New AI Estimates the Size of Remote Refugee Camps Using Satellite Data
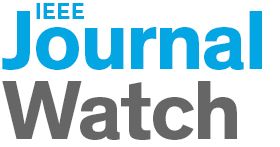
Over the course of the Syrian civil war that has been raging since 2011, the number of displaced refugees has been growing at an alarming rate. The Rukban refugee camp at the nation's southern border near Jordan is one eye-opening example. According to UN data, the number of refugee tents set up in the area has increased from roughly 132 to 11,702 over the four-year period between 2015 and 2019.
Being able to monitor the expansion of refugee camps is important for humanitarian planning and resource allocation. But keeping a reliable count can prove challenging when refugee camps become so large. To support these efforts, several models have been developed to analyze satellite data and estimate the population of refugee camps based on the number of tents that are detected. One recent DARPA-funded research project has led to a new machine learning algorithm with high precision and accuracy in accomplishing the task. (In this case, precision and accuracy are closely related but not synonymous. Accuracy refers to the ratio of all correctly identified pixels-with and without tents; precision is the correct identification of tent-containing pixels only.) The new model is described in a recent publication in IEEE Geoscience and Remote Sensing Letters.
Jiang Li, a professor at Old Dominion University, is a co-developer of the new model. The program was trained and tested using satellite data across two time points, in 2016 and 2017. It takes satellite images and breaks them down into arbitrary pieces, extracting spectral and spatial information. Filters help classify the data at the pixel level. This approach is referred to as a fully convolutional network (FCN) model.
In their study, the researchers compared their FCN model to several others, finding modest increases in accuracy and substantial increases in precision. Their model was up to 4.49 percent more accurate than the others, and as much as 41.99 percent more precise.
In the validation data, manual labelling showed 775 tents and our FCN model discovered 763 tents, with 1.55 percent error," says Li. All other competing models have errors ranging from 12.9 percent to 510.90 percent [meaning they drastically overcounted the number of tents], and are much less accurate."
With the model developed, Li says his team is waiting for guidance from DARPA before implementing the tool in a real-world setting. We are certain that real applications of our new model will require us to connect to some potential users, such as UNOSAT, DHS, and other government agencies. This process may take time," he says. In any event, our model is generic and can be adapted to other applications such as flood and hurricane damage assessment, urban change detection, et cetera."
While it's certainly true that the model could be adapted for other applications, Li cautions that the process could be labor intensive. His team relied on an existing database of labeled image data to build the tent-sensing FCN model, but adapting the model for other purposes could potentially mean manually labelling a new dataset of relevant images.
The data hungry problem is currently a big hurdle for this application. We are investigating state-of-the-art data augmentation strategies and active learning methods as alternatives in order to further improve training efficiency," says Li.
< Back to IEEE Journal Watch