The Biggest Questions: How did life begin?
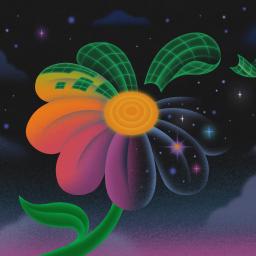
How life begins is one of the biggest and hardest questions in science. All we know is that something happened on Earth more than 3.5 billion years ago, and it may well have occurred on many other worlds in the universe as well.
But we don't know what does the trick. Somehow a soup of nonliving chemicals like water and methane must combine and self-organize, growing ever more complex and coordinated, until eventually it gives rise to a living cell.
One of the biggest difficulties is the sheer complexity of the problem: even the simplest known bacteria have well over 100 genes and contain hundreds of kinds of molecules, all furiously interacting in a microscopic dance. The environment on the primordial Earth must also have been complicated: huge numbers of different chemicals, from metals and minerals to water and gases, all being blasted around by winds and volcanic eruptions.
The experimental parameter space is almost infinite," says Wilhelm Huck, a chemist at Radboud University in Nijmegen, the Netherlands.
Now, a few researchers are trying a new approach: harnessing artificial intelligence to zero in on the winning conditions. Specifically, several groups have started using machine-learning tools that can identify patterns in data sets too huge and messy for the human brain to comprehend.
The hope is that these tools will help researchers achieve in years what would otherwise take decades. By pointing the way to the fastest and sturdiest processes for generating complexity, they could help us devise a universal theory of the origins of life-one that applies not just on Earth but on any other world.
It's early days-but there have already been some significant advances.
Making life from scratchThe origins-of-life problem is at least partly about chemistry: what mix of chemicals, under what conditions, is required for life to form? Chemistry is going to answer this question-one of the deepest questions humanity has," says Leroy Lee" Cronin, a chemist at the University of Glasgow in the UK.
The study of the origins of life was kick-started by an experiment published in 1953. Stanley Miller, a graduate student supervised by the chemist Harold Urey, mixed water and three gases in glass flasks, which were heated and subjected to electric shocks that mimicked the lightning he assumed regularly struck the young Earth. Within days, this setup had produced glycine, the simplest amino acid and one of the building blocks of proteins.
While the Miller experiment did not produce life or anything close to it, it became iconic because it was relatively unsupervised: Miller just set it up and let it run. This was meant to mimic conditions on the young Earth, where there were no synthetic chemists to guide chemical reactions to the correct" end. However, the purported realism of the experiment was also a problem: it produced so many chemicals that identifying them all and understanding how they formed was almost impossible.
Many subsequent experiments in prebiotic" chemistry have been more carefully controlled. They have succeeded in producing many more amino acids, sugars, and other chemicals of life. However, it's not clear that such meticulously curated reactions would take place without human intervention-and so they may tell us nothing about the primordial Earth. What researchers want is a way to get back to the Miller experiment, finding better ways to explore what happens in uncontrolled complex mixtures.
That's where machine learning can come in. The technology has already been applied to existing problems in biology: notably, Google DeepMind's system AlphaFold has successfully predicted the three-dimensional folded shapes of thousands of proteins. To make this possible, its creators first trained AlphaFold on the known structures of many proteins. Once it had learned the patterns, it was able to predict, with high accuracy, the structures of other proteins that had not yet been characterized.
Betul Kacar at the University of Wisconsin-Madison and her colleagues did something similar in a study published in 2022. They were trying to reconstruct the evolutionary history of proteins called rhodopsins, which bacteria use to absorb energy from light. In particular, they wanted to know what kinds of light the earliest rhodopsins absorbed, as this would indicate what sort of environment they evolved in.
By comparing the genes that code for rhodopsin in distantly related microbes, they were able to estimate the sequences of the oldest rhodopsin genes-genes that no longer exist. Furthermore, they concluded that these early rhodopsin proteins were tuned to specific frequencies of light. They determined this by using a machine-learning technique developed by another group, which could predict the light sensitivity of present-day rhodopsins. Kacar's team used machine learning to show that the primordial rhodopsins were most sensitive to green light. This suggested that the microbes they were part of lived a little below the surface of a body of water, where other frequencies of light were blocked by the water. This fits with other lines of evidence about where the first life originated.
Exploring the messWhat about those complex mixtures of chemicals? One approach to understanding them was pioneered by the synthetic chemist Bartosz Grzybowski at the Institute for Basic Science in Ulsan, Republic of Korea, in a study published in 2020.
The team compiled data on prebiotic chemistry from dozens of papers published since the Miller experiment in 1953, each of which demonstrated a small number of reactions. They combined them into a single database, creating a network of reactions. Then they wrote a computer program to predict new reactions, based on which kinds of interactions can take place between different kinds of chemicals.
Starting from six simple starting materials, including water and ammonia, they showed it was possible to create tens of thousands of chemicals under mild conditions-including many of those found in live organisms. Crucially, the software predicted reactions that have never been observed, several of which the researchers then performed. At a stroke, they had identified a host of new chemical reactions that could have been important in the formation of the first life.
The study would have been impossible without the software. A human is not going to map a network of tens or thousands or millions of connections," says Grzybowski. However, he emphasizes that the software was coded by chemists and obeys explicit rules. I wouldn't even call our stuff AI," he says, but rather a hybrid system."
Despite its correct predictions, Grzybowski's reaction network is still a little theoretical. We also need to know how fast each of the reactions goes, and whether the by-products from earlier reactions will interfere with later ones. Huck, the chemist at Radboud University in the Netherlands, and his colleagues have begun tackling this with help from machine learning.
