A Roadmap for Regulating AI Programs
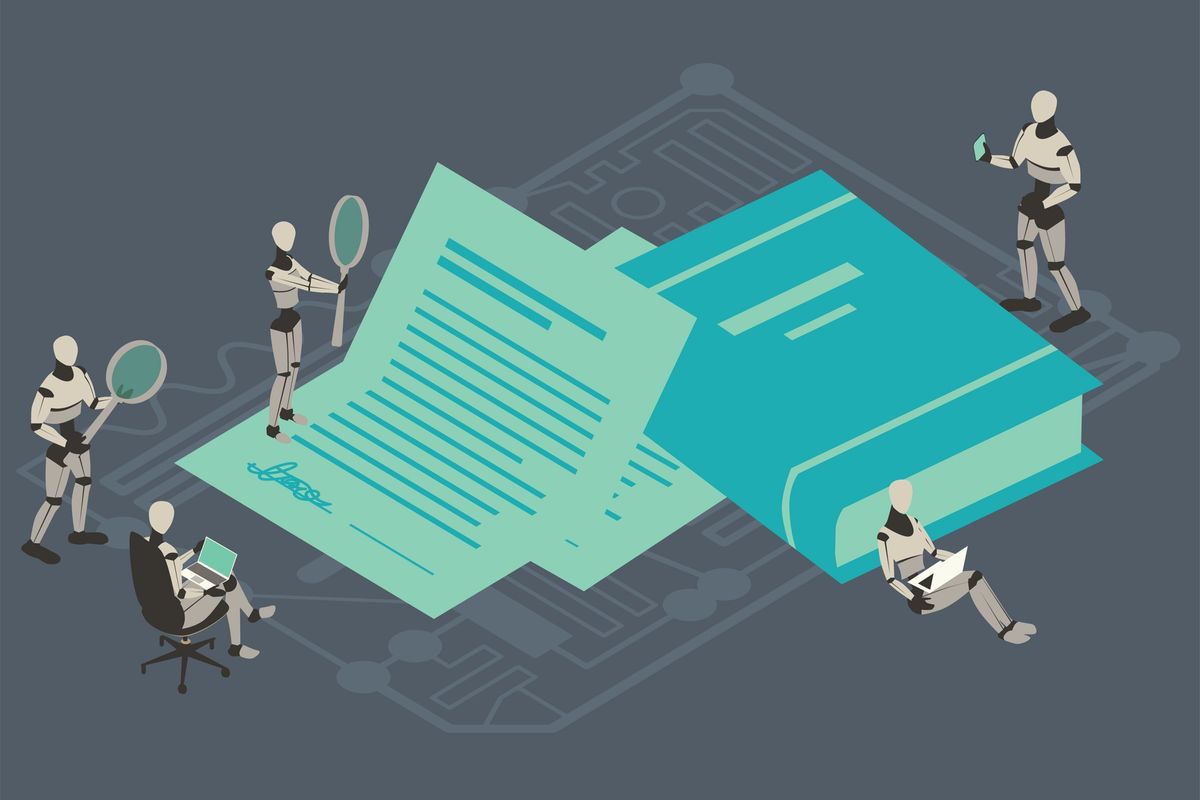
Globally, policymakers are debating governance approaches to regulate automated systems, especially in response to growing anxiety about unethical use of generative AI technologies such as ChatGPT and DALL-E. Legislators and regulators are understandably concerned with balancing the need to limit the most serious consequences of AI systems without stifling innovation with onerous government regulations. Fortunately, there is no need to start from scratch and reinvent the wheel.
As explained in the IEEE-USA article How Should We Regulate AI?," the IEEE 1012 Standard for System, Software, and Hardware Verification and Validation already offers a road map for focusing regulation and other risk management actions.
Introduced in 1988, IEEE 1012 has a long history of practical use in critical environments. The standard applies to all software and hardware systems including those based on emerging generative AI technologies. IEEE 1012 is used to verify and validate many critical systems including medical tools, the U.S. Department of Defense's weapons systems, and NASA's manned space vehicles.
In discussions of AI risk management and regulation, many approaches are being considered. Some are based on specific technologies or application areas, while others consider the size of the company or its user base. There are approaches that either include low-risk systems in the same category as high-risk systems or leave gaps where regulations would not apply. Thus, it is understandable why a growing number of proposals for government regulation of AI systems are creating confusion.
