This new system can teach a robot a simple household task within 20 minutes
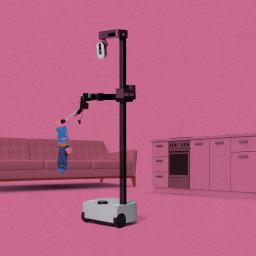
A new system that teaches robots a domestic task in around 20 minutes could help the field of robotics overcome one of its biggest challenges: a lack of training data.
The open-source system, called Dobb-E, was trained using data collected from real homes. It can help to teach a robot how to open an air fryer, close a door, or straighten a cushion, among other tasks.
While other types of AI, such as large language models, are trained on huge repositories of data scraped from the internet, the same can't be done with robots, because the data needs to be physically collected. This makes it a lot harder to build and scale training databases.
Similarly, while it's relatively easy to train robots to execute tasks inside a laboratory, these conditions don't necessarily translate to the messy unpredictability of a real home.
To combat these problems, the team came up with a simple, easily replicable way to collect the data needed to train Dobb-E-usingan iPhone attached to a reacher-grabber stick, the kind typically used to pick up trash. Then they set the iPhone to record videos of what was happening.
Volunteers in 22 homes in New York completed certain tasks using the stick, including opening and closing doors and drawers, turning lights on and off, and placing tissues in the trash. The iPhones' lidar systems, motion sensors, and gyroscopes were used to record data on movement, depth, and rotation-important information when it comes to training a robot to replicate the actions on its own.
After they'd collected just 13 hours' worth of recordings in total, the team used the data to train an AI model to instruct a robot in how to carry out the actions. The model used self-supervised learning techniques, which teach neural networks to spot patterns in data sets by themselves, without being guided by labeled examples.
The next step involved testing how reliably a commercially available robot called Stretch, which consists of a wheeled unit, a tall pole, and a retractable arm, was able to use the AI system to execute the tasks. An iPhone held in a 3D-printed mount was attached to Stretch's arm to replicate the setup on the stick.
The researchers tested the robot in 10 homes in New York over 30 days, and it completed 109 household tasks with an overall success rate of 81%. Each task typically took Dobb-E around 20 minutes to learn: five minutes of demonstration from a human using the stick and attached iPhone, followed by 15 minutes of fine-tuning, when the system compared its previous training with the new demonstration.
Once the fine-tuning was complete, the robot was able to complete simple tasks like pouring from a cup, opening blinds and shower curtains, or pulling board-game boxes from a shelf. It could also perform multiple actions in quick succession, such as placing a can in a recycling bag and then lifting the bag.
However, not every task was successful. The system was confused by reflective surfaces like mirrors. Also, because the robot's center of gravity is low, tasks that require pulling something heavy at height, like opening fridge doors, proved too risky to attempt.
The research represents tangible progress for the home robotics field, says Charlie C. Kemp, cofounder of the robotics firm Hello Robot and a former associate professor at Georgia Tech. Although the Dobb-E team used Hello Robot's research robot, Kemp was not involved in the project.
The future of home robots is really coming. It's not just some crazy dream anymore," he says. Scaling up data has always been a challenge in robotics, and this is a very creative, clever approach to that problem."
To date, Roomba and other robotic vacuum cleaners are the only real commercial home robot successes, says Jiajun Wu, an assistant professor of computer science at Stanford University who was not involved in the research. Their job is easier because Roombas don't interact with objects-in fact, their aim is to avoid them. It's much more challenging to develop home robots capable of doing a wider range of tasks, which is what this research could help advance.
The NYU research team has made all elements of the project open source, and they're hoping others will download the code and help expand the range of tasks that robots running Dobb-E will be able to achieve.
Our hope is that when we get more and more data, at some point when Dobb-E sees a new home, you don't have to show it more examples," says Lerrel Pinto, a computer science researcher at New York University who worked on the project.
We want to get to the point when we don't have to teach the robot new tasks, because it already knows all the tasks in most houses," he says.