Drones That Can Fly Better Than You Can
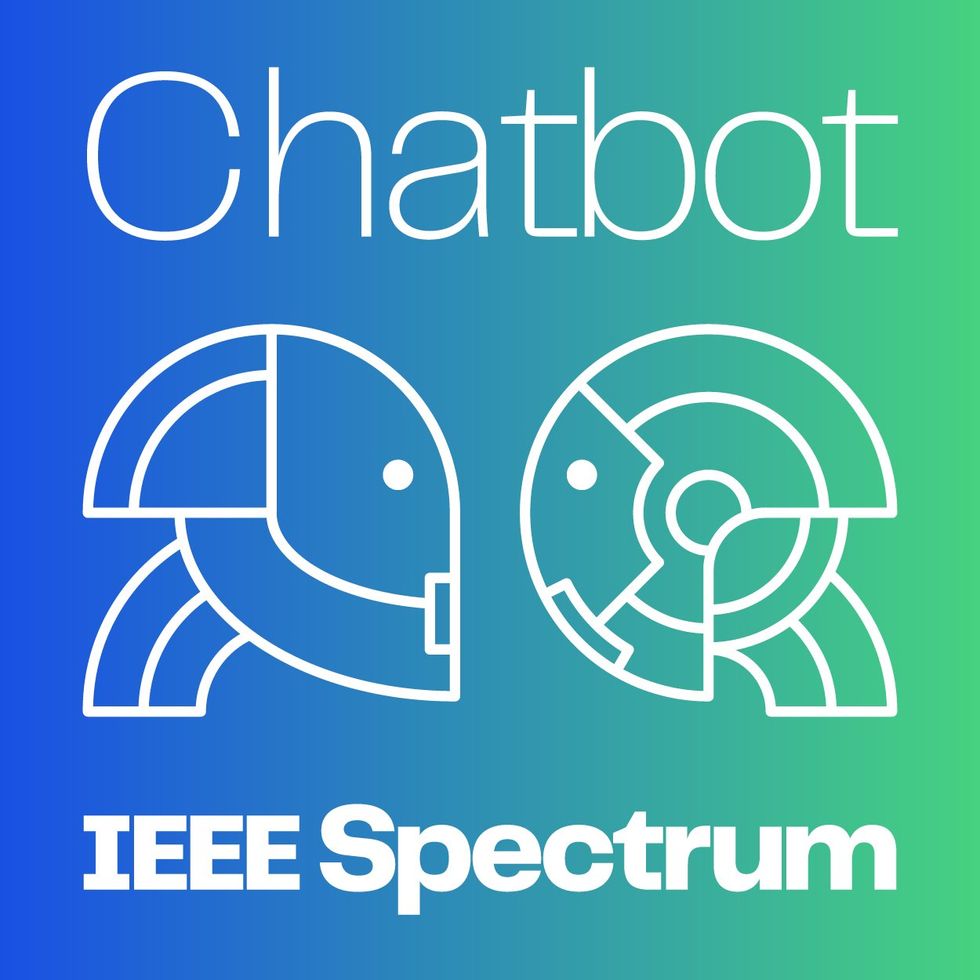
Episode 3: Drones That Can Fly Better Than You Can
Evan Ackerman: I'm Evan Ackerman, and welcome to Chatbot, a new podcast from IEEE Spectrum where robotics experts interview each other about things that they find fascinating. On this episode of Chatbot, we'll be talking with Davide Scaramuzza and Adam Bry about agile autonomous drones. Adam Bry is the CEO of Skydio, a company that makes consumer camera drones with an astonishing amount of skill at autonomous tracking and obstacle avoidance. Foundation for Skydio's drones can be traced back to Adam's work on autonomous agile drones at MIT, and after spending a few years at Google working on Project Wing's delivery drones, Adam cofounded Skydio in 2014. Skydio is currently on their third generation of consumer drones, and earlier this year, the company brought on three PhD students from Davide's lab to expand their autonomy team. Davide Scaramuzza directs the Robotics and Perception group at the University of Zurich. His lab is best known for developing extremely agile drones that can autonomously navigate through complex environments at very high speeds. Faster, it turns out, than even the best human drone racing champions. Davide's drones rely primarily on computer vision, and he's also been exploring potential drone applications for a special kind of camera called an event camera, which is ideal for fast motion under challenging lighting conditions. So Davide, you've been doing drone research for a long time now, like a decade, at least, if not more.