Determining risk levelsIEEE 1012 focuses risk management resources on the systems with the most risk, regardless of other factors. It does so by determining risk as a function of both the severity of consequences and their likelihood of occurring, and then it assigns the most intense levels of risk management to the highest-risk systems. The standard can distinguish, for example, between a facial recognition system used to unlock a cellphone (where the worst consequence might be relatively light) and a facial recognition system used to identify suspects in a criminal justice application (where the worst consequence could be severe).
IEEE 1012 presents a specific set of activities for the verification and validation (V&V) of any system, software, or hardware. The standard maps four levels of likelihood (reasonable, probable, occasional, infrequent) and the four levels of consequence (catastrophic, critical, marginal, negligible) to a set of four integrity levels (see Table 1). The intensity and depth of the activities varies based on how the system falls along a range of integrity levels (from 1 to 4). Systems at integrity level 1 have the lowest risks with the lightest V&V. Systems at integrity level 4 could have catastrophic consequences and warrant substantial risk management throughout the life of the system. Policymakers can follow a similar process to target regulatory requirements to AI applications with the most risk.
Table 1: IEEE 1012 Standard's Map of Integrity Levels Onto a Combination of Consequence and Likelihood LevelsLikelihood of occurrence of an operating state that contributes to the error (decreasing order of likelihood) | ||||
Error consequence | Reasonable | Probable | Occasional | Infrequent |
Catastrophic | 4 | 4 | 4 or 3 | 3 |
Critical | 4 | 4 or 3 | 3 | 2 or 1 |
Marginal | 3 | 3 or 2 | 2 or 1 | 1 |
Negligible | 2 | 2 or 1 | 1 | 1 |
As one might expect, the highest integrity level, 4, appears in the upper-left corner of the table, corresponding to high consequence and high likelihood. Similarly, the lowest integrity level, 1, appears in the lower-right corner. IEEE 1012 includes some overlaps between the integrity levels to allow for individual interpretations of acceptable risk, depending on the application. For example, the cell corresponding to occasional likelihood of catastrophic consequences can map onto integrity level 3 or 4.
Policymakers can customize any aspect of the matrix shown in Table 1. Most substantially, they could change the required actions assigned to each risk tier. IEEE 1012 focuses specifically on V&V activities.
Policymakers can and should consider including some of those for risk management purposes, but policymakers also have a much broader range of possible intervention alternatives available to them, including education; requirements for disclosure, documentation, and oversight; prohibitions; and penalties.
The standard offers both wise guidance and practical strategies for policymakers seeking to navigate confusing debates about how to regulate new AI systems."
When considering the activities to assign to each integrity level, one commonsense place to begin is by assigning actions to the highest integrity level where there is the most risk and then proceeding to reduce the intensity of those actions as appropriate for lower levels. Policymakers should ask themselves whether voluntary compliance with risk management best practices such as the NIST AI Risk Management Framework is sufficient for the highest risk systems. If not, they could specify a tier of required action for the highest risk systems, as identified by the consequence levels and probability levels discussed earlier. They can specify such requirements for the highest tier of systems without a concern that they will inadvertently introduce barriers for all AI systems, even low-risk internal systems.
That is a great way to balance concern for public welfare and management of severe risks with the desire not to stifle innovation.
A time-tested processIEEE 1012 recognizes that managing risk effectively means requiring action throughout the life cycle of the system, not simply focusing on the final operation of a deployed system. Similarly, policymakers need not be limited to placing requirements on the final deployment of a system. They can require actions throughout the entire process of considering, developing, and deploying a system.
IEEE 1012 also recognizes that independent review is crucial to the reliability and integrity of outcomes and the management of risk. When the developers of a system are the same people who evaluate its integrity and safety, they have difficulty thinking out of the box about problems that remain. They also have a vested interest in a positive outcome. A proven way to improve outcomes is to require independent review of risk management activities.
IEEE 1012 further tackles the question of what really constitutes independent review, defining three crucial aspects: technical independence, managerial independence, and financial independence.
IEEE 1012 is a time-tested, broadly accepted, and universally applicable process for ensuring that the right product is correctly built for its intended use. The standard offers both wise guidance and practical strategies for policymakers seeking to navigate confusing debates about how to regulate new AI systems. IEEE 1012 could be adopted as is for V&V of software systems, including the new systems based on emerging generative AI technologies. The standard also can serve as a high-level framework, allowing policymakers to modify the details of consequence levels, likelihood levels, integrity levels, and requirements to better suit their own regulatory intent.