In a study published in 2022, Huck's team performed the formose reaction, which creates sugars from simple carbon-based molecules. Given that a sugar called deoxyribose is used to make DNA, making sugars is a crucial early step in the origins of life. The formose reaction does this, but there's a catch. It tends to undergo a combinatorial explosion," says Huck: it produces dozens or hundreds of products, which vary enormously depending on the exact conditions.
Huck's team carried out the reaction in small flow chambers to keep it under control. They varied a number of conditions, including the temperature and the availability of different chemicals; stopped it once it had made a few dozen chemicals; and analyzed the mixture.
Environmental conditions like temperature determine what products are formed in the reaction, says Huck. However, it's not obvious how or why: tiny changes in conditions sometimes have little effect, but sometimes they lead to drastically different outcomes. That's where machine learning came in: after some training, the software was able to predict what the reaction would spit out. This takes us a step closer to understanding the conditions for making sugars on the primordial Earth.
Determining the environmental conditions and other parameters that prevailed at the time is one of the biggest problems for origins-of-life research, says Wentao Ma, a computer modeler at Wuhan University in China. Techniques like machine learning will help narrow it down. In a 2021 study, Ma and his colleagues simulated a mixture of nucleic acids. Using machine learning, they were able to find the optimal conditions for creating nucleic acids that could speed up the formation of their own building blocks-the kind of virtuous circle on which life depends.
Finally, machine learning can also help create high-fidelity simulations of the precise mechanisms by which chemical reactions happen-which is crucial for predicting when they will and won't work. Key tools for this are computer models that simulate all the atoms in a mixture as they bounce around and interact with each other. When performing the simulation, we can have access to the microscopic behavior of the system," says Timothee Devergne, a modeler at Sorbonne University in Paris.
However, these atomistic" simulations quickly become incredibly time consuming. Every single interaction between atoms requires solving complex equations, so it has been very challenging to simulate the complex mixtures that existed on the primordial Earth. Consequently, experiments in prebiotic chemistry have been something of a black box: we can see what's being spat out, but exactly what happened is mysterious.
Devergne is using machine learning to crack this problem. Back in 2007, researchers at ETH Zurich in Switzerland developed a neural network that could learn the most likely solutions to the necessary equations. This sped up the calculations by several orders of magnitude. Devergne and his colleagues are now applying this method to prebiotic chemistry. As a proof of principle, in a study published in 2022 they used machine learning to simulate the reactions that made glycine in Miller's experiment-something Devergne's supervisor had previously simulated without machine learning. The neural network reduced the computation time by a factor of 10 to 50. Similar results from another group were released as a preprint in 2022.
What's the use?Everyone contacted for this story agreed that the use of machine learning and other AI tools in research on the origins of life is at a very early stage. Some are wary of over-hyping the approach.
It can't tell us new stuff, because it knows what it knows," says Valentina Erastova, a computational scientist at the University of Edinburgh. Machine-learning tools can only make accurate predictions after being fed enormous amounts of high-quality data, she says: It can show you trends and it can show you links, but the links it will show are also completely biased by how you train it."
What is clear is that AI-type tools can speed up what would otherwise be drudgery. For example, in 2018 Cronin's team described a robot that can perform chemical experiments and analyses faster than humans. It used machine learning to assess the progress of reactions in real time, and to predict which mixtures would and would not react. Cronin has already spent years digitizing chemistry: he plans to use these systems to conduct experiments in prebiotic chemistry and discover pathways to the formation of life. In analogy to AlphaFold, he says he wants to make AlphaSoup."
The power of machine learning is that it can see patterns in huge data sets when humans can't. You pick up patterns in complex mixtures and pinpoint processes taking place that you can't spot yourself," says Huck. It's such a high-dimensional space that the patterns elude you."
The hope is that these methods will enable researchers to finally understand what is happening in complex interacting mixtures like those found on the primordial Earth. This [technology] allows us to study way bigger systems than before," says Devergne.
There is one final question. Suppose one of the experiments succeeds and a biochemist manages to make a simple form of life in the lab-or suppose the Perseverance rover on Mars discovers an extraterrestrial microbe. How will we know if what we're looking at is truly alive?
This is an old problem in geochemistry," says Jim Cleaves, a geochemist at Howard University in Washington, DC. How do you say that something is living or not living?"
For Cronin, the answer is assembly theory." He and his colleagues argue that life's distinguishing feature is that it produces large numbers of highly complex objects. They define an object's complexity by the number of steps required to make it. In a 2021 study, they presented evidence that they could distinguish between samples produced by life and samples produced without it, based on the measured complexity of the molecules. Machine learning was used to speed up the analyses.
In a study published in September, Cleaves and his colleagues used machine learning more directly. They trained a neural network on a wide range of substances, including basmati rice, coal, and shale. Afterwards it could identify biological and nonbiological samples with 90% accuracy. The AI used a subtly different metric from Cronin's: it focuses on the overall mix of chemical components within a sample, Cleaves says, rather than the complexity of individual components. They're complementary ideas," he says.
Cleaves says methods like these could be applied to the data that comes back from probes like NASA's Mars rovers. For example, the Curiosity rover has an instrument called Sample Analysis at Mars (SAM) that performs chemical analyses similar to those his team used. With relatively minor modifications, he says, you could do it now."
Meanwhile researchers like Cronin and Huck are plowing ahead with their studies of primordial biochemistry. I think we will be able to use machine-learning techniques like AlphaFold, but we're going to have to retrain them on the chemical soup," says Cronin. We need to make AlphaSoup. If we can make AlphaSoup, we're going to be away to the races."
Michael Marshall is a freelance writer based in the UK. He mostly covers life sciences, health and the environment. His first book The Genesis Quest is about the origins of life and is out now.