What using artificial intelligence to help monitor surgery can teach us
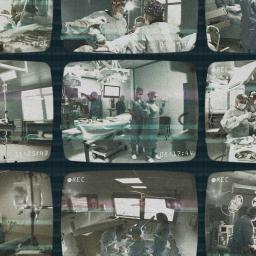
This story originally appeared in The Algorithm, our weekly newsletter on AI. To get stories like this in your inbox first, sign up here.
Every year, some 22,000 Americans a year are killed as a result of serious medical errors in hospitals, many of them on operating tables. There have been cases where surgeons have left surgical sponges inside patients' bodies or performed the wrong procedure altogether.
Teodor Grantcharov, a professor of surgery at Stanford, thinks he has found a tool to make surgery safer and minimize human error: AI-powered black boxes" in operating theaters that work in a similar way to an airplane's black box. These devices, built by Grantcharov's company Surgical Safety Technologies, record everything in the operating room via panoramic cameras, microphones in the ceiling, and anesthesia monitors before using artificial intelligence to help surgeons make sense of the data. They capture the entire operating room as a whole, from the number of times the door is opened to how many non-case-related conversations occur during an operation.
These black boxes are in use in almost 40 institutions in the US, Canada, and Western Europe, from Mount Sinai to Duke to the Mayo Clinic. But are hospitals on the cusp of a new era of safety-or creating an environment of confusion and paranoia?Read the full story by Simar Bajaj here.
This resonated with me as a story with broader implications. Organizations in all sectors are thinking about how to adopt AI to make things safer or more efficient. What this example from hospitals shows is that the situation is not always clear cut, and there are many pitfalls you need to avoid.
Here are three lessons about AI adoption that I learned from this story:
1. Privacy is important, but not always guaranteed.Grantcharov realized very quickly that the only way to get surgeons to use the black box was to make them feel protected from possible repercussions. He has designed the system to record actions but hide the identities of both patients and staff, even deleting all recordings within 30 days. His idea is that no individual should be punished for making a mistake.
The black boxes render each person in the recording anonymous; an algorithm distorts people's voices and blurs out their faces, transforming them into shadowy, noir-like figures. So even if you know what happened, you can't use it against an individual.
But this process is not perfect. Before 30-day-old recordings are automatically deleted, hospital administrators can still see the operating room number, the time of the operation, and the patient's medical record number, so even if personnel are technically de-identified, they aren't truly anonymous. The result is a sense that Big Brother is watching," says Christopher Mantyh, vice chair of clinical operations at Duke University Hospital, which has black boxes in seven operating rooms.
2. You can't adopt new technologies without winning people over first.People are often justifiably suspicious of the new tools, and the system's flaws when it comes to privacy are part of why staff have been hesitant to embrace it. Many doctors and nurses actively boycotted the new surveillance tools. In one hospital, the cameras were sabotaged by being turned around or deliberately unplugged. Some surgeons and staff refused to work in rooms where they were in place.
At the hospital where some of the cameras were initially sabotaged, it took up to six months for surgeons to get used to them. But things went much more smoothly once staff understood the guardrails around the technology. They started trusting it more after one-on-one conversations in which bosses explained how the data was automatically de-identified and deleted.
3. More data doesn't always lead to solutions.You shouldn't adopt new technologies for the sake of adopting new technologies, if they are not actually useful. But to determine whether AI technologies work for you, you need to ask some hard questions. Some hospitals have reported small improvements based on black-box data. Doctors at Duke University Hospital use the data to check how often antibiotics are given on time, and they report turning to this data to help decrease the amount of time operating rooms sit empty between cases.
But getting buy-in from some hospitals has been difficult, because there haven't yet been any large, peer-reviewed studies showing how black boxes actually help to reduce patient complications and save lives. Mount Sinai's chief of general surgery, Celia Divino, says that too much data can be paralyzing. How do you interpret it? What do you do with it?" she asks. This is always a disease."
Read the full story by Simar Bajaj here.
Now read the rest of The AlgorithmDeeper LearningHow a simple circuit could offer an alternative to energy-intensive GPUs
On a table in his lab at the University of Pennsylvania, physicist Sam Dillavou has connected an array of breadboards via a web of brightly colored wires. The setup looks like a DIY home electronics project-and not a particularly elegant one. But this unassuming assembly, which contains 32 variable resistors, can learn to sort data like a machine-learning model. The hope is that the prototype will offer a low-power alternative to the energy-guzzling graphical processing unit chips widely used in machine learning.
Why this matters:AI chips are expensive, and there aren't enough of them to meet the current demand fueled by the AI boom. Training a large language model takes the same amount of energy as the annual consumption of more than a hundred US homes, and generating an image with generative AI uses as much energy ascharging your phone. Dillavou and his colleagues built this circuit as an exploratory effort to find better computing designs.Read more from Sophia Chen here.
Bits and BytesPropagandists are using AI too-and companies need to be open about it
OpenAI has reported on influence operations that use its AI tools. Such reporting, alongside data sharing, should become the industry norm, argue Josh A. Goldstein and Renee DiResta. (MIT Technology Review)
Digital twins are helping scientists run the world's most complex experiments
Engineers use the high-fidelity models to monitor operations, plan fixes, and troubleshoot problems. Digital twins can also use artificial intelligence and machine learning to help make sense of vast amounts of data. (MIT Technology Review)
Silicon Valley is in an uproar over California's proposed AI safety bill
The bill would force companies to create a kill switch" to turn off powerful AI models, guarantee they will not build systems with hazardous capabilities such as creating bioweapons," and report their safety testing. Tech companies argue that this would hinder innovation" and kill open-source development in California. The tech sector loathes regulation, so expect this bill to face a lobbying storm. (FT)
OpenAI offers a peek inside the guts of ChatGPT
The company released a new research paper identifying how the AI model that powers ChatGPT works and how it stores certain concepts. The paper was written by the company's now-defunct superalignment team, which was disbanded after its leaders, including OpenAI cofounder Ilya Sutskever, left the company. OpenAI has facedcriticism from former employeeswho argue that the company is rushing to build AI and ignoring the risks. (Wired)
The AI search engine Perplexity is directly ripping off content from news outlets
The buzzy startup, which has been touted as a challenger to Google Search, has republished parts of exclusive stories from multiple publications, includingForbes and Bloomberg, with inadequate attribution. It's an ominous sign of what could be coming for news media. (Forbes)
It looked like a reliable news site. It was an AI chop shop.
A wild story about how a site called BNN Breaking, which had amassed millions of readers, an international team of journalists, and a publishing deal with Microsoft, was actually just regurgitating AI-generated content riddled with errors. (NYT)