on our ability to leverage the newest AI technology at scale," and Amazon encourages customers to innovate faster with the most comprehensive set of AI and [Machine Learning] services."
We're used to working on things that we just don't know exactly what they are for [...] we know we're working on some of those big tech devices. And while I don't need to be called an employee or anything like that, you very rarely hear a big tech company acknowledge the invisible workforce that is behind a lot of this technology."
The biggest tech companies in the world imagine a near future where AI will replace a lot of human labor, unleashing new efficiency and productivity. But this vision ignores the fact that much of what we think of as AI" is actually powered by tedious, low-paid human labor.
I think one of the mythologies around AI computing is that they actually work as intended. I think right now, what human labor is compensating for is essentially a lot of gaps in the way that the systems work," Laura Forlano, Associate Professor of Design at the Institute of Design at Illinois Institute of Technology, told Motherboard. On the one hand, the industry can claim that these things are happening, you know, magically behind the scenes, or that much of what's going on is the computing. However, we know that in so many different examples, whether we look at online content, how to operate an autonomous vehicle, or if we look at medical devices, human labor is being used to address the gaps in where that system really isn't able to work."
Humans like Kauffman, who help create the raw materials used to train these systems, rarely have insight into what their hard work will ultimately be used to create.
We're used to working on things that we just don't know exactly what they are for [...] we know we're working on some of those big tech devices. And while I don't need to be called an employee or anything like that, you very rarely hear a big tech company acknowledge the invisible workforce that is behind a lot of this technology," Kauffman said. They lead people to believe that AI is smarter and more advanced than where it actually is, which is [why] we're still training it every single day."
Tech companies hire tens of thousands of gig workers to maintain the illusion that their machine-learning algorithms are fully self-functional, and that each new AI tool is capable of solving a number of issues out of the box. In reality, AI development has a lot more in common with material production cycles than we realize.
I think that the public doesn't have a good awareness of the fact that this is a supply chain. It's a global supply chain, it contains uneven geographic flows and relations. And that it is based on a huge amount of human labor," Kelle Howson, a postdoctoral researcher on the Fairwork project at the Oxford Internet Institute, told Motherboard.
Howson can't say for sure whether tech companies are intentionally obscuring human AI laborers, but that doing so certainly works in their interests. I think that in some ways it supports their business models to do so because there's this perception that the work is done," said Howson. You as a client access a platform interface, post your project, and the work is delivered immediately. It's almost like magic. There was maybe never any human involved or [that's what] it feels like, and so there's a sense of efficiency. And that really goes along with the kind of narrative that Silicon Valley likes to tell. The disruption, the tech solutionism, the move fast and break things kind of ideas."
Like other global supply chains, the AI pipeline is greatly imbalanced. Developing countries in the Global South are powering the development of AI systems by doing often low-wage beta testing, data annotating and labeling, and content moderation jobs, while countries in the Global North are the centers of power benefiting from this work.
There are a lot more workers on microwork platforms in the Global South, compared to the Global North. So the majority of the labor supply on these platforms is concentrated in the Global South, whereas the majority of the demand is located in the Global North," Howson said. We know from experience with other supply chains, agri-food, textiles, that when there are these relations of outsourcing work to lower wage labor and low-income countries, often that goes along with exploitive relationships and poorer labor protections, poorer working conditions."
In a 2021 paper on the role of global labor in AI development, AI ethics researchers argued that the current inclusion of workers from the Global South in the AI pipeline is a continuation of exploitative practices-not unlike the history of colonial exploitation, where Western states took advantage of people from the Global South and their resources for access to cheap, physically tolling labor to benefit their institutions and businesses.
Essentially, people [in the Global South] often get paid what is labeled as a fair wage, just based on the GDP or the local income of their respective context. But the work is very rote and very manual and a bit tiring as well, even though you can't obviously compare it to the physical labor that was done and the plantation work throughout the colonial days," Chinasa T. Okolo, one of the paper's authors and a Ph.D. student in the Department of Computer Science at Cornell University, told Motherboard. But this work is being contracted to the same regions and similar companies as well. Content moderation definitely gets more troubling for the workers themselves, having to view different kinds of materials all day which is definitely mentally taxing for someone to be exposed to all the time. We have seen workers in these countries wage suits against employers or companies like Meta, for example, to challenge the working conditions they're forced to be in."
