Outperforming competitors as a data-driven organization
In 2006, British mathematician Clive Humby said, data is the new oil." While the phrase is almost a cliche, the advent ofgenerative AIis breathing new life into this idea. A global study on theFuture of Enterprise Data & AI by WNS Triange and Corinium Intelligence shows 76% of C-suite leaders and decision-makers are planning or implementing generative AI projects.
Harnessing the potential of data through AI is essential in today's business environment. A McKinseyreport says data-driven organizations demonstrate EBITDA increases of up to 25%. AI-driven data strategy can boost growth and realize untapped potential by increasing alignment with business objectives, breaking down silos, prioritizing data governance, democratizing data, and incorporating domain expertise.
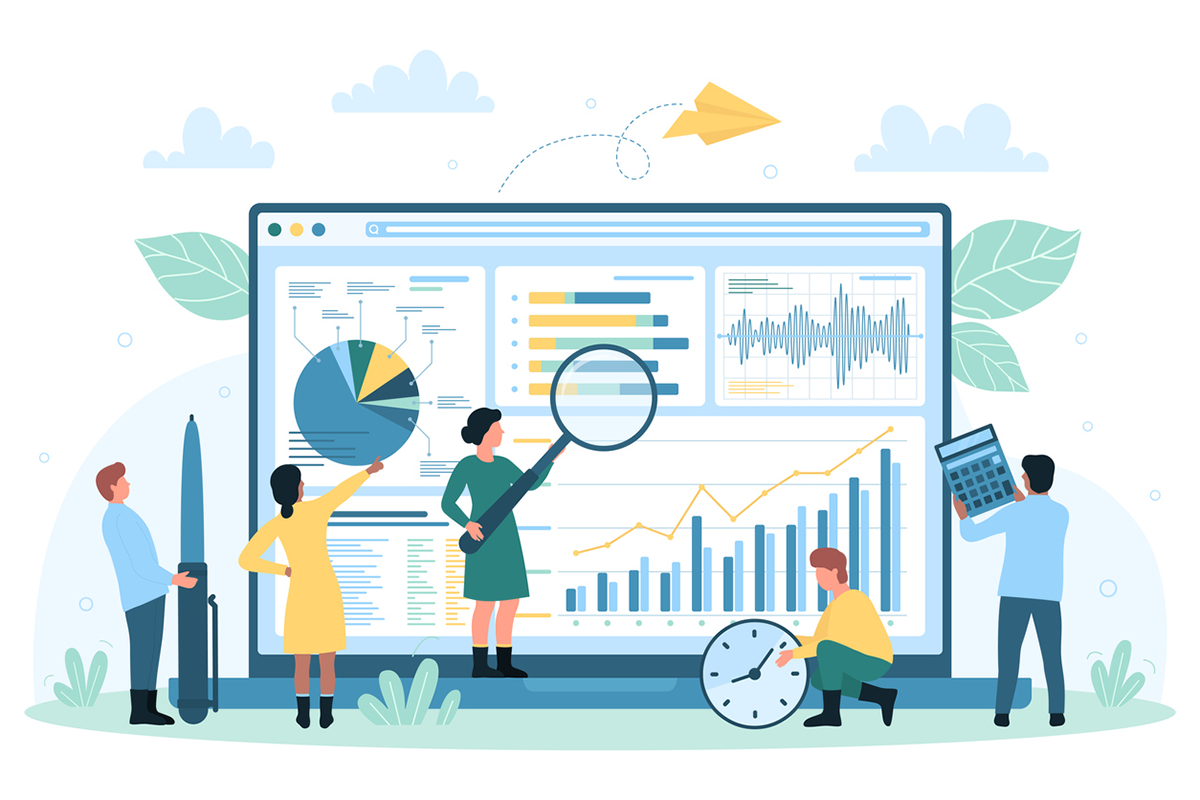
Companies need to have the necessary data foundations, data ecosystems, and data culture to embrace an AI-driven operating model,"says Akhilesh Ayer, executive vice president and global head of AI, analytics, data, and research practice atWNS Triange, a unit of business process management company WNS Global Services.
A unified data ecosystemEmbracing an AI-driven operating model requires companies to makedatathe foundation of their business. Business leaders need to ensure every decision-making process is data-driven, so that individual judgment-based decisions are minimized," says Ayer. This makes real-time data collection essential.For example, if I'm doing fraud analytics for a bank, I need real-time data of a transaction," explains Ayer. Therefore, the technology team will have to enable real-time data collection for that to happen."
Real-time data is just one element of a unified data ecosystem. Ayer says an all-round approach is necessary. Companies need clear direction from senior management; well-defined control of data assets; cultural and behavioral changes; and the ability to identify the right business use cases and assess the impact they'll create.
