Generative adversarial network produces a "universal fingerprint" that will unlock many smartphones
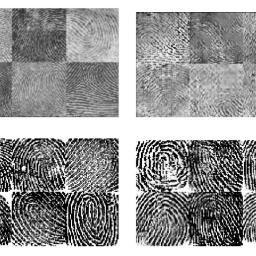
Researchers at NYU and U Michigan have published a paper explaining how they used a pair of machine-learning systems to develop a "universal fingerprint" that can fool the lowest-security fingerprint sensors 76% of the time (it is less effective against higher-security sensors).
The researchers used "generative adversarial networks" (GAN) to develop their attack: this technique uses a pair of machine learning systems, a "generator" which tries to fool a "discriminator," to produce a kind of dialectical back-and-forth in that creates fakes that are harder and harder to detect.
The output was a fake fingerprint designed to attack the capacitive sensors in smartphones and other devices; these sensors work from partial prints that can be rotated out of their original orientation and still unlock the device, and are thus the easiest to fool.
Smartphones generally operate at the second tier of security, in which they are expected to generate false positives 0.1% of the time; and at this level, the researchers were able to spoof the sensors 22% of the time.
Recent research has demonstrated the vulnerability of fingerprint recognition systems to dictionary attacks based on MasterPrints. MasterPrints are real or synthetic fingerprints that can fortuitously match with a large number of fingerprints thereby undermining the security afforded by fingerprint systems. Previous work by Roy et al. generated synthetic MasterPrints at the feature-level. In this work we generate complete image-level MasterPrints known as DeepMasterPrints, whose attack accuracy is found to be much superior than that of previous methods. The proposed method, referred to as Latent Variable Evolution, is based on training a Generative Adversarial Network on a set of real fingerprint images. Stochastic search in the form of the Covariance Matrix Adaptation Evolution Strategy is then used to search for latent input variables to the generator network that can maximize the number of impostor matches as assessed by a fingerprint recognizer. Experiments convey the efficacy of the proposed method in generating DeepMasterPrints. The underlying method is likely to have broad applications in fingerprint security as well as fingerprint synthesis.
DeepMasterPrints: Generating MasterPrints for Dictionary Attacks via Latent Variable Evolution [Philip Bontrager, Aditi Roy, Julian Togelius, Nasir Memon and Arun Ross/Arxiv]
Researchers Created Fake 'Master' Fingerprints to Unlock Smartphones [Daniel Oberhaus/Motherboard]