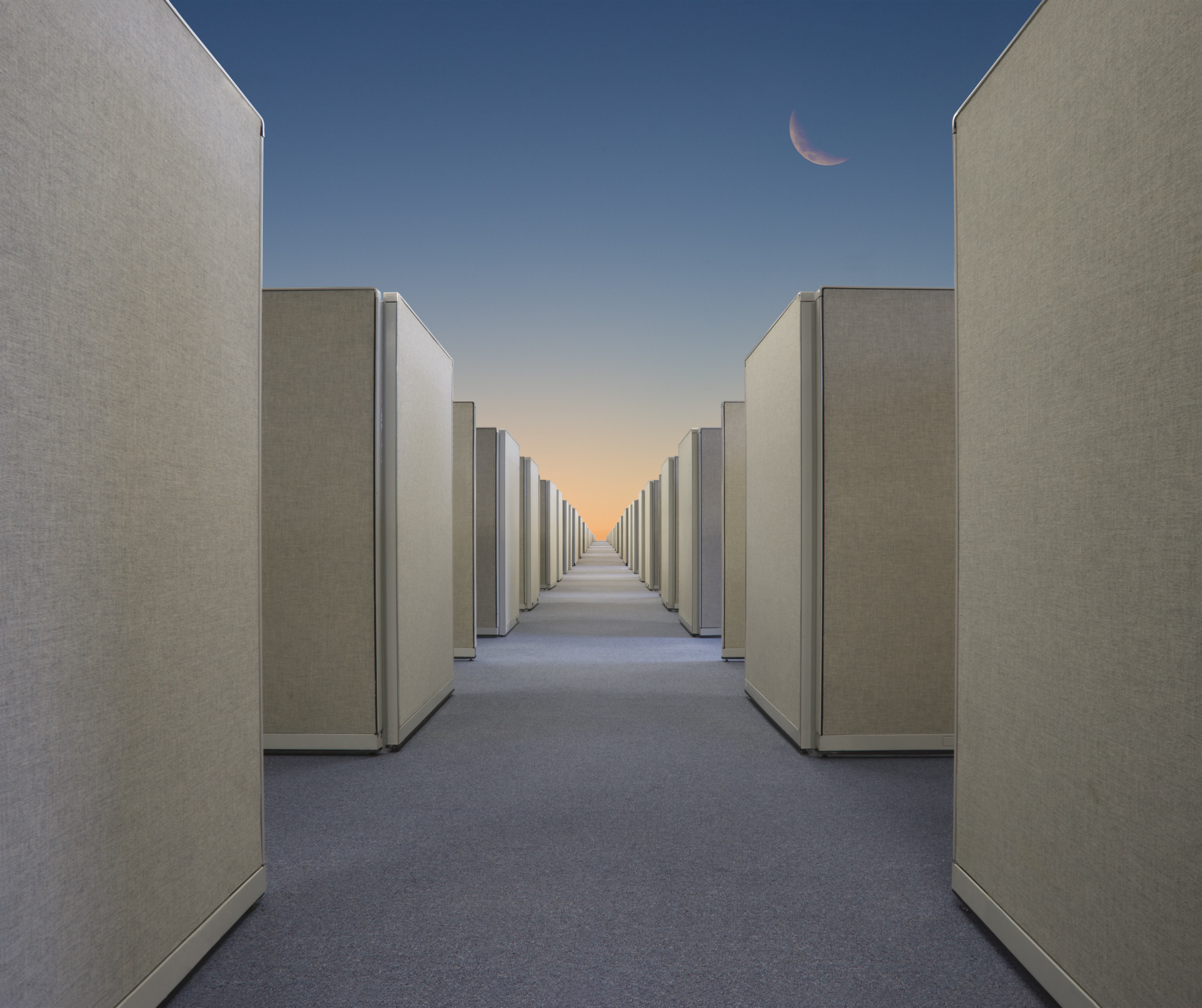
In May, a former content moderator named Daniel Motaung filed a lawsuit in Nairobi, Kenya, accusing Facebook parent company Meta and its largest outsourcing partner, Sama, of forced labor, human trafficking, and union busting. In an investigation by TIME Magazine, Sama's mission to provide poor countries with ethical" and dignified digital work" was quickly proven to be a facade of participation-washing," which is what researchers like Forlano define as companies including workers in a post-colonial structure of global power" as a form of virtue signaling, rather than having them as meaningful, democratic partners. Motaung and other Sama employees told TIME that they were taking home as little as $1.50 per hour, and at least two content moderators were diagnosed with mental illnesses such as PTSD following their work viewing graphic images and videos depicting rape, murder, and dismemberment.
