Deep Learning breakthrough made by Rice University scientists
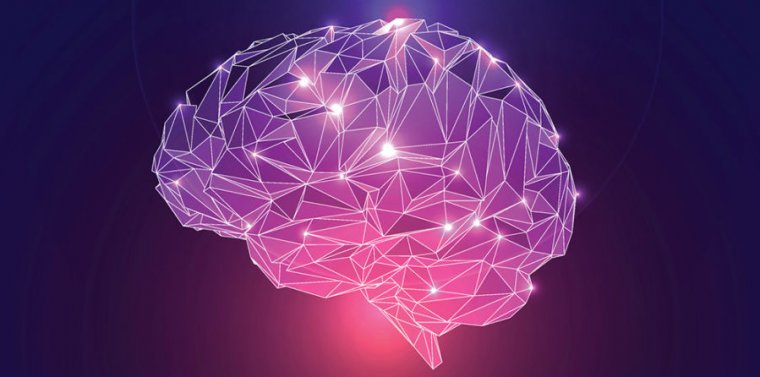
Enlarge (credit: pitju / Adobe Stock)
In an earlier deep learning article, we talked about how inference workloads-the use of already-trained neural networks to analyze data-can run on fairly cheap hardware, but running the training workload that the neural network "learns" on is orders of magnitude more expensive.
In particular, the more potential inputs you have to an algorithm, the more out of control your scaling problem gets when analyzing its problem space. This is where MACH, a research project authored by Rice University's Tharun Medini and Anshumali Shrivastava, comes in. MACH is an acronym for Merged Average Classifiers via Hashing, and according to lead researcher Shrivastava, "[its] training times are about 7-10 times faster, and... memory footprints are 2-4 times smaller" than those of previous large-scale deep learning techniques.
In describing the scale of extreme classification problems, Medini refers to online shopping search queries, noting that "there are easily more than 100 million products online." This is, if anything, conservative-one data company claimed Amazon US alone sold 606 million separate products, with the entire company offering more than three billion products worldwide. Another company reckons the US product count at 353 million. Medini continues, "a neural network that takes search input and predicts from 100 million outputs, or products, will typically end up with about 2,000 parameters per product. So you multiply those, and the final layer of the neural network is 200 billion parameters ... [and] I'm talking about a very, very dead simple neural network model."
Read 6 remaining paragraphs | Comments