Twitter Bots Are Spreading Massive Amounts of COVID-19 Misinformation
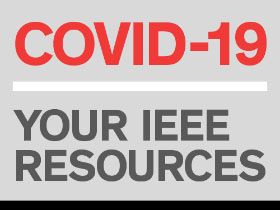
Back in February, the World Health Organization called the flood of misinformation about the coronavirus flowing through the Internet a massive infodemic." Since then, the situation has not improved. While social media platforms have promised to detect and label posts that contain misleading information related to COVID-19, they haven't stopped the surge.
But who is responsible for all those misleading posts? To help answer the question, researchers at Indiana University's Observatory on Social Media used a tool of their own creation called BotometerLite that detects bots on Twitter. They first compiled a list of what they call low-credibility domains" that have been spreading misinformation about COVID-19, then used their tool to determine how many bots were sharing links to this misinformation.
Their findings, which they presented at this year's meeting of the Association for the Advancement of Artificial Intelligence, revealed that bots overwhelmingly spread misinformation about COVID-19 as opposed to accurate content. They also found that some of the bots were acting in a coordinated fashion" to amplify misleading messages.
The scale of the misinformation problem on Twitter is alarming. The researchers found that overall, the number of tweets sharing misleading COVID-19 information was roughly equivalent to the number of tweets that linked to New York Times articles.
We talked with Kai-Cheng Yang, a PhD student who worked on this research, about the bot-detection game.
This conversation has been condensed and edited for clarity.
IEEE Spectrum: How much of the overall misinformation is being spread by bots?
Kai-Cheng Yang: For the links to the low-credibility domains, we find about 20 to 30 percent are shared by bots. The rest are likely shared by humans.
Spectrum: How much of this activity is bots sharing links themselves, and how much is them amplifying tweets that contain misinformation?
Yang: It's a combination. We see some of the bots sharing the links directly and other bots are retweeting tweets containing those links, so they're trying to interact with each other.
Spectrum: How do your Botometer and BotometerLite tools identify bots? What are they looking for?
Yang: Both Botometer and BotometerLite are implemented as supervised machine learning models. We first collect a group of Twitter accounts that are manually annotated as bots or humans. We extract their characteristics from their profiles (number of friends, number of followers, if using background image, etc), and we collect data on content, sentiment, social network, and temporal behaviors. We then train our machine learning models to learn how bots are different from humans in terms of these characteristics. The differences between Botometer and BotometerLite is that Botometer considers all these characteristics whereas BotometerLite only focuses on the profiles for efficiency.
Spectrum: The links these bots are sharing: Where do they lead?
Yang: We have compiled a list of 500 or so low-credibility domains. They're mostly news sites, but we would characterize many of them as fake news.' We also consider extremely hyper-partisan websites as low-credibility.
Spectrum: Can you give a few examples of the kinds of COVID-related misinformation that appear on these sites?
Yang: Common themes include U.S. politics, status of the outbreak, and economic issues. A lot of the articles are not necessarily fake, but they can be hyper-partisan and misleading in some sense. We also see false information like: the virus is weaponized, or political leaders have already been vaccinated.
Spectrum: Did you look at whether the bots spreading misinformation have followers, and whether those followers are humans or other bots?
Yang: Examining the followers of Twitter accounts is much harder due the API rate limit, and we didn't conducted such analysis this time.
Spectrum: In your paper, you write that some of the bots seem to be acting in a coordinated fashion. What does that mean?
Yang: We find that some of the accounts (not necessarily all bots) were sharing information from the same set of low-credibility websites. For two arbitrary accounts, this is very unlikely, yet we found some accounts doing so together. The most plausible explanation is that these accounts were coordinated to push the same information.
Spectrum: How do you detect bot networks?
Yang: I'm assuming you are referring to the network shown in the paper. For that, we simply extract the list of websites each account shares and then find the accounts that have very similar lists and consider them to be connected.
Spectrum: What do you think can be done to reduce the amount of misinformation we're seeing on social media?
Yang: I think it has to be done by the platforms. They can do flagging, or if they know a source is low-credibility, maybe they can do something to reduce the exposure. Another thing we can do is improve the average person's journalism literacy: Try to teach people that there might be those kinds of low-credibility sources or fake news online and to be careful. We have seen some recent studies indicating that if you tell the user what they're seeing might be from low-credibility sources, they become much more sensitive to such things. They're actually less likely to share those articles or links.
Spectrum: Why can't Twitter prevent the creation and proliferation of bots?
Yang: My understanding is that when you try to make your tool or platform easy to use for real users, it opens doors for the bot creators at the same time. So there is a trade-off.
In fact, according to my own experience, recently Twitter started to ask the users to put in their phone numbers and perform more frequent two-step authentications and recaptcha checks. It's quite annoying for me as a normal Twitter user, but I'm sure it makes it harder, though still possible, to create or control bots. I'm happy to see that Twitter has stepped up.
< Back to IEEE COVID-19 Resources