The researchers note that while labeling and content moderation companies like Samasource, Scale AI, and Mighty AI operate in the United States, their labor force relies heavily on low-wage workers from sub-Saharan Africa and Southeast Asia. This leads to a significant disparity between the millions in profits earned by data labeling companies and worker earnings; for example, workers at Samasource earn around $8 USD a day while the company made $19 million in 2019," authors including Okolo wrote in a 2021 paper. While Lee notes that $8 USD may well be a living wage in certain areas, the massive profit disparity remains despite the importance of these workers to the core businesses of these companies."
Companies like Meta justify the outsourcing of labor in less developed countries by claiming that doing what they call impact-sourcing," which is when companies intentionally hire workers from disadvantaged or vulnerable populations to provide them with opportunities they otherwise don't have. But experts warn that behind this practice are unsafe and unethical working conditions that lack regulations and fail to redistribute power.
Sara Enright, project director at the Global Impact Sourcing Coalition (GISC), told MIT Technology Review, If it is solely gig work in which an individual is accessing part-time wages through an hour a day here and there, that is not impact employment, because it does not actually lead to career development and ultimately poverty alleviation."
Experts say that outsourcing these workers is advantageous for big tech companies, not only saving them money, but also making it easier for big tech companies to avoid strict judicial review. It also creates distance between the workers and the company itself, allowing it to uphold the magical and sophisticated marketing of its AI tools.
If there are labor protections and the workers' jurisdiction, it's incredibly hard to enforce them, when the client is in another country, and the platform is in a third country," Howson said. They're classified as independent contractors, so they have very little recourse to any local labor protections, and any legislative frameworks which would allow them to unionize or to engage in collective bargaining with the platforms."
Due to this structural power imbalance, workers often lack the ability to speak out about their clients, whether about ethical concerns regarding the dataset they interact with or labor violations, such as the refusal of adequate pay.
In addition to not getting an adequate wage, a lot of this labeling is rushed as well. In my personal experience using Amazon Mechanical Turk, I've had experiences with bots and people putting in spurious answers to questions. These dynamics definitely have influence on the quality of these data sets as well."
When Motherboard asked crowd worker marketplace Clickworker, which markets AI Solutions as one of the main jobs on its platform, how it makes sure workers are able to vocalize any mistreatment received from a client, a spokesperson replied, This cannot happen with us, because the Clickworkers have no contact with the customer and all handling is done by us."
It's also a product of this geographical discrimination that is enabled by the planetary labor market, where clients and workers are directly kind of connecting in real-time forming these short-term contracts and then moving on," Howson said. As a micro worker, it's not just incredibly difficult, but also probably not worth your while to contest these individual instances when they happen. Because you're spending a couple of minutes on a task. If you're not paid for that task, it takes so much longer to go through the platform's due process, mediation mechanisms than it would to just move on to the next task."
Kauffman is one of the workers leading Turkopticon, a non profit organization that advocates for Turkers' rights and brings issues to Amazon. She is currently part of the organization's effort to find justice against an AI company called AI Insights" that put over 70,000 tasks on Mechanical Turk only for the company to reject all the completed work it received. This meant that the company could keep and see the work but didn't have to pay the workers for their time. Kauffman said that after the work was rejected, the company promptly exited the platform, but its account still exists, which means if it chose to reactivate it, it could.
