Battery charging meets machine learning
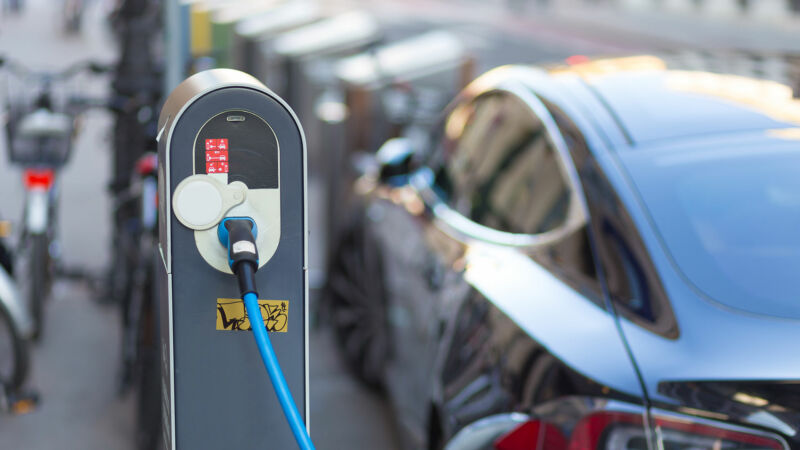
Enlarge / The seemingly simple act of charging is getting increasingly complex. (credit: Argonne National Lab)
Batteries tend to involve lots of trade-offs. You can have high capacity, but it means more weight and a slower charge. Or you can charge quickly and see the lifetime of your battery drop with each cycle. There are ways to optimize performance-figuring out the fastest charging you can do without cutting into the battery life-but that varies from product to product and requires extensive testing to identify.
But perhaps that testing is not so extensive, thanks to a new system described in the journal Nature. The system uses a combination of machine learning and Bayesian inference to rapidly zero in on the optimal charging pattern for any battery, cutting the amount of testing needed down considerably.
Not so fastFast charging is obviously useful for everything from phones to cars. But when a battery is subjected to fast charging, it doesn't store its ions quite as efficiently. The overall capacity will go down, and there's the potential for permanent damage, as some of the lithium ends up precipitating out and becoming unavailable for future use.
Read 12 remaining paragraphs | Comments