Machine learning could vastly speed up the search for new metals
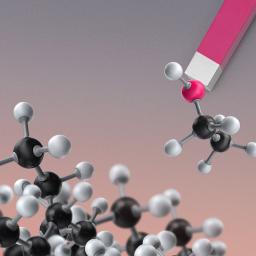
Machine learning could help develop new types of metals with useful properties, such as resistance to extreme temperatures and rust, according to new research. This could be useful in a range of sectors-for example, metals that perform well at lower temperatures could improve spacecraft, while metals that resist corrosion could be used for boats and submarines.
Currently, scientists typically run experiments in the lab to look for ways to combine metals to create new ones. Usually they start off with one well-known element, like iron, which is cheap and malleable, and add one or two others to see the effect on the original material. It's a laborious process of trial and error that inevitably yields more duds than useful results.
But the new paper, published in Science earlier this month, suggests that with AI, researchers can far more precisely predict which combinations of metals will show promise.
Researchers from the Max Planck Institute managed to identify 17 promising new metals using this method. The team was hunting for metals with a low level of invar," which refers to how much materials expand or contract when exposed to high or low temperatures.
Metals with low invar don't change size under extreme temperatures. They are commonly used in industries where that property is useful-for example, the transportation and storage of natural gas, explains Ziyuan Rao, a materials science researcher at the Max Planck Institute and a coauthor of the paper.
The team managed to find these new metals through a combination of AI and lab experiments. First, they had to overcome a significant challenge: a lack of existing data they could use to train the machine-learning models. They trained the models on the data they had-several hundred data points describing the properties of existing metal alloys. The AI system used that data to make predictions for new metals that would exhibit low invar.
The researchers then created those metals in a lab, measured the results, and fed those results back into the machine-learning model. The process continued that way-the model suggesting metal combinations, the researchers testing them and feeding the data back in-until the 17 promising new metals emerged.
The findings could help pave the way for greater use of machine learning in materials science, a field that still relies heavily on laboratory experimentation. Also, the technique of using machine learning to make predictions that are then checked in the lab could be adapted for discovery in other fields, such as chemistry and physics, say experts in materials science.
To understand why it's a significant development, it's worth looking at the traditional way new compounds are usually created, says Michael Titus, an assistant professor of materials engineering at Purdue University, who was not involved in the research. The process of tinkering in the lab is painstaking and inefficient.
It's truly like finding a needle in a haystack to find materials that exhibit a special property," Titus says. He often tells his new graduate students that there are easily a million possible new materials waiting to be discovered. Machine learning could help researchers decide which paths to pursue.
Easo George, a professor of materials science and engineering at the University of Tennessee, who wasn't involved in this research, was surprised by what the team was able to accomplish using the new technique.
It's very impressive," he says.
In the future, the team wants to use machine learning to help discover new alloys with more than just one desirable property. George agrees that computational methods will be crucial to the future of materials science.
The machine-learning approach is probably going to be dominant, because people have tried to scan very large spaces experimentally, but that is very time consuming and expensive," he says. The test is, are you finding something useful?"