Normally, if a Turker's work is rejected, they can contact the client and ask why, and in some cases revise and resubmit the task. But many Turkers who reached out to AI Insights and Amazon were either ignored or told nothing could be done. As a result, Kauffman says, many workers lost work and saw their approval ratings decrease, which makes it harder to find good-paying work on the platform.
There were emails that were sent to Amazon about this particular situation, to which if a reply was given, it said that, Amazon doesn't get in the middle of requesters and workers. And so they couldn't and wouldn't do anything about it," Kauffman said. We've been trying to get it out there that this is happening and we know that Amazon has the capability to step in and fix this and they just aren't and that's really frustrating."
Kauffman explained that in order to sign up as a worker, she had to provide her social security, driver's license, and banking information in order to verify that she was a legitimate worker. In comparison, she said, requesters can input fake names and emails, and aren't held to the same verification standards. This power imbalance is largely what Turkopticon is fighting to remedy. Turkers demand the platform limit the number of rejections it will allow to affect a worker's approval rate when a requester rejects all work and to consult with their coalition of worker forums to create solutions that improve MTurk for both requesters and workers.
The overall rate at which Workers' tasks are rejected by Requesters is very low (less than one percent), and Workers have access to a number of metrics that can help them determine if they want to work on a task, including the Requester's historical record of accepting tasks," An Amazon Web Services spokesperson told Motherboard. MTurk continues to help a wide range of Workers earn money and contribute to the growth of their communities." Amazon also said that it monitors mass rejections and that it has made improvements to its process since the AI Insights incident.
Howson told Motherboard that there is a lot of unpaid labor built into the cloud working economy on gig work platforms like Mechanical Turk and Clickworker. In addition to the low and unguaranteed compensations, workers on these platforms have to spend a lot of time refreshing their home screens, bidding and searching for jobs, and racing to be the first ones to accept the jobs if chosen. The number of cloud workers also far outweighs the amount of work available and creates high levels of competition on the platforms. Clients are thus able to easily take advantage of the accessibility and abundance of cheap work. This, too, disproportionately affects crowd workers in the Global South, who have a more difficult time accessing tasks and job opportunities and have a greater ratio of unpaid to paid labor time, according to Howson's research.
Though this quick turnaround for work meets the industry model of creating more and faster, such practices also call into question how effective the work is for clients themselves. Low-paying conditions not only affect workers but also in turn sets up the greater probability that clients will receive lower quality work.
When the workers especially on Amazon Mechanical Turk are assigned tasks, they are beholden to the person who creates a task for them to get paid," Okolo said. In addition to not getting an adequate wage, a lot of this labeling is rushed as well. In my personal experience using Amazon Mechanical Turk, I've had experiences with bots and people putting in spurious answers to questions. These dynamics definitely have influence on the quality of these data sets as well."
There are ways to certainly pay to ensure better quality. And that's probably one of the first ways into thinking about this is that, to the extent that AI relies on consistency and quality, if you're not paying for it, you're not going to get that," Jennifer King, the Privacy and Data Policy Fellow at the Stanford Institute for Human-Centered Artificial Intelligence (HAI), told Motherboard. I think this is a lot of these incentives around how products are developed, where the engineering and the AI modeling pieces are the best paid and most desirable, [while] data labeling really takes a backseat."
Kauffman wants to push back against the assumption that jobs like data labeling are unskilled" just because are low-paid and involve repetitive tasks. She explained that workers are required to have certain qualifications and take tests in order to access certain tasks.
People now have skills and knowledge that they didn't have prior to taking this test and reviewing the materials. So this idea that people are uneducated, [after] we've learned so many different things about so many different topics is unreal," Kauffman said. There is a constant learning there where anyone couldn't just sit down and do without picking up additional skills."
