AI-generated images can teach robots how to act
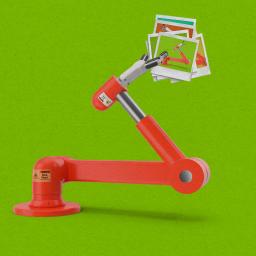
Generative AI models can produce images in response to prompts within seconds, and they've recently been used for everything from highlighting their own inherent bias to preserving precious memories.
Now, researchers from Stephen James's Robot Learning Lab in London are using image-generating AI models for a new purpose: creating training data for robots. They've developed a new system, called Genima, that fine-tunes the image-generating AI model Stable Diffusion to draw robots' movements, helping guide them both in simulations and in the real world. The research is due to be presented at the Conference on Robot Learning (CoRL) next month.
The system could make it easier to train different types of robots to complete tasks-machines ranging from mechanical arms to humanoid robots and driverless cars. It could also help make AI web agents, a next generation of AI tools that can carry out complex tasks with little supervision, better at scrolling and clicking, says Mohit Shridhar, a research scientist specializing in robotic manipulation, who worked on the project.
You can use image-generation systems to do almost all the things that you can do in robotics," he says. We wanted to see if we could take all these amazing things that are happening in diffusion and use them for robotics problems."
To teach a robot to complete a task, researchers normally train a neural network on an image of what's in front of the robot. The network then spits out an output in a different format-the coordinates required to move forward, for example.
Genima's approach is different because both its input and output are images, which is easier for the machines to learn from, says Ivan Kapelyukh, a PhD student at Imperial College London, who specializes in robot learning but wasn't involved in this research.
It's also really great for users, because you can see where your robot will move and what it's going to do. It makes it kind of more interpretable, and means that if you're actually going to deploy this, you could see before your robot went through a wall or something," he says.
Genima works by tapping into Stable Diffusion's ability to recognize patterns (knowing what a mug looks like because it's been trained on images of mugs, for example) and then turning the model into a kind of agent-a decision-making system.
MOHIT SHRIDHAR, YAT LONG (RICHIE) LO, STEPHEN JAMES ROBOT LEARNING LABFirst, the researchers fine-tuned stable Diffusion to let them overlay data from robot sensors onto images captured by its cameras.
The system renders the desired action, like opening a box, hanging up a scarf, or picking up a notebook, into a series of colored spheres on top of the image. These spheres tell the robot where its joint should move one second in the future.
The second part of the process converts these spheres into actions. The team achieved this by using another neural network, called ACT, which is mapped on the same data. Then they used Genima to complete 25 simulations and nine real-world manipulation tasks using a robot arm. The average success rate was 50% and 64%, respectively.
Although these success rates aren't particularly high, Shridhar and the team are optimistic that the robot's speed and accuracy can improve. They're particularly interested in applying Genima to video-generation AI models, which could help a robot predict a sequence of future actions instead of just one.
The research could be particularly useful for training home robots to fold laundry, close drawers, and other domestic tasks. However, its generalized approach means it's not limited to a specific kind of machine, says Zoey Chen, a PhD student at the University of Washington, who has also previously used Stable Diffusion to generate training data for robots but was not involved in this study.
This is a really exciting new direction," she says. I think this can be a general way to train data for all kinds of robots."