IBM Toolkit Aims at Boosting Efficiencies in AI Chips
In February 2019, IBM Research launched its AI Hardware Center with the stated aim of improving AI computing efficiency by 1,000 times within the decade. Over the last two years, IBM says they've been meeting this ambitious goal: They've improved AI computing efficiency, they claim, by two-and-a-half times per year.
Big Blue's big AI efficiency push comes in the midst of a boom in AI chip startups. Conventional chips often choke on the huge amount of data shuttling back and forth between memory and processing. And many of these AI chip startups say they've built a better mousetrap.
There's an environmental angle to all this, too. Conventional chips waste a lot of energy performing AI algorithms inefficiently. Which can have deleterious effects on the climate.
Recently IBM reported two key developments on their AI efficiency quest. First, IBM will now be collaborating with Red Hat to make IBM's AI digital core compatible with the Red Hat OpenShift ecosystem. This collaboration will allow for IBM's hardware to be developed in parallel with the software, so that as soon as the hardware is ready, all of the software capability will already be in place.
We want to make sure that all the digital work that we're doing, including our work around digital architecture and algorithmic improvement, will lead to the same accuracy," says Mukesh Khare, vice president of IBM Systems Research.
Second, IBM and the design automation firm Synopsys are open-sourcing an analog hardware acceleration kit - highlighting the capabilities analog AI hardware can provide.
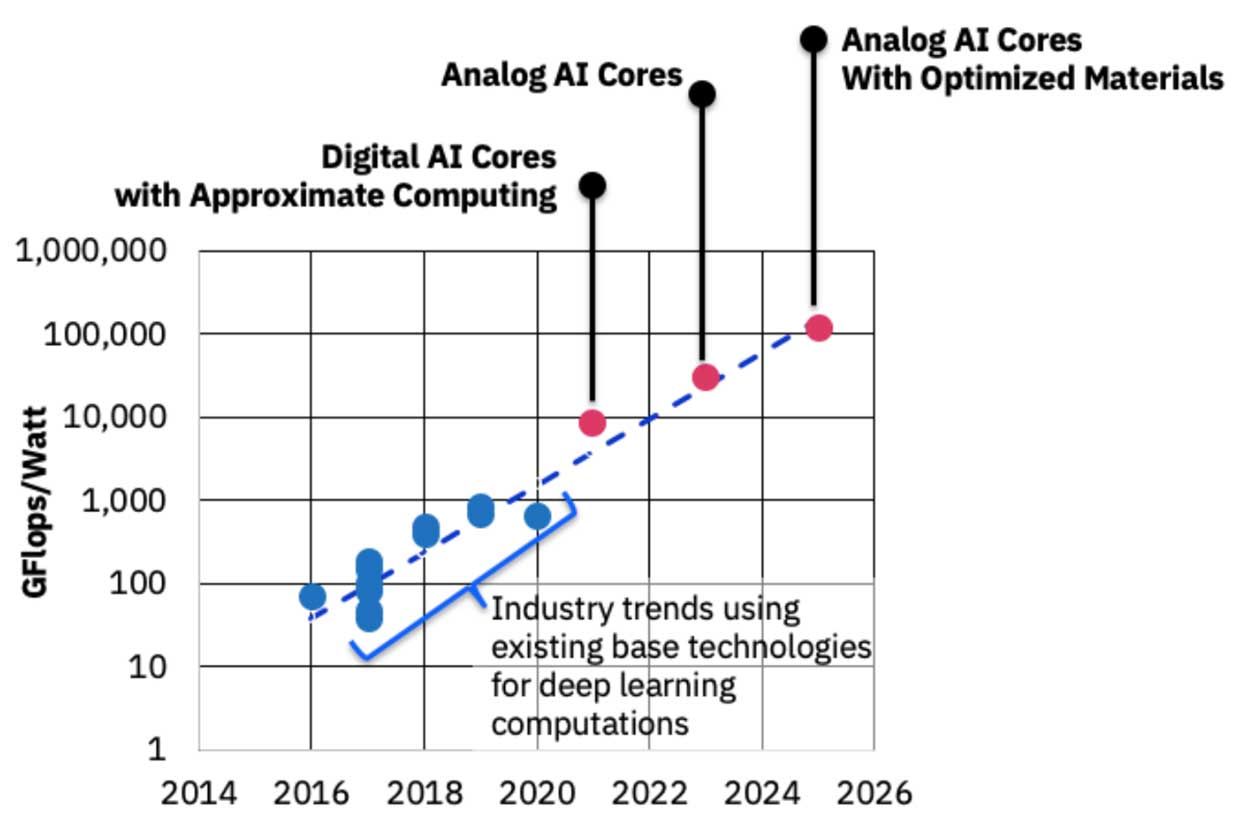
Analog AI is aimed at the so-called von Neumann bottleneck, in which data gets stuck between computation and memory. Analog AI addresses this challenge by performing the computation in the memory itself.
The Analog AI toolkit will be available to startups, academics, students and businesses, according to Khare. They can all ... learn how to leverage some of these new capabilities that are coming down the pipeline. And I'm sure the community may come up with even better ways to exploit this hardware than some of us can come up with," Khare says.
A big part of this toolkit will be the design tools provided by Synopsys.
The data movement is so vast in an AI chip that it really necessitates that memory has to be very close to its computing to do these massive metrics computations," says Arun Venkatachar, vice president of Artificial Intelligence & Central Engineering at Synopsys. As a result, for example, the interconnects become a big challenge."
He says IBM and Synopsys have worked together on both hardware and software for the Analog AI toolkit.
Here, we were involved through the entire stack of chip development: materials research and physics, to the device and all the way through verification and software," Venkatachar says.
Khare says for IBM this holistic approach translated to fundamental device and materials research, chip design, chip architecture, system design software and emulation, as well as a testbed for end-users to to validate performance improvements.
It's important for us to work in tandem and across the entire stack," Khare adds. Because developing hardware without having the right software infrastructure is not complete, and the other way around as well."