AI is changing how we study bird migration
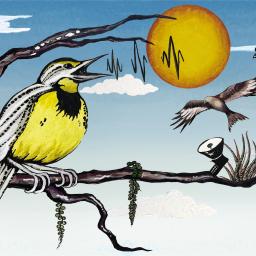
A small songbird soars above Ithaca, New York, on a September night. He is one of 4 billion birds, a great annual river of feathered migration across North America. Midair, he lets out what ornithologists call a nocturnal flight call to communicate with his flock. It's the briefest of signals, barely 50 milliseconds long, emitted in the woods in the middle of the night. But humans have caught it nevertheless, with a microphone topped by a focusing funnel. Moments later, software called BirdVoxDetect, the result of a collaboration between New York University, the Cornell Lab of Ornithology, and Ecole Centrale de Nantes, identifies the bird and classifies it to the species level.
Biologists like Cornell's Andrew Farnsworth had long dreamed of snooping on birds this way. In a warming world increasingly full of human infrastructure that can be deadly to them, like glass skyscrapers and power lines, migratory birds are facing many existential threats. Scientists rely on a combination of methods to track the timing and location of their migrations, but each has shortcomings. Doppler radar, with the weather filtered out, can detect the total biomass of birds in the air, but it can't break that total down by species. GPS tags on individual birds and careful observations by citizen-scientist birders help fill in that gap, but tagging birds at scale is an expensive and invasive proposition. And there's another key problem: Most birds migrate at night, when it's more difficult to identify them visually and while most birders are in bed. For over a century, acoustic monitoring has hovered tantalizingly out of reach as a method that would solve ornithologists' woes.
In the late 1800s, scientists realized that migratory birds made species-specific nocturnal flight calls-acoustic fingerprints." When microphones became commercially available in the 1950s, scientists began recording birds at night. Farnsworth led some of this acoustic ecology research in the 1990s. But even then it was challenging to spot the short calls, some of which are at the edge of the frequency range humans can hear. Scientists ended up with thousands of tapes they had to scour in real time while looking at spectrograms that visualize audio. Though digital technology made recording easier, the perpetual problem," Farnsworth says, was that it became increasingly easy to collect an enormous amount of audio data, but increasingly difficult to analyze even some of it."
Then Farnsworth met Juan Pablo Bello, director of NYU's Music and Audio Research Lab. Fresh off a project using machine learning to identify sources of urban noise pollution in New York City, Bello agreed to take on the problem of nocturnal flight calls. He put together a team including the French machine-listening expert Vincent Lostanlen, and in 2015, the BirdVox project was born to automate the process. Everyone was like, Eventually, when this nut is cracked, this is going to be a super-rich source of information,'" Farnsworth says. But in the beginning, Lostanlen recalls, there was not even a hint that this was doable." It seemed unimaginable that machine learning could approach the listening abilities of experts like Farnsworth.
Andrew is our hero," says Bello. The whole thing that we want to imitate with computers is Andrew."
They started by training BirdVoxDetect, a neural network, to ignore faults like low buzzes caused by rainwater damage to microphones. Then they trained the system to detect flight calls, which differ between (and even within) species and can easily be confused with the chirp of a car alarm or a spring peeper. The challenge, Lostanlen says, was similar to the one a smart speaker faces when listening for its unique wake word," except in this case the distance from the target noise to the microphone is far greater (which means much more background noise to compensate for). And, of course, the scientists couldn't choose a unique sound like Alexa" or Hey Google" for their trigger. For birds, we don't really make that choice. Charles Darwin made that choice for us," he jokes. Luckily, they had a lot of training data to work with-Farnsworth's team had hand-annotated thousands of hours of recordings collected by the microphones in Ithaca.
With BirdVoxDetect trained to detect flight calls, another difficult task lay ahead: teaching it to classify the detected calls by species, which few expert birders can do by ear. To deal with uncertainty, and because there is not training data for every species, they decided on a hierarchical system. For example, for a given call, BirdVoxDetect might be able to identify the bird's order and family, even if it's not sure about the species-just as a birder might at least identify a call as that of a warbler, whether yellow-rumped or chestnut-sided. In training, the neural network was penalized less when it mixed up birds that were closer on the taxonomical tree.
Last August, capping off eight years of research, the team published a paper detailing BirdVoxDetect's machine-learning algorithms. They also released the software as a free, open-source product for ornithologists to use and adapt. In a test on a full season of migration recordings totaling 6,671 hours, the neural network detected 233,124 flight calls. In a 2022 study in the Journal of Applied Ecology, the team that tested BirdVoxDetect found acoustic data as effective as radar for estimating total biomass.
BirdVoxDetect works on a subset of North American migratory songbirds. But through few-shot" learning, it can be trained to detect other, similar birds with just a few training examples. It's like learning a language similar to one you already speak, Bello says. With cheap microphones, the system could be expanded to places around the world without birders or Doppler radar, even in vastly different recording conditions. If you go to a bioacoustics conference and you talk to a number of people, they all have different use cases," says Lostanlen. The next step for bioacoustics, he says, is to create a foundation model, like the ones scientists are working on for natural-language processing and image and video analysis, that would be reconfigurable for any species-even beyond birds. That way, scientists won't have to build a new BirdVoxDetect for every animal they want to study.
The BirdVox project is now complete, but scientists are already building on its algorithms and approach. Benjamin Van Doren, a migration biologist at the University of Illinois Urbana-Champaign who worked on BirdVox, is using Nighthawk, a new user-friendly neural network based on both BirdVoxDetect and the popular birdsong ID app Merlin, to study birds migrating over Chicago and elsewhere in North and South America. And Dan Mennill, who runs a bioacoustics lab at the University of Windsor, says he's excited to try Nighthawk on flight calls his team currently hand-annotates after they're recorded by microphones on the Canadian side of the Great Lakes. One weakness of acoustic monitoring is that unlike radar, a single microphone can't detect the altitude of a bird overhead or the direction in which it is moving. Mennill's lab is experimenting with an array of eight microphones that can triangulate to solve that problem. Sifting through recordings has been slow. But with Nighthawk, the analysis will speed dramatically.
With birds and other migratory animals under threat, Mennill says, BirdVoxDetect came at just the right time. Knowing exactly which birds are flying over in real time can help scientists keep tabs on how species are doing and where they're going. That can inform practical conservation efforts like Lights Out" initiatives that encourage skyscrapers to go dark at night to prevent bird collisions. Bioacoustics is the future of migration research, and we're really just getting to the stage where we have the right tools," he says. This ushers us into a new era."
Christian Elliott is a science and environmental reporter based in Illinois.