Davide Scaramuzza: Since 2009. 15 years.
Ackerman: So what still fascinates you about drones after so long?
Scaramuzza: So what fascinates me about drones is their freedom. So that was the reason why I decided, back then in 2009, to actually move from ground robots-I was working at the time on self-driving cars-to drones. And actually, the trigger was when Google announced the self-driving car project, and then for me and many researchers, it was clear that actually many things were now transitioning from academia to industry, and so we had to come up with new ideas and things. And then with my PhD adviser at that time [inaudible] we realized, actually, that drones, especially quadcopters, were just coming out, but they were all remote controlled or they were actually using GPS. And so then we said, What about flying drones autonomously, but with the onboard cameras?" And this had never been done until then. But what fascinates me about drones is the fact that, actually, they can overcome obstacles on the ground very quickly, and especially, this can be very useful for many applications that matter to us all today, like, first of all, search and rescue, but also other things like inspection of difficult infrastructures like bridges, power [inaudible] oil platforms, and so on.
Ackerman: And Adam, your drones are doing some of these things, many of these things. And of course, I am fascinated by drones and by what your drone is able to do, but I'm curious. When you introduce it to people who have maybe never seen it, how do you describe, I guess, almost the magic of what it can do?
Adam Bry: So the way that we think about it is pretty simple. Our basic goal is to build in the skills of an expert pilot into the drone itself, which involves a little bit of hardware. It means we need sensors that see everything in every direction and we need a powerful computer on board, but is mostly a software problem. And it becomes quite application-specific. So for consumers, for example, our drones can follow and film moving subjects and avoid obstacles and create this incredibly compelling dynamic footage. And the goal there is really what would happen if you had the world's best drone pilot flying that thing, trying to film something in an interesting, compelling way. We want to make that available to anybody using one of our products, even if they're not an expert pilot, and even if they're not at the controls when it's flying itself. So you can just put it in your hand, tell it to take off, it'll turn around and start tracking you, and then you can do whatever else you want to do, and the drone takes care of the rest. In the industrial world, it's entirely different. So for inspection applications, say, for a bridge, you just tell the drone, Here's the structure or scene that I care about," and then we have a product called 3D Scan that will automatically explore it, build a real-time 3D map, and then use that map to take high-resolution photos of the entire structure.
And to follow on a bit to what Davide was saying, I mean, I think if you sort of abstract away a bit and think about what capability do drones offer, thinking about camera drones, it's basically you can put an image sensor or, really, any kind of sensor anywhere you want, any time you want, and then the extra thing that we're bringing in is without needing to have a person there to control it. And I think the combination of all those things together is transformative, and we're seeing the impact of that in a lot of these applications today, but I think that that really- realizing the full potential is a 10-, 20-year kind of project.
Ackerman: It's interesting when you talk about the way that we can think about the Skydio drone is like having an expert drone pilot to fly this thing, because there's so much skill involved. And Davide, I know that you've been working on very high-performance drones that can maybe challenge even some of these expert pilots in performance. And I'm curious, when expert drone pilots come in and see what your drones can do autonomously for the first time, is it scary for them? Are they just excited? How do they react?
Scaramuzza: First of all, actually, they say, Wow." So they can not believe what they see. But then they get super excited, but at the same time, nervous. So we started working on autonomous drone racing five years ago, but in the first three years, we have been flying very slowly, like three meters per second. So they were really snails. But then in the last two years is when actually we started really pushing the limits, both in control and planning and perception. So these are our most recent drone, by the way. And now we can really fly at the same level of agility as humans. Not yet at the level to beat human, but we are very, very close. So we started the collaboration with Marvin, who is the Swiss champion, and he's only- now he's 16 years old. So last year he was 15 years old. So he's a boy. And he actually was very mad at the drone. So he was super, super nervous when he saw this. So he didn't even smile the first time. He was always saying, I can do better. I can do better." So actually, his reaction was quite scared. He was scared, actually, by what the drone was capable of doing, but he knew that, basically, we were using the motion capture. Now [inaudible] try to play in a fair comparison with a fair setting where both the autonomous drone and the human-piloted drone are using both onboard perceptions or egocentric vision, then things might end up differently.