Aligning business goals with data initiativesAnAI-driven data strategywill only boost competitiveness if it underpins primary business goals.Ayer says companies must determine their business goals before deciding what to do with data.
One way to start, Ayer explains, is a data-and-AI maturity audit or a planning exercise to determine whether an enterprise needs a data product roadmap.This can determine if a business needs to re-architect the way data is organized or implement a data modernization initiative," hesays.
The demand for personalization, convenience, and ease in the customer experience is a central and differentiating factor. How businesses use customer data is particularly important for maintaining a competitive advantage, and can fundamentally transform business operations.
Ayer citesWNS Triange's work with a retail clientas an example of how evolving customer expectations drive businesses to make better use of data. The retailer wanted greater value from multiple data assets to improve customer experience. In a data triangulation exercise while modernizing the company's data with cloud and AI, WNS Triange created a unified data store with personalization models to increase return on investment and reduce marketing spend. Greater internal alignment of data is just one way companies can directly benefit and offer an improved customer experience," says Ayer.
Weeding out silosRegardless of an organization's data ambitions, few manage to thrive without clear and effective communication. Modern data practices have process flows or application programming interfaces that enable reliable, consistent communication between departments to ensure secure and seamless data-sharing, says Ayer.
This is essential tobreaking down silosand maintaining buy-in.When companies encourage business units to adopt better data practices through greater collaboration with other departments and data ecosystems, every decision-making process becomes automatically data-driven," explainsAyer.
WNS Triange helped a well-established insurer remove departmental silos and establish better communication channels. Silos were entrenched. The company had multiple business lines in different locations and legacy data ecosystems. WNS Triange brought them together and secured buy-in for a common data ecosystem. The silos are gone and there's the ability to cross leverage," saysAyer. As a group, they decide what prioritization they should take; which data program they need to pick first; and which businesses should be automated and modernized."
Data ownership beyond ITRemoving silos is not always straightforward. In many organizations, data sits in different departments. To improve decision-making, Ayer says, businesses can unite underlying data from various departments and broaden data ownership. One way to do this is to integrate the underlying data and treat this data as a product.
While IT can lay out the system architecture and design, primary data ownership shifts to business users. They understand what data is needed and how to use it,says Ayer.This means you give the ownership and power of insight-generation to the users," he says.
This data democratization enables employees to adopt data processes and workflows that cultivate a healthy data culture. Ayer says companies are investing in trainings in this area. We've even helped a few companies design the necessary training programs that they need to invest in," he says.
Tools for data decentralizationData mesh anddata fabric, powered by AI, empower businesses to decentralize data ownership, nurture the data-as-a-product concept, and create a more agile business.
For organizations adopting a data fabric model, it's crucial to include a data ingestion framework to manage new data sources. Dynamic data integration must be enabled because it's new data with a new set of variables," says Ayer. How it integrates with an existing data lake or warehouse is something that companies should consider."
Ayer cites WNS Triange's collaboration with a travel client as an example of improving data control. The client had various business lines in different countries, meaning controlling data centrally was difficult and ineffective. WNS Triange deployed a data mesh and data fabric ecosystem that allowed for federated governance controls. This boosted data integration and automation, enabling the organization to become more data-centric and efficient.
A governance structure for allGovernance controls can be federated, which means that while central IT designs the overall governance protocols, you hand over some of the governance controls to different business units, such as data-sharing, security, and privacy, making data deployment more seamless and effective," says Ayer.
AI-powered data workflow automation canadd precision and improve downstream analytics.For example, Ayer says, in screening insurance claims for fraud, when an insurer's data ecosystem and workflows are fully automated, instantaneous AI-driven fraud assessments are possible.
The ability to process a fresh claim, bring it into a central data ecosystem, match the policyholder's information with the claim's data, and make sure that the claim-related information passes through a model to give a recommendation, and then push back that recommendation into the company's workflow is the phenomenal experience of improving downstream analytics,"Ayer says.
Data-driven organizations of the futureA well-crafted data strategy aligned with clear business objectives can seamlessly integrate AI tools and technologies into organizational infrastructure. This helps ensure competitive advantage in the digital age.
To benefit from any data strategy, organizations must continuously overcome barriers such as legacy data platforms, slow adoption, and cultural resistance. It's extremely critical that employees embrace it for the betterment of themselves, customers, and other stakeholders," Ayer points out.Organizations can stay data-driven by aligning data strategy with business goals, ensuring stakeholders' buy-in and employees' empowerment for smoother adoption, and using the right technologies and frameworks."
This content was produced by Insights, the custom content arm of MIT Technology Review. It was not written by MIT Technology Review's editorial staff.