Many technology users don't realize that they are implicated in the AI pipeline as well. Most of us have performed unpaid labor in training AI systems, whether we are solving a CAPTCHA to prove we're not a robot, or guiding autonomous cars away from roadblocks that the vehicle can't identify. As a 2020 study co-authored by Forlano notes: Users also improve the performance of ML models as they interact with them, a single unanticipated click can update a model's parameters and future accuracy. This work sometimes is so deeply integrated into the ways in which users navigate the Internet that it is performed unconsciously, e.g. when using Google Maps and producing data movement patterns that enable traffic predictions. But other times it becomes more conscious, e.g. when classifying photos when completing a reCAPTCHA, or ranking Uber drivers."
More recently, AI text-to-image generators like DALL-E 2, Midjourney, and Stable Diffusion, and language prediction AI models like GPT-3 have all demonstrated astonishing predictive capabilities. Yet these tools also benefit from the same relationship between human labor and AI training.
These large language models, which generate an image or produce text based on a user's input prompt, are trained using deep learning methods. This means that these models are capable of mimicking the way our brains work by parsing through layers and layers of human-created data to come up with an appropriate image or text result. These datasets are all products of human labor.
For example, Stable Diffusion was trained on the LAION-5B open source dataset, a collection of 5.8 billion images and captions scraped from the internet. The images and captions are all products of unpaid human labor, from people coding and designing websites to users uploading and posting images on them. While the predictive frameworks of AI models such as Generative Adversarial Networks (GANs) have been advancing at an extremely rapid pace, the models have become so massive and complicated that their outputs are virtually impossible to explain. This is why bias and racist stereotypes are so common in the outputs of AI systems. When you build a system with billions of parameters and training examples, it simply mirrors the biases of the aggregate data-and a disconnect emerges between what the system was built upon and what AI experts hope it can do.
There's a gap between the knowledge that computer scientists have about the system and the knowledge that the sociologists might have about the system, in that they're coming from very different places," Forlano said. And so even within communities that work on AI, there have been accounts in many mainstream newspapers that even computer scientists that work on these systems don't always know how the systems are coming to the conclusions that they're coming to."
But Forlano emphasizes that the problem is more fundamental, and can't be solved by simply adding more data to improve the system. One of the logical conclusions that a computer scientist might come to is that if you just add more and correct data to the systems that ultimately they will become better. But that in itself is a fallacy. No amount of data is going to fix the systems."
By highlighting the ways in which human labor underlines much of the AI pipeline, AI experts and researchers hope to dismantle the move fast and break things" attitude that rules technological processes and exploits the underlying workers. Most people can agree on the fact that humans will always be part of AI, from developing models to checking for certain biases and errors. Thus, AI experts argue that the focus should be on how to decolonize the AI development process and include humans in an ethical and sustainable way.
We need to think seriously about the human labor in the loop driving AI. This workforce deserves training, support and compensation for being at-the-ready and willing to do an important job that many might find tedious or too demanding," Mary L. Gray and Siddharth Suri, authors of the book Ghost Work: How to Stop Silicon Valley from Building a New Global Underclass, wrote in a 2017 article for the Harvard Business Review.
Some of the concrete steps the authors recommend include requiring more transparency from tech companies, creating policies that improve working conditions and wages for data trainers, and providing workers with education opportunities that allow them to contribute to the AI models in ways besides labeling.
Marie-Therese Png, a doctoral student at the Oxford Internet Institute and research intern at DeepMind Ethics and Society, proposed in her research that the AI governance process needs to be restructured to include the Global South as a co-governor." This means acknowledging the colonial power asymmetries that are replicated in the AI pipeline, and giving actors from the Global South influence over agenda-setting, decision-making, and resource power."
This is similar to what Forlano and her co-authors argue in their paper. They describe a design-with" mentality rather than a design for" one, in which companies lack consultation and representation from groups that are affected by the AI system. Experts do not often have a good understanding of how to design effective participatory processes or engage the right stakeholders to achieve the desired outcomes," the authors wrote. Participation workshops can become performative, where experts do not actually take the needs or recommendations of the different stakeholder groups into consideration."
The study's authors suggest that all participants in training AI should be recognized as work, which gives everyday users the ability to opt-in or opt-out of free online labor practices that would train a Machine Learning (ML) system. And if they choose to opt-in, they should be compensated accordingly or be provided with greater incentives. People should be compensated for the work that they do to improve systems," Forlano said. And that if it's not done in an equitable way, it's just another kind of exploitation."