Because in fact, actually, our vision-based drone, so flying with onboard vision, was quite slow. But actually now, after one year of pushing, we are at a level, actually, that we can fly a vision-based drone at the level of Marvin, and we are even a bit better than Marvin at the current moment, using only onboard vision. So we can fly- in this arena, the space allows us to go up to 72 kilometers per hour. We reached the 72 kilometers per hour, and we even beat Marvin in three consecutive laps so far. So that's [inaudible]. But we want to now also compete against other pilots, other world champions, and see what's going to happen.
Ackerman: Okay. That's super impressive.
Bry: Can I jump in and ask a question?
Ackerman: Yeah, yeah, yeah.
Bry: I'm interested if you- I mean, since you've spent a lot of time with the expert pilots, if you learn things from the way that they think and fly, or if you just view them as a benchmark to try to beat, and the algorithms are not so much inspired by what they do.
Scaramuzza: So we did all these things. So we did it also in a scientific manner. So first, of course, we interviewed them. We asked any sort of question, what type of features are you actually focusing your attention, and so on, how much is the people around you, the supporters actually influencing you, and the hearing the other opponents actually screaming while they control [inaudible] influencing you. So there is all these psychological effects that, of course, influencing pilots during a competition. But then what we tried to do scientifically is to really understand, first of all, what is the latency of a human pilot. So there have been many studies that have been done for car racing, Formula One, back in the 80s and 90s. So basically, they put eye trackers and tried to understand- they tried to understand, basically, what is the latency between what you see until basically you act on your steering wheel. And so we tried to do the same for human pilots. So we basically installed an eye tracking device on our subjects. So we called 20 subjects from all across Switzerland, some people also from outside Switzerland, with different levels of expertise.
But they were quite good. Okay? We are not talking about median experts, but actually already very good experts. And then we would let them rehearse on the track, and then basically, we were capturing their eye gazes, and then we basically measured the time latency between changes in eye gaze and changes in throttle commands on the joystick. And we measured, and this latency was 220 milliseconds.
Ackerman: Wow. That's high.
Scaramuzza: That includes the brain latency and the behavioral latency. So that time to send the control commands, once you process the information, the visual information to the fingers. So-
Bry: I think [crosstalk] it might just be worth, for the audience anchoring that, what's the typical control latency for a digital control loop. It's- I mean, I think it's [crosstalk].
Scaramuzza: It's typically in the- it's typically in the order of- well, from images to control commands, usually 20 milliseconds, although we can also fly with the much higher latencies. It really depends on the speed you want to achieve. But typically, 20 milliseconds. So if you compare 20 milliseconds versus the 220 milliseconds of the human, you can already see that, eventually, the machine should beat the human. Then the other thing that you asked me was, what did we learn from human pilots? So what we learned was- interestingly, we learned that basically they were always pushing the throttle of the joystick at the maximum thrust, but actually, this is-
Bry: Because that's very consistent with optimal control theory.
Scaramuzza: Exactly. But what we then realized, and they told us, was that it was interesting for them to observe that actually, for the AI, was better to brake earlier rather than later as the human was actually doing. And we published these results in Science Robotics last summer. And we did this actually using an algorithm that computes the time optimal trajectory from the start to the finish through all the gates, and by exploiting the full quadrotor dynamical model. So it's really using not approximation, not point-mass model, not polynomial trajectories. The full quadrotor model, it takes a lot to compute, let me tell you. It takes like one hour or more, depending on the length of the trajectory, but it does a very good job, to a point that Gabriel Kocher, who works for the Drone Racing League, told us, Ah, this is very interesting. So I didn't know, actually, I can push even faster if I start braking before this gate."
