Four trends that changed AI in 2023
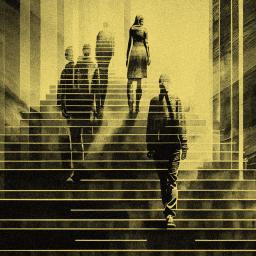
This story originally appeared in The Algorithm, our weekly newsletter on AI. To get stories like this in your inbox first, sign up here.
This has been one of the craziest years in AI in a long time: endless product launches, boardroom coups, intense policy debates about AI doom, and a race to find the next big thing. But we've also seen concrete tools and policies aimed at getting the AI sector to behave more responsibly and hold powerful players accountable. That gives me a lot of hope for the future of AI.
Here's what 2023 taught me:
1. Generative AI left the lab with a vengeance, but it's not clear where it will go nextThe year started with Big Techgoing all inon generative AI. The runaway success ofOpenAI's ChatGPTprompted every major tech company to release its own version. This year might go down in history as the year we saw the most AI launches: Meta'sLLaMA 2, Google'sBardchatbot andGemini, Baidu'sErnie Bot, OpenAI'sGPT-4, and a handful of other models, including one from a French open-source challenger, Mistral.
But despite the initial hype, we haven't seen any AI applications become an overnight success. Microsoft and Google pitchedpowerful AI-powered search, but it turned out to be more of a dud than a killer app. The fundamental flaws in language models, such as the fact that they frequentlymake stuff up, led to some embarrassing (and, let's be honest, hilarious) gaffes. Microsoft's Bing would frequently reply to people's questions withconspiracy theories, andsuggestedthat a New York Times reporter leave his wife. Google's Bard generated factually incorrect answers for its marketing campaign, which wiped $100 billion off the company's share price.
There is now a frenetic hunt for a popular AI product that everyone will want to adopt. Both OpenAI and Google are experimenting with allowing companies and developers to create customized AI chatbots and letting people build their own applications using AI-no coding skills needed. Perhaps generative AI will end up embedded inboringbut useful tools to help us boost ourproductivity at work. It might take the form ofAI assistants-maybe withvoice capabilities-andcoding support. Next year will be crucial in determining the real value of generative AI.
2. We learned a lot about how language models actually work, but we still know very littleEven though tech companies are rolling out large language models into products at a frenetic pace, there is stilla lot we don't know about how they work. They make stuff up and have severegender and ethnicbiases. This year we also found out that different language models generate texts with differentpolitical biases, and that theymake great toolsfor hacking people's private information. Text-to-image models can beprompted to spit out copyrighted images and pictures of real people, and they can easily be tricked intogenerating disturbing images. It's been great to see so much research into the flaws of these models, because this could take us a step closer to understanding why they behave the way they do, and ultimately fix them.
Generative models can be very unpredictable, and this year there were lots of attempts to try to make them behave as their creators want them to. OpenAI shared that it uses a technique calledreinforcement learning from human feedback, which uses feedback from users to help guide ChatGPT to more desirable answers. Astudy from the AI lab Anthropicshowed how simple natural-language instructions can steer large language models to make their results less toxic. But sadly, a lot of these attempts end up being quick fixes rather than permanent ones. Then there are misguided approaches like banning seemingly innocuous words such as placenta" from image-generating AI systems to avoid producing gore. Tech companies come up with workarounds like these because they don't know why models generate the content they do.
We also got a better sense of AI's true carbon footprint. Generating an image using a powerful AI modeltakes as much energy as fully charging your smartphone, researchers at the AI startup Hugging Face and Carnegie Mellon University found. Until now, the exact amount of energy generative AI uses has been a missing piece of the puzzle. More research into this could help us shift the way we use AI to be more sustainable.
3. AI doomerism went mainstreamChatter about the possibility that AI poses an existential risk to humans became familiar this year. Hundreds of scientists, business leaders, and policymakers have spoken up, from deep-learning pioneersGeoffrey Hintonand Yoshua Bengio to the CEOs of top AI firms, such as Sam Altman and Demis Hassabis, to the California congressman Ted Lieu and the former president of Estonia Kersti Kaljulaid.
