Top AI Shops Fail Transparency Test
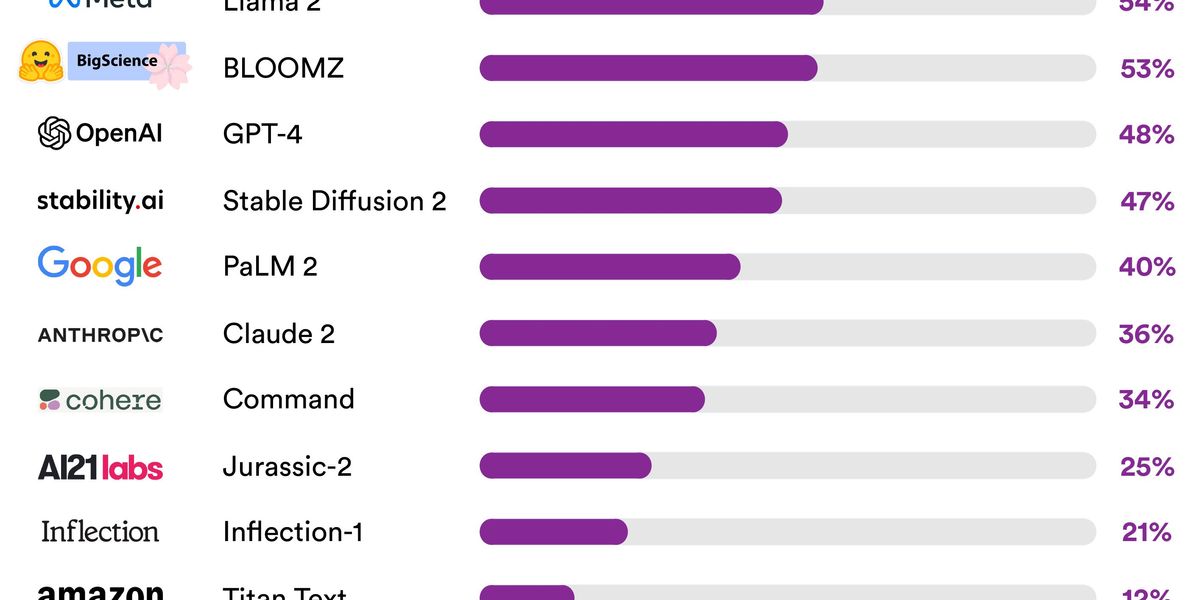
In July and September, 15 of the biggest AI companies signed on to the White House's voluntary commitments to manage the risks posed by AI. Among those commitments was a promise to be more transparent: to share information across the industry and with governments, civil society, and academia," and to publicly report their AI systems' capabilities and limitations. Which all sounds great in theory, but what does it mean in practice? What exactly is transparency when it comes to these AI companies' massive and powerful models?
Thanks to a report spearheaded by Stanford's Center for Research on Foundation Models (CRFM), we now have answers to those questions. The foundation models they're interested in are general-purpose creations like OpenAI's GPT-4 and Google's PaLM 2, which are trained on a huge amount of data and can be adapted for many different applications. The Foundation Model Transparency Index graded 10 of the biggest such models on 100 different metrics of transparency.
The highest total score goes to Meta's Llama 2, with 54 out of 100.
They didn't do so well. The highest total score goes to Meta's Llama 2, with 54 out of 100. In school, that'd be considered a failing grade. No major foundation model developer is close to providing adequate transparency," the researchers wrote in a blog post, revealing a fundamental lack of transparency in the AI industry."
Rishi Bommasani, a PhD candidate at Stanford's CRFM and one of the project leads, says the index is an effort to combat a troubling trend of the past few years. As the impact goes up, the transparency of these models and companies goes down," he says. Most notably, when OpenAI versioned-up from GPT-3 to GPT-4, the company wrote that it had made the decision to withhold all information about architecture (including model size), hardware, training compute, dataset construction, [and] training method."
The 100 metrics of transparency (listed in full in the blog post) include upstream factors relating to training, information about the model's properties and function, and downstream factors regarding the model's distribution and use. It is not sufficient, as many governments have asked, for an organization to be transparent when it releases the model," says Kevin Klyman, a research assistant at Stanford's CRFM and a coauthor of the report. It also has to be transparent about the resources that go into that model, and the evaluations of the capabilities of that model, and what happens after the release."
To grade the models on the 100 indicators, the researchers searched the publicly available data, giving the models a 1 or 0 on each indicator according to predetermined thresholds. Then they followed up with the 10 companies to see if they wanted to contest any of the scores. In a few cases, there was some info we had missed," says Bommasani.
Spectrum contacted representatives from a range of companies featured in this index; none of them had replied to requests for comment as of our deadline.
Labor in AI is a habitually opaque topic. And here it's very opaque, even beyond the norms we've seen in other areas."
-Rishi Bommasani, Stanford
The provenance of training data for foundation models has become a hot topic, with several lawsuits alleging that AI companies illegally included authors' copyrighted material in their training data sets. And perhaps unsurprisingly, the transparency index showed that most companies have not been forthcoming about their data. The model Bloomz from the developer Hugging Face got the highest score in this particular category, with 60 percent; none of the other models scored above 40 percent, and several got a zero.
A heatmap shows how the 10 models did on categories ranging from data to impact. Stanford Center for Research on Foundation Models
Companies were also mostly mum on the topic of labor, which is relevant because models require human workers to refine their models. For example, OpenAI uses a process called reinforcement learning with human feedback to teach models like GPT-4 which responses are most appropriate and acceptable to humans. But most developers don't make public the information about who those human workers are and what wages they're paid, and there's concern that this labor is being outsourced to low-wage workers in places like Kenya. Labor in AI is a habitually opaque topic," says Bommasani, and here it's very opaque, even beyond the norms we've seen in other areas."
Hugging Face is one of three developers in the index that the Stanford researchers considered open," meaning that the models' weights are broadly downloadable. The three open models (Llama 2 from Meta, Hugging Face's Bloomz, and Stable Diffusion from Stability AI) are currently leading the way in transparency, scoring greater or equal to the best closed model.
While those open models scored transparency points, not everyone believes they're the most responsible actors in the arena. There's a great deal of controversy right now about whether or not such powerful models should be open sourced and thus potentially available to bad actors; just a few weeks ago, protesters descended on Meta's San Francisco office to decry the irreversible proliferation" of potentially unsafe technology.
Bommasani and Klyman say the Stanford group is committed to keeping up with the index, and are planning to update it at least once a year. The team hopes that policymakers around the world will turn to the index as they craft legislation regarding AI, as there are regulatory efforts ongoing in many countries. If companies do better at transparency in the 100 different areas highlighted by the index, they say, lawmakers will have better insights into which areas require intervention. If there's pervasive opacity on labor and downstream impacts," says Bommasani, this gives legislators some clarity that maybe they should consider these things."
It's important to remember that even if a model had gotten a high transparency score in the current index, that wouldn't necessarily mean it was a paragon of AI virtue. If a company disclosed that a model was trained on copyrighted material and refined by workers paid less than minimum wage, it would still earn points for transparency about data and labor.
We're trying to surface the facts" as a first step, says Bommasani. Once you have transparency, there's much more work to be done."