2023 Innovator of the Year: As AI models are released into the wild, Sharon Li wants to ensure they’re safe
Sharon Li is MIT Technology Review's 2023 Innovator of the Year. Meet the rest of this year's Innovators Under 35.
As we launch AI systems from the lab into the real world, we need to be prepared for these systems to break in surprising and catastrophic ways. It's already happening. Last year, for example, a chess-playing robot arm in Moscow fractured the finger of a seven-year-old boy. The robot grabbed the boy's finger as he was moving a chess piece and let go only after nearby adults managed to pry open its claws.
This did not happen because the robot was programmed to do harm. It was because the robot was overly confident that the boy's finger was a chess piece.
The incident is a classic example of something Sharon Li, 32, wants to prevent. Li, an assistant professor at the University of Wisconsin, Madison, is a pioneer in an AI safety feature called out-of-distribution (OOD) detection. This feature, she says, helps AI models determine when they should abstain from action if faced with something they weren't trained on.
Li developed one of the first algorithms on out-of-distribution detection for deep neural networks. Google has since set up a dedicated team to integrate OOD detection into its products. Last year, Li's theoretical analysis of OOD detection was chosen from over 10,000 submissions as an outstanding paper by NeurIPS, one of the most prestigious AI conferences.
We're currently in an AI gold rush, and tech companies are racing to release their AI models. But most of today's models are trained to identify specific things and often fail when they encounter the unfamiliar scenarios typical of the messy, unpredictable real world. Their inability to reliably understand what they know" and what they don't know" is the weakness behind many AI disasters.
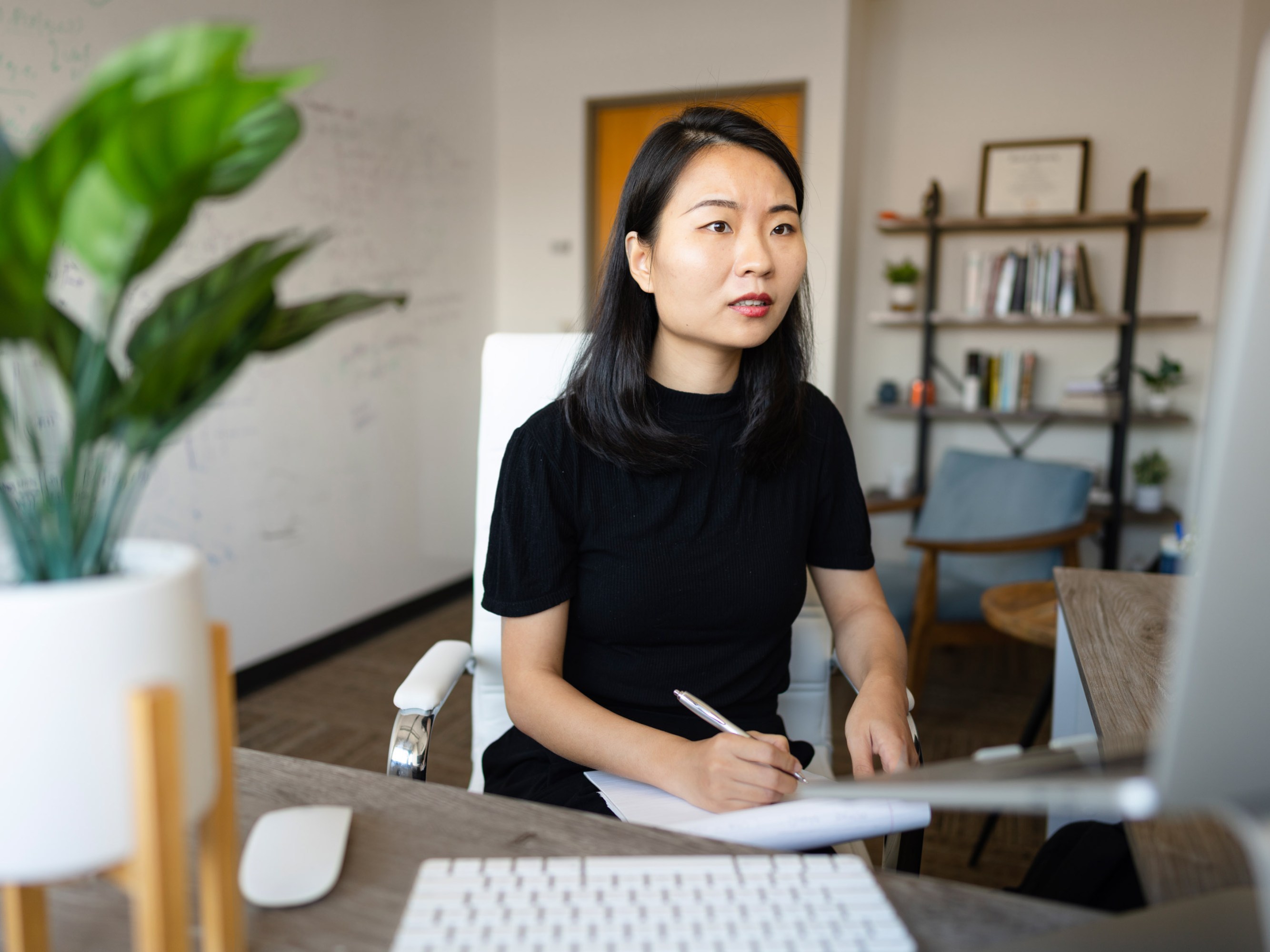
Li's work calls on the AI community to rethink its approach to training. A lot of the classic approaches that have been in place over the last 50 years are actually safety unaware," she says.
Her approach embraces uncertainty by using machine learning to detect unknown data out in the world and design AI models to adjust to it on the fly. Out-of-distribution detection could help prevent accidents when autonomous cars run into unfamiliar objects on the road, or make medical AI systems more useful in finding a new disease.
In all those situations, what we really need [is a] safety-aware machine learning model that's able to identify what it doesn't know," says Li.
This approach could also aid today's buzziest AI technology, large language models such as ChatGPT. These models are often confident liars, presenting falsehoods as facts. This is where OOD detection could help. Say a person asks a chatbot a question it doesn't have an answer to in its training data. Instead of making something up, an AI model using OOD detection would decline to answer.
Li's research tackles one of the most fundamental questions in machine learning, says John Hopcroft, a professor at Cornell University, who was her PhD advisor.
Her work has also seen a surge of interest from other researchers. What she is doing is getting other researchers to work," says Hopcroft, who adds that she's basically created one of the subfields" of AI safety research.
Now, Li is seeking a deeper understanding of the safety risks relating to large AI models, which are powering all kinds of new online applications and products. She hopes that by making the models underlying these products safer, we'll be better able to mitigate AI's risks.
The ultimate goal is to ensure trustworthy, safe machine learning," she says.
Sharon Li is one of MIT Technology Review's 2023 Innovators Under 35. Meet the rest of this year's honorees.