Deepstack AI has beaten professional poker players in heads-up no-limit Texas hold’em
by noreply@blogger.com (brian wang) from NextBigFuture.com on (#28WSC)
Artificial intelligence has seen a number of breakthroughs in recent years, with games often serving as significant milestones. A common feature of games with these successes is that they involve information symmetry among the players, where all players have identical information. This property of perfect information, though, is far more common in games than in real-world problems.
Poker is the quintessential game of imperfect information, and it has been a longstanding challenge problem in artificial intelligence. In this paper researchers introduce DeepStack, a new algorithm for imperfect information settings such as poker. It combines recursive reasoning to handle information asymmetry, decomposition to focus computation on the relevant decision, and a form of intuition about arbitrary poker situations that is automatically learned from selfplay games using deep learning. In a study involving dozens of participants and 44,000 hands of poker, DeepStack becomes the first computer program to beat professional poker players in heads-up no-limit Texas hold'em. Furthermore, they show this approach dramatically reduces worst-case exploitability compared to the abstraction paradigm that has been favored for over a decade
DeepStack was evaluated against 33 professional poker players from the International Federation of Poker. Each participant was asked to play a 3,000-game match over a month.
DeepStack takes a fundamentally different approach. It continues to use the recursive reasoning of CFR to handle information asymmetry. However, it does not compute and store a complete strategy prior to play and so has no need for explicit abstraction. Instead it considers each particular situation as it arises during play, but not in isolation. It avoids reasoning about the entire remainder of the game by substituting the computation beyond a certain depth with a fast approximate estimate. This estimate can be thought of as DeepStack's intuition: a gut feeling of the value of holding any possible private cards in any possible poker situation. Finally, DeepStack's intuition, much like human intuition, needs to be trained. They train it with deep learning using examples generated from random poker situations. We show that DeepStack is theoretically sound, produces substantially less exploitable strategies than abstraction-based techniques, and is the first program to beat professional poker players at HUNL with a remarkable average win rate of over 450 mbb/g.
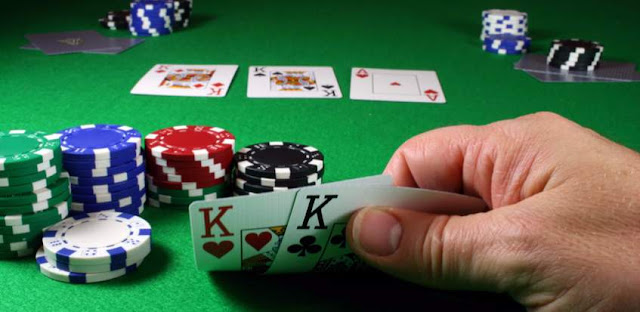
A rival AI poker team of researcher from Carnegie Mellon University announced a $200,000 match between its system, Libratus, and four poker pros: Jason Les, Dong Kim, Daniel McAulay, and Jimmy Chou. Collectively, the four human pros will play 120,000 hands of heads-up no-limit Texas hold 'em over 20 days against Libratus.
At the end of day two, however, Libratus was up by $150,126. It was winning against three players and losing against one.
Read more


Poker is the quintessential game of imperfect information, and it has been a longstanding challenge problem in artificial intelligence. In this paper researchers introduce DeepStack, a new algorithm for imperfect information settings such as poker. It combines recursive reasoning to handle information asymmetry, decomposition to focus computation on the relevant decision, and a form of intuition about arbitrary poker situations that is automatically learned from selfplay games using deep learning. In a study involving dozens of participants and 44,000 hands of poker, DeepStack becomes the first computer program to beat professional poker players in heads-up no-limit Texas hold'em. Furthermore, they show this approach dramatically reduces worst-case exploitability compared to the abstraction paradigm that has been favored for over a decade
DeepStack was evaluated against 33 professional poker players from the International Federation of Poker. Each participant was asked to play a 3,000-game match over a month.
DeepStack takes a fundamentally different approach. It continues to use the recursive reasoning of CFR to handle information asymmetry. However, it does not compute and store a complete strategy prior to play and so has no need for explicit abstraction. Instead it considers each particular situation as it arises during play, but not in isolation. It avoids reasoning about the entire remainder of the game by substituting the computation beyond a certain depth with a fast approximate estimate. This estimate can be thought of as DeepStack's intuition: a gut feeling of the value of holding any possible private cards in any possible poker situation. Finally, DeepStack's intuition, much like human intuition, needs to be trained. They train it with deep learning using examples generated from random poker situations. We show that DeepStack is theoretically sound, produces substantially less exploitable strategies than abstraction-based techniques, and is the first program to beat professional poker players at HUNL with a remarkable average win rate of over 450 mbb/g.
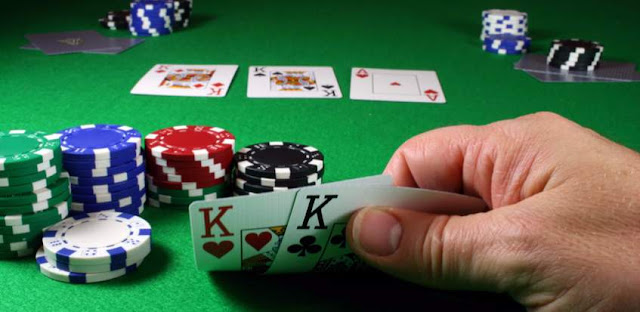
A rival AI poker team of researcher from Carnegie Mellon University announced a $200,000 match between its system, Libratus, and four poker pros: Jason Les, Dong Kim, Daniel McAulay, and Jimmy Chou. Collectively, the four human pros will play 120,000 hands of heads-up no-limit Texas hold 'em over 20 days against Libratus.
At the end of day two, however, Libratus was up by $150,126. It was winning against three players and losing against one.
Read more