Bry: Yeah, it seems like it went the other way around. The optimal control strategy taught the human something.
Ackerman: Davide, do you have some questions for Adam?
Scaramuzza: Yes. So since you mentioned that basically, one of the scenarios or one of the applications that you are targeting, it is basically cinematography, where basically, you want to take amazing shots at the level of Hollywood, maybe producers, using your autonomous drones. And this is actually very interesting. So what I want to ask you is, in general, so going beyond cinematography, if you look at the performance of autonomous drones in general, it still looks to me that, for generic applications, they are still behind human pilot performance. I'm thinking of beyond cinematography and beyond the racing. I'm thinking of search and rescue operations and many things. So my question to Adam is, do you think that providing a higher level of agility to your platform could potentially unlock new use cases or even extend existing use cases of the Skydio drones?
Bry: You're asking specifically about agility, flight agility, like responsiveness and maneuverability?
Scaramuzza: Yes. Yes. Exactly.
Bry: I think that it is- I mean, in general, I think that most things with drones have this kind of product property where the more you get better at something, the better it's going to be for most users, and the more applications will be unlocked. And this is true for a lot of things. It's true for some things that we even wish it wasn't true for, like flight time. Like the longer the flight time, the more interesting and cool things people are going to be able to do with it, and there's kind of no upper limit there. Different use cases, it might taper off, but you're going to unlock more and more use cases the longer you can fly. I think that agility is one of these parameters where the more, the better, although I will say it's not the thing that I feel like we're hitting a ceiling on now in terms of being able to provide value to our users. There are cases within different applications. So for example, search and rescue, being able to fly through a really tight gap or something, where it would be useful. And for capturing cinematic videos, similar story, like being able to fly at high speed through some really challenging course, where I think it would make a difference. So I think that there are areas out there in user groups that we're currently serving where it would matter, but I don't think it's like the- it's not the thing that I feel like we're hitting right now in terms of sort of the lowest-hanging fruit to unlock more value for users. Yeah.
Scaramuzza: So you believe, though, that in the long term, actually achieving human-level agility would actually be added value for your drones?
Bry: Definitely. Yeah. I mean, one sort of mental model that I think about for the long-term direction of the products is looking at what birds can do. And the agility that birds have and the kinds of maneuvers that that makes them capable of, and being able to land in tricky places, or being able to slip through small gaps, or being able to change direction quickly, that affords them capability that I think is definitely useful to have in drones and would unlock some value. But I think the other really interesting thing is that the autonomy problem spans multiple sort of ranges of hierarchy, and when you get towards the top, there's human judgment that I think is very- I mean, it's crucial to a lot of things that people want to do with drones, and it's very difficult to automate, and I think it's actually relatively low value to automate. So for example, in a search and rescue mission, a person might have- a search and rescue worker might have very particular context on where somebody is likely to be stuck or maybe be hiding or something that would be very difficult to encode into a drone. They might have some context from a clue that came up earlier in the case or something about the environment or something about the weather.
And so one of the things that we think a lot about in how we build our products-we're a company. We're trying to make useful stuff for people, so we have a pretty pragmatic approach on these fronts- is basically- we're not religiously committed to automating everything. We're basically trying to automate the things where we can give the best tool to somebody to then apply the judgment that they have as a person and an operator to get done what they want to get done.
Scaramuzza: And actually, yeah, now that you mentioned this, I have another question. So I've watched many of your previous tech talks and also interacted with you guys at conferences. So what I learned-and correct me if I'm wrong-is that you're using a lot of deep learning on the perception side, so as part of a 3D construction, semantic understanding. But it seems to me that on the control and planning side, you're still relying basically on optimal control. And I wanted to ask you, so if this is the case, are you happy there with optimal control? We also know that Boston Dynamics is actually using only optimal control. Actually, they even claim they are not using any deep learning in control and planning. So is this actually also what you experience? And if this is the case, do you believe in the future, actually, you will be using deep learning also in planning and control, and where exactly do you see the benefits of deep learning there?
