The race to find new materials with AI needs more data. Meta is giving massive amounts away for free.
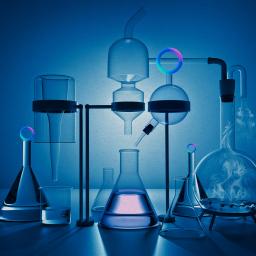
Meta is releasing a massive data set and models, called Open Materials 2024, that could help scientists use AI to discover new materials much faster. OMat24 tackles one of the biggest bottlenecks in the discovery process: data.
To find new materials, scientists calculate the properties of elements across the periodic table and simulate different combinations on computers. This work could help us discover new materials with properties that can help mitigate climate change, for example, by making better batteries or helping create new sustainable fuels. But it requires massive data sets that are hard to come by. Creating them requires a lot of computing power and is very expensive. Many of the top data sets and models available now are also proprietary, and researchers don't have access to them. That's where Meta is hoping to help: The company is releasing its new data set and models today for free and is making them open source. The data set and models are available on Hugging Face for anyone to download, tinker with, and use.
We're really firm believers that by contributing to the community and building upon open-source data models, the whole community moves further, faster," says Larry Zitnick, the lead researcher for the OMat project.
Zitnick says the newOMat24 model will top the Matbench Discovery leaderboard, which ranks the best machine-learning models for materials science. Its data set will also be one of the biggest available.
Materials science is having a machine-learning revolution," says Shyue Ping Ong, a professor of nanoengineering at the University of California, San Diego, who was not involved in the project.
Previously, scientists were limited to doing very accurate calculations of material properties on very small systems or doing less accurate calculations on very big systems, says Ong. The processes were laborious and expensive. Machine learning has bridged that gap, and AI models allow scientists to perform simulations on combinations of any elements in the periodic table much more quickly and cheaply, he says.
Meta's decision to make its data set openly available is more significant than the AI model itself, says Gabor Csanyi, a professor of molecular modeling at the University of Cambridge, who was not involved in the work.
This is in stark contrast to other large industry players such as Google and Microsoft, which also recently published competitive-looking models which were trained on equally large but secret data sets," Csanyi says.
To create the OMat24 data set, Meta took an existing one called Alexandria and sampled materials from it. Then they ran various simulations and calculations of different atoms to scale it.
Meta's data set has around 110 million data points, which is many times larger than earlier ones. Others also don't necessarily have high-quality data, says Ong.
Meta has significantly expanded the data set beyond what the current materials science community has done, and with high accuracy, says Ong.
Creating the data sets requires vast computational capacity, and Meta is one of the few companies in the world that can afford that. Zitnick says the company has another motive for this work: It's hoping to find new materials to make its smart augmented-reality glasses more affordable.
Previous work on open databases, such as one created by the Materials Project, has transformed computational materials science over the last decade, says Chris Bartel, an assistant professor of chemical engineering and materials science at the University of Minnesota, who was also not involved in Meta's work.
Tools such as Google's GNoME (graphical networks for material exploration) have shown that the potential to find new materials increases with the size of the training set, he adds.
The public release of the [OMat24] data set is truly a gift for the community and is certain to immediately accelerate research in this space," Bartel says.