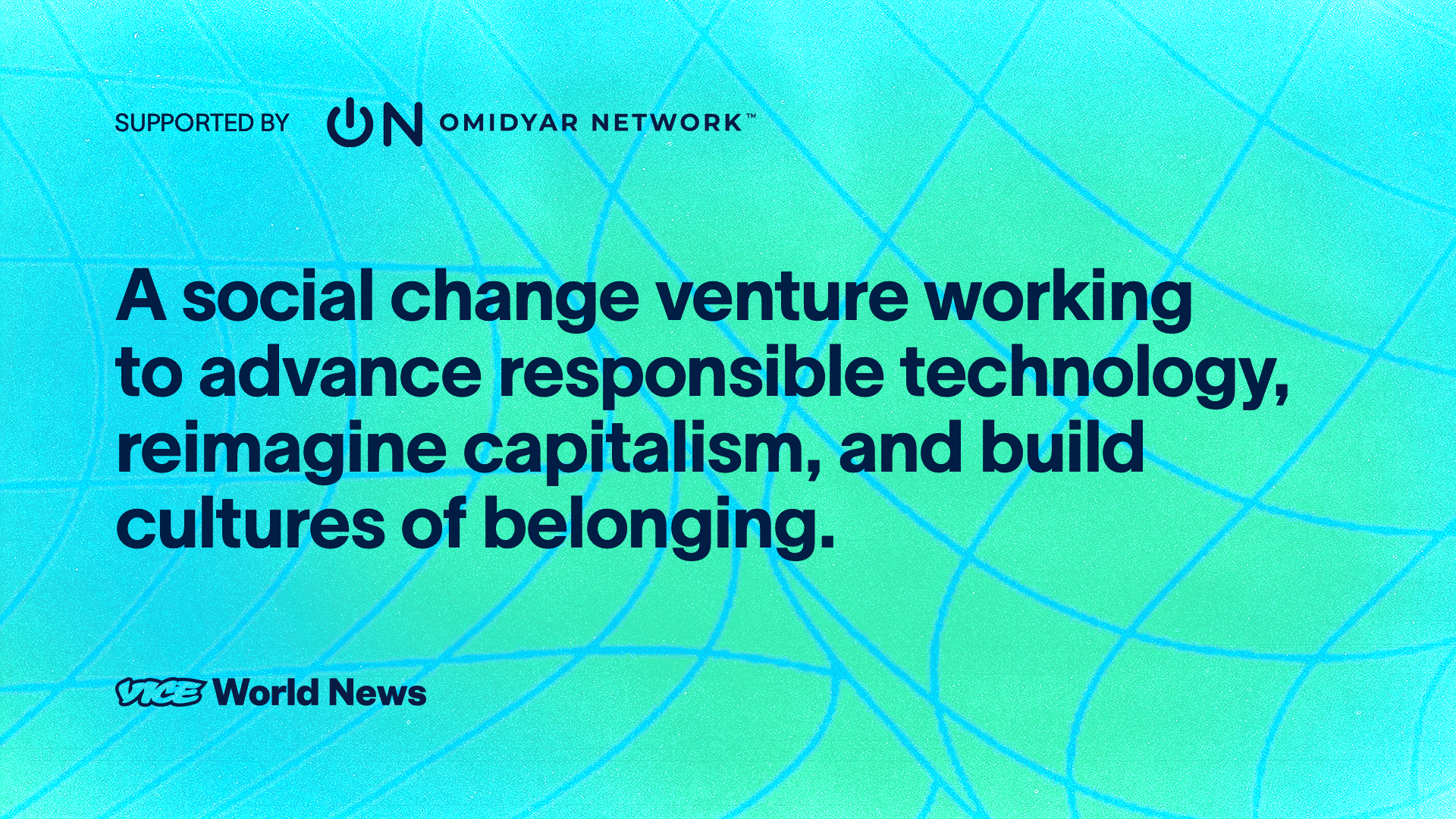