Bry: Yeah, that's a super interesting question. So what you described at a high level is essentially right. So our perception stack- and we do a lot of different things in perception, but we're pretty heavily using deep learning throughout, for semantic understanding, for spatial understanding, and then our planning and control stack is based on more conventional kind of optimal control optimization and full-state feedback control techniques, and it generally works pretty well. Having said that, we did- we put out a blog post on this. We did a research project where we basically did end-to-end- pretty close to an end-to-end learning system where we replaced a good chunk of the planning stack with something that was based on machine learning, and we got it to the point where it was good enough for flight demonstrations. And for the amount of work that we put into it, relative to the capability that we got, I think the results were really compelling. And my general outlook on this stuff- I think that the planning and controls is an area where the models, I think, provide a lot of value. Having a structured model based on physics and first principles does provide a lot of value, and it's admissible to that kind of modeling. You can write down the mass and the inertia and the rotor parameters, and the physics of quadcopters are such that those things tend to be pretty accurate and tend to work pretty well, and by starting with that structure, you can come up with quite a capable system.
Having said that, I think that the- to me, the trajectory of machine learning and deep learning is such that eventually I think it will dominate almost everything, because being able to learn based on data and having these representations that are incredibly flexible and can encode sort of subtle relationships that might exist but wouldn't fall out of a more conventional physics model, I think is really powerful, and then I also think being able to do more end-to-end stuff where subtle sort of second- or third-order perception impact- or second- or third-order perception or real world, physical world things can then trickle through into planning and control actions, I think is also quite powerful. So generally, that's the direction I see us going, and we've done some research on this. And I think the way you'll see it going is we'll use sort of the same optimal control structure we're using now, but we'll inject more learning into it, and then eventually, the thing might evolve to the point where it looks more like a deep network in end-to-end.
Scaramuzza: Now, earlier you mentioned that you foresee that in the future, drones will be flying more agilely, similar to human pilots, and even in tight spaces. You mentioned passing through a narrow gap or even in a small corridor. So when you navigate in tight spaces, of course, ground effect is very strong. So do you guys then model these aerodynamic effects, ground effect- not just ground effect. Do you try to model all possible aerodynamic effects, especially when you fly close to structures?
Bry: It's an interesting question. So today we don't model- we estimate the wind. We estimate the local wind velocity-and we've actually found that we can do that pretty accurately-around the drone, and then the local wind that we're estimating gets fed back into the control system to compensate. And so that's kind of like a catch-all bucket for- you could think about ground effect as like a variation- this is not exactly how it works, obviously, but you could think about it as like a variation in the local wind, and our response times on those, like the ability to estimate wind and then feed it back into control, is pretty quick, although it's not instantaneous. So if we had like a feed forward model where we knew as we got close to structures, This is how the wind is likely to vary," we could probably do slightly better. And I think you're- what you're pointing at here, I basically agree with. I think the more that you kind of try to squeeze every drop of performance out of these things you're flying with maximum agility in very dense environments, the more these things start to matter, and I could see us wanting to do something like that in the future, and that stuff's fun. I think it's fun when you sort of hit the limit and then you have to invent better new algorithms and bring more information to bear to get the performance that you want.
On this- perhaps related. You can tell me. So you guys have done a lot of work with event cameras, and I think that you were- this might not be right, but from what I've seen, I think you were one of the first, if not the first, to put event cameras on quadcopters. I'd be very interested in- and you've probably told these stories a lot, but I still think it'd be interesting to hear. What steered you towards event cameras? How did you find out about them, and what made you decide to invest in research in them?
Scaramuzza: [crosstalk] first of all, let me explain what an event camera is. An event camera is a camera that has also pixels, but differently from a standard camera, an event camera only sends information when there is motion. So if there is no motion, then the camera doesn't stream any information. Now, the camera does this through smart pixels, differently from a standard camera, where every pixel triggers information the same time at equidistant time intervals. In an event camera, the pixels are smart, and they only trigger information whenever a pixel detects motion. Usually, a motion is recorded as a change of intensity. And the stream of events happens asynchronously, and therefore, the byproduct of this is that you don't get frames, but you only get a stream of information continuously in time with microsecond temporal resolution. So one of the key advantages of event cameras is that, basically, you can actually record phenomena that actually would take expensive high-speed cameras to perceive. But the key difference with a standard camera is that an event camera works in differential mode. And because it works in differential mode, by basically capturing per-pixel intensity differences, it consumes very little power, and it also has no motion blur, because it doesn't accumulate photons over time.