Existential risk has become one of thebiggest memes in AI. The hypothesis is that one day we will build an AI that isfar smarter than humans, and this could lead to grave consequences. It's an ideology championed by many in Silicon Valley, includingIlya Sutskever, OpenAI's chief scientist, who played a pivotal role inousting OpenAI CEO Sam Altman(and then reinstating him a few days later).
But not everyone agrees with this idea.Meta's AI leadersYann LeCun and Joelle Pineau have said that these fears are ridiculous" and the conversation about AI risks has become unhinged." Many other power players in AI, such as researcherJoy Buolamwini, say that focusing on hypothetical risks distracts from the very real harms AI is causing today.
Nevertheless, the increased attention on the technology's potential to cause extreme harm has prompted many important conversations about AI policy and animated lawmakers all over the world to take action.
4. The days of the AI Wild West are overThanks to ChatGPT, everyone from the US Senate to the G7 wastalkingabout AI policy and regulation this year. In early December, European lawmakers wrapped up a busy policy year when they agreed on theAI Act, which will introduce binding rules and standards on how to develop the riskiest AI more responsibly. It will also ban certain unacceptable" applications of AI, such as police use of facial recognition in public places.
The White House, meanwhile, introduced anexecutive order on AI, plus voluntary commitments from leading AI companies. Its efforts aimed to bring more transparency and standards for AI and gave a lot of freedom to agencies to adapt AI rules to fit their sectors.
One concrete policy proposal that got a lot of attention was watermarks-invisible signals in text and images that can be detected by computers, in order to flag AI-generated content. These could be used to track plagiarism or help fight disinformation, and this year we saw research that succeeded in applying them to AI-generatedtextandimages.
It wasn't just lawmakers that were busy, but lawyers too. We saw arecord number of lawsuits, as artists and writers argued that AI companies hadscraped their intellectual propertywithout their consent and with no compensation. In an exciting counter-offensive, researchers at the University of Chicago developedNightshade, a new data-poisoning tool that lets artists fight back against generative AI by messing up training data in ways that could cause serious damage to image-generating AI models. There is a resistance brewing, and I expect more grassroots efforts to shift tech's power balance next year.
Now we know what OpenAI's superalignment team has been up to
OpenAI has announced the first results from its superalignment team, its in-house initiative dedicated to preventing a superintelligence-a hypothetical future AI that can outsmart humans-from going rogue. The team is led by chief scientistIlya Sutskever, who was part of the group that just last month fired OpenAI's CEO, Sam Altman, only to reinstate him a few days later.
Business as usual:Unlike many of the company's announcements, this heralds no big breakthrough. In a low-key research paper, the team describes a technique that lets a less powerful large language model supervise a more powerful one-and suggests that this might be a small step toward figuring out how humans might supervise superhuman machines.Read more from Will Douglas Heaven.
Bits and BytesGoogle DeepMind used a large language model to solve an unsolvable math problem
In a paper published in Nature, the company says it is the first time a large language model has been used to discover a solution to a long-standing scientific puzzle-producing verifiable and valuable new information that did not previously exist.(MIT Technology Review)
This new system can teach a robot a simple household task within 20 minutes
A new open-source system, called Dobb-E, was trained using data collected from real homes. It can help to teach a robot how to open an air fryer, close a door, or straighten a cushion, among other tasks. It could also help the field of robotics overcome one of its biggest challenges: a lack of training data.(MIT Technology Review)
ChatGPT is turning the internet into plumbing
German media giant Axel Springer, which owns Politico and Business Insider, announced a partnership with OpenAI, in which the tech company will be able to use its news articles as training data and the news organizations will be able to use ChatGPT to do summaries of news. This column has a clever point: tech companies are increasingly becoming gatekeepers for online content, and journalism is just plumbing for a digital faucet." (The Atlantic)
Meet the former French official pushing for looser AI rules after joining startup Mistral
A profile of Mistral AI cofounder Cedric O, who used to be France's digital minister. Before joining France's AI unicorn, he was a vocal proponent of strict laws for tech, but he lobbied hard against rules in the AI Act that would have restricted Mistral's models. He was successful: the company's models don't meet the computing threshold set by the law, and its open-source models are also exempt from transparency obligations. (Bloomberg)