So I would say that for robotics, what I- because you asked me how did I find out. So what I really, really saw, actually, that was very useful for robotics about event cameras were two particular things. First of all, the very high temporal resolution, because this can be very useful for safety, critical systems. And I'm thinking about drones, but also to avoid collisions in the automotive setting, because now we are also working in automotive settings as well. And also when you have to navigate in low-light environments, where using a standard camera with the high exposure times, you would actually be coping with a lot of motion blur that would actually cause a feature loss and other artifacts, like impossibility to detect objects and so on. So event cameras excel at this. No motion blur and very low latency. Another thing that could be also very interesting for especially lightweight robotics-and I'm thinking of micro drones-would be actually the fact that they consume also very little power. So little power, in fact, just to be on an event camera consumes one milliwatt, on average, because in fact, the power consumption depends on the dynamics of the scene. If nothing moves, then the power consumption is very negligible. If something moves, it is between one milliwatt or maximum 10 milliwatt.
Now, the interesting thing is that if you then couple event cameras with the spiking neuromorphic chips that also consume less than one milliwatt, you can actually mount them on a micro drones, and you can do amazing things, and we started working on it. The problem is that how do you train spiking networks? But that's another story. Other interesting things where I see potential applications of event cameras are also, for example- now, think about your keyframe features of the Skydio drones. And here what you are doing, guys, is that basically, you are flying the drones around, and then you're trying to send 3D positions and orientation of where you would like then [inaudible] to fly faster through. But the images have been captured while the drone is still. So basically, you move the drone to a certain position, you orient it in the direction where later you want it to fly, and then you record the position and orientation, and later, the drone will fly agilely through it. But that means that, basically, the drone should be able to relocalize fast with respect to this keyframe. Well, at some point, there are failure modes. We already know it. Failure modes. When the illumination goes down and there is motion blur, and this is actually something where I see, actually, the event camera could be beneficial. And then other things, of course [crosstalk]-
Ackerman: Do you agree with that, Adam?
Bry: Say again?
Ackerman: Do you agree, Adam?
Bry: I guess I'm- and this is why kind of I'm asking the question. I'm very curious about event cameras. When I have kind of the pragmatic hat on of trying to build these systems and make them as useful as possible, I see event cameras as quite complementary to traditional cameras. So it's hard for me to see a future where, for example, on our products, we would be only using event cameras. But I can certainly imagine a future where, if they were compelling from a size, weight, cost standpoint, we would have them as an additional sensing mode to get a lot of the benefits that Davide is talking about. And I don't know if that's a research direction that you guys are thinking about. And in a research context, I think it's very cool and interesting to see what can you do with just an event camera. I think that the most likely scenario to me is that they would become like a complementary sensor, and there's probably a lot of interesting things to be done of using standard cameras and event cameras side by side and getting the benefits of both, because I think that the context that you get from a conventional camera that's just giving you full static images of the scene, combined with an event camera could be quite interesting. You can imagine using the event camera to sharpen and get better fidelity out of the conventional camera, and you could use the event camera for faster response times, but it gives you less of a global picture than the conventional camera. So Davide's smiling. Maybe I'm- I'm sure he's thought about all these ideas as well.
Scaramuzza: Yeah. We have been working on that exact thing, combining event cameras with standard cameras, now for the past three years. So initially, when we started almost 10 years ago, of course, we only focused on event cameras alone, because it was intellectually very challenging. But the reality is that an event camera-let's not forget-it's a differential sensor. So it's only complementary with standard camera. You will never get the full absolute intensity from out of an event camera. We show that you can actually reproduce the grayscale intensity up to an unknown absolute intensity with very high fidelity, by the way, but it's only complementary to a standard camera, as you correctly said. So actually, you already mentioned everything we are working on and we have also already published. So for example, you mentioned unblurring blurry frames. This also has already been done, not by my group, but a group of Richard Hartley at the University of Canberra in Australia. And what we also showed in my group last year is that you can also generate super slow motion video by combining an event camera with a standard camera, by basically using the events in the blind time between two frames to interpolate and generate arbitrary frames at any arbitrary time. And so we show that we could actually upsample a low frame rate video by a factor of 50, and this with only consuming one-fortieth of the memory footprint. And this is interesting, because-
Bry: Do you think from- this is a curiosity question. From a hardware standpoint, I'm wondering if it'll go the next- go even a bit further, like if we'll just start to see image sensors that do both together. I mean, you could certainly imagine just putting the two pieces of silicon right next to each other, or- I don't know enough about image sensor design, but even at the pixel level, you could have pixel- like just superimposed on the same piece of silicon. You could have event pixels next to standard accumulation pixels and get both sets of data out of one sensor.
Scaramuzza: Exactly. So both things have been done. So-
Bry: [crosstalk].
Scaramuzza: -the latest one I described, we actually installed an event camera side by side with a very high-resolution standard camera. But there is already an event camera called DAVIS that outputs both frames and events between the frames. This has been available already since 2016, but at the very low resolution, and only last year it reached the VGA resolution. That's why we are combining-
Bry: That's like [crosstalk].
Scaramuzza: -an event camera with a high-resolution standard camera, because want to basically see what we could possibly do one day when these event cameras are also available [inaudible] resolution together with a standard camera overlaid on the same pixel array. But there is a good news, because you also asked me another question about cost of this camera. So the price, as you know very well, drops as soon as there is a mass product for it. The good news is that Samsung has now a product called SmartThings Vision Sensor that basically is conceived for indoor home monitoring, so to basically detect people falling at home, and this device automatically triggers an emergency call. So this device is using an event camera, and it costs 180, which is much less than the cost of an event camera when you buy it from these companies. It's around 3,000. So that's a very good news. Now, if there will be other bigger applications, we can expect that the price would go down a lot, below even $5. That's what these companies are openly saying. I mean, what I expect, honestly, is that it will follow what we experience with the time-of-flight cameras. I mean, the first time-of-flight cameras cost around $15,000, and then 15 years later, they were below $150. I'm thinking of the first Kinect tool that was time-of-flight and so on. And now we have them in all sorts of smartphones. So it all depends on the market.
Ackerman: Maybe one more question from each of you guys, if you've got one you've been saving for the end.
Scaramuzza: Okay. The very last question [inaudible]. Okay. I ask, Adam, and then you tell me if you want to answer or rather not. It's, of course, about defense. So the question I prepared, I told Evan. So I read in the news that Skydio donated 300K of equivalent of drones to Ukraine. So my question is, what are your views on military use or dual use of quadcopters, and what is the philosophy of Skydio regarding defense applications of drones? I don't know if you want to answer.
Bry: Yeah, that's a great question. I'm happy to answer that. So our mission, which we've talked about quite publicly, is to make the world more productive, creative, and safe with autonomous flight. And the position that we've taken, and which I feel very strongly about, is that working with the militaries of free democracies is very much in alignment and in support of that mission. So going back three or four years, we've been working with the US Army. We won the Army's short-range reconnaissance program, which was essentially a competition to select the official kind of soldier-carried quadcopter for the US Army. And the broader trend there, which I think is really interesting and in line with what we've seen in other technology categories, is basically the consumer and civilian technology just raced ahead of the traditional defense systems. The military has been using drones for decades, but their soldier-carried systems were these multi-hundred-thousand-dollar things that are quite clunky, quite difficult to use, not super capable. And our products and other products in the consumer world basically got to the point where they had comparable and, in many cases, superior capability at a fraction of the cost.
And I think- to the credit of the US military and other departments of defense and ministries of defense around the world, I think people realized that and decided that they were better off going with these kind of dual-use systems that were predominantly designed and scaled in civilian markets, but also had defense applicability. And that's what we've done as a company. So it's essentially our consumer civilian product that's extended and tweaked in a couple of ways, like the radios, some of the security protocols, to serve defense customers. And I'm super proud of the work that we're doing in Ukraine. So we've donated $300,000 worth of systems. At this point, we've sold way, way more than that, and we have hundreds of systems in Ukraine that are being used by Ukrainian defense forces, and I think that's good important work. The final piece of this that I'll say is we've also decided and we aren't doing and we won't put weapons on our drones. So we're not going to build actual munition systems, which I think is- I don't think there's anything ethically wrong with that. Ultimately, militaries need weapons systems, and those have an important role to play, but it's just not something that we want to do as a company, and is kind of out of step with the dual-use philosophy, which is really how we approach these things.
I have a question that I'm- it's aligned with some of what we've talked about, but I'm very interested in how you think about and focus the research in your lab, now that this stuff is becoming more and more commercialized. There's companies like us and others that are building real products based on a lot of the algorithms that have come out of academia. And in general, I think it's an incredibly exciting time where the pace of progress is accelerating, there's more and more interesting algorithms out there, and it seems like there's benefits flowing both ways between research labs and between these companies, but I'm very interested in how you're thinking about that these days.
Scaramuzza: Yes. It's a very interesting question. So first of all, I think of you also as a robotics company. And so what you are demonstrating is what [inaudible] of robotics in navigation and perception can do, and the fact that you can do it on a drone, it means you can also do it on other robots. And that actually is a call for us researchers, because it pushes us to think of new venues where we can actually contribute. Otherwise, it looks like everything has been done. And so what, for example, we have been working on in my lab is trying to- so towards the goal of achieving human-level performance, how do humans do navigate? They don't do ultimate control and geometric 3D reconstruction. We have a brain that does everything end to end, or at least with the [inaudible] subnetworks. So one thing that we have been playing with has been now deep learning for already now, yeah, six years. But in the last two years, we realized, actually, that you can do a lot with deep networks, and also, they have some advantages compared to the usual traditional autonomy architectures- architecture of autonomous robots. So what is the standard way to control robots, be it flying or ground? You have [inaudible] estimation. They have a perception. So basically, special AI, semantic understanding. Then you have localization, path planning, and control.
Now, all these modules are basically communicating with one another. Of course, you want them to communicate in a smart way, because you want to also try to plan trajectories that facilitate perception, so you have no motion blur while you navigate, and so on. But somehow, they are always conceived by humans. And so what we are trying to understand is whether you can actually replace some of these blocks or even all blocks and up to each point with deep networks, which begs the question, can you even train a policy end to end that takes as input some sort of sensory, like either images or even sensory obstructions, and outputs control commands of some sort of output abstraction, like [inaudible] or like waypoints? And what we found out is that, yes, this can be done. Of course, the problem is that for training these policies, you need a lot of data. And how do you generate this data? You can not fly drones in the real world. So we started working more and more in simulation. So now we are actually training all these things in simulation, even for forests. And thanks to the video game engines like Unity, now you can download a lot of these 3D environments and then deploy your algorithms there that train and teach a drone to fly in just a bunch of hours rather than flying and crashing drones in the real world, which is very costly as well. But the problem is that we need better simulators.
We need better simulators, and I'm not just thinking of for the realism. I think that one is actually somewhat solved. So I think we need the better physics like aerodynamic effects and other non-idealities. These are difficult to model. So we are also working on these kind of things. And then, of course, another big thing would be you would like to have a navigation policy that is able to abstract and generalize to different type of tasks, and possibly, at some point, even tell your drone or robot a high-level description of the task, and the drone or the robot would actually accomplish the task. That would be the dream. I think that the robotics community, we are moving towards that.
Bry: Yeah. I agree. I agree, and I'm excited about it.
Ackerman: We've been talking with Adam Bry from Skydio and Davide Scaramuzza from the University of Zurich about agile autonomous drones, and thanks again to our guests for joining us. For Chatbot and IEEE Spectrum, I'